
Data Engineering Podcast
This show goes behind the scenes for the tools, techniques, and difficulties associated with the discipline of data engineering. Databases, workflows, automation, and data manipulation are just some of the topics that you will find here.
Latest episodes

103 snips
Sep 17, 2023 • 1h 2min
Building Linked Data Products With JSON-LD
In this podcast, Brian Platz discusses the concept and implications of linked data, the benefits of using JSON-LD for building semantic data products, the challenges faced in building linked data products, and the need for improved data management tools.

25 snips
Sep 10, 2023 • 1h 1min
An Overview Of The State Of Data Orchestration In An Increasingly Complex Data Ecosystem
Nick Schrock, creator of Dagster, discusses the state of data orchestration technology and its application. They explore the challenges and benefits of orchestrators, the balance between information and infrastructure, and the capabilities and challenges of data orchestration. They also discuss low code and no code solutions in data work, their integration into software engineering, and the role of data orchestration in ML workflows.

15 snips
Sep 4, 2023 • 42min
Eliminate The Overhead In Your Data Integration With The Open Source dlt Library
The podcast explores the dlt project, an open source Python library for data loading. It discusses the challenges in data integration, the benefits of dlt over other tools, and how to start building pipelines. Other topics include the journey of becoming a data engineer, performance considerations of using Python, collaboration in data integration, and integration with different runtimes. The hosts emphasize the need for better education in data management and practical solutions.

Aug 28, 2023 • 1h 1min
Building An Internal Database As A Service Platform At Cloudflare
This podcast explores how Cloudflare provides PostgreSQL as a service to their developers for low latency and high uptime services at global scale. They discuss challenges in maintaining high uptime and managing data volume, scaling considerations and load balancing strategies, the evolvement of database engines, differences in version upgrades between Postgres and MySQL, innovative usage and challenges in building a database platform at Cloudflare, and lessons learned in building their system.

Aug 20, 2023 • 55min
Harnessing Generative AI For Creating Educational Content With Illumidesk
Generative AI in educational content creation, building a data-driven experience for learners, challenges of dealing with large amounts of data, analyzing learner interactions and improving content development, data normalization and personalized learning paths, implementation and architecture of Illumidesk platform, evolution of platform and incorporating LLM framework into data engineering pipeline, application and usage of Illumidesk for content creation.

26 snips
Aug 14, 2023 • 47min
Unpacking The Seven Principles Of Modern Data Pipelines
Summary
Data pipelines are the core of every data product, ML model, and business intelligence dashboard. If you're not careful you will end up spending all of your time on maintenance and fire-fighting. The folks at Rivery distilled the seven principles of modern data pipelines that will help you stay out of trouble and be productive with your data. In this episode Ariel Pohoryles explains what they are and how they work together to increase your chances of success.
Announcements
Hello and welcome to the Data Engineering Podcast, the show about modern data management
Introducing RudderStack Profiles. RudderStack Profiles takes the SaaS guesswork and SQL grunt work out of building complete customer profiles so you can quickly ship actionable, enriched data to every downstream team. You specify the customer traits, then Profiles runs the joins and computations for you to create complete customer profiles. Get all of the details and try the new product today at dataengineeringpodcast.com/rudderstack
This episode is brought to you by Datafold – a testing automation platform for data engineers that finds data quality issues before the code and data are deployed to production. Datafold leverages data-diffing to compare production and development environments and column-level lineage to show you the exact impact of every code change on data, metrics, and BI tools, keeping your team productive and stakeholders happy. Datafold integrates with dbt, the modern data stack, and seamlessly plugs in your data CI for team-wide and automated testing. If you are migrating to a modern data stack, Datafold can also help you automate data and code validation to speed up the migration. Learn more about Datafold by visiting dataengineeringpodcast.com/datafold
Your host is Tobias Macey and today I'm interviewing Ariel Pohoryles about the seven principles of modern data pipelines
Interview
Introduction
How did you get involved in the area of data management?
Can you start by defining what you mean by a "modern" data pipeline?
At Rivery you published a white paper identifying seven principles of modern data pipelines:
Zero infrastructure management
ELT-first mindset
Speaks SQL and Python
Dynamic multi-storage layers
Reverse ETL & operational analytics
Full transparency
Faster time to value
What are the applications of data that you focused on while identifying these principles?
How do the application of these principles influence the ability of organizations and their data teams to encourage and keep pace with the use of data in the business?
What are the technical components of a pipeline infrastructure that are necessary to support a "modern" workflow?
How do the technologies involved impact the organizational involvement with how data is applied throughout the business?
When using managed services, what are the ways that the pricing model acts to encourage/discourage experimentation/exploration with data?
What are the most interesting, innovative, or unexpected ways that you have seen these seven principles implemented/applied?
What are the most interesting, unexpected, or challenging lessons that you have learned while working with customers to adapt to these principles?
What are the cases where some/all of these principles are undesirable/impractical to implement?
What are the opportunities for further advancement/sophistication in the ways that teams work with and gain value from data?
Contact Info
LinkedIn
Parting Question
From your perspective, what is the biggest gap in the tooling or technology for data management today?
Closing Announcements
Thank you for listening! Don't forget to check out our other shows. Podcast.__init__ covers the Python language, its community, and the innovative ways it is being used. The Machine Learning Podcast helps you go from idea to production with machine learning.
Visit the site to subscribe to the show, sign up for the mailing list, and read the show notes.
If you've learned something or tried out a project from the show then tell us about it! Email hosts@dataengineeringpodcast.com) with your story.
To help other people find the show please leave a review on Apple Podcasts and tell your friends and co-workers
Links
Rivery
7 Principles Of The Modern Data Pipeline
ELT
Reverse ETL
Martech Landscape
Data Lakehouse
Databricks
Snowflake
The intro and outro music is from The Hug by The Freak Fandango Orchestra / CC BY-SASponsored By:Datafold: 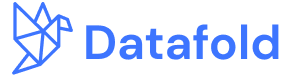
This episode is brought to you by Datafold – a testing automation platform for data engineers that finds data quality issues before the code and data are deployed to production. Datafold leverages data-diffing to compare production and development environments and column-level lineage to show you the exact impact of every code change on data, metrics, and BI tools, keeping your team productive and stakeholders happy. Datafold integrates with dbt, the modern data stack, and seamlessly plugs in your data CI for team-wide and automated testing. If you are migrating to a modern data stack, Datafold can also help you automate data and code validation to speed up the migration. Learn more about Datafold by visiting [dataengineeringpodcast.com/datafold](https://www.dataengineeringpodcast.com/datafold) today!Rudderstack: 
Introducing RudderStack Profiles. RudderStack Profiles takes the SaaS guesswork and SQL grunt work out of building complete customer profiles so you can quickly ship actionable, enriched data to every downstream team. You specify the customer traits, then Profiles runs the joins and computations for you to create complete customer profiles. Get all of the details and try the new product today at [dataengineeringpodcast.com/rudderstack](https://www.dataengineeringpodcast.com/rudderstack)Support Data Engineering Podcast

10 snips
Aug 6, 2023 • 1h 2min
Quantifying The Return On Investment For Your Data Team
Exploring how to calculate the ROI for data teams, the podcast covers methods of measuring ROI, collecting and analyzing data for efficiency, optimizing queries, generative AI, innovative approaches to ROI, and the biggest gaps in data management tooling.

14 snips
Jul 31, 2023 • 1h 10min
Strategies For A Successful Data Platform Migration
Summary
All software systems are in a constant state of evolution. This makes it impossible to select a truly future-proof technology stack for your data platform, making an eventual migration inevitable. In this episode Gleb Mezhanskiy and Rob Goretsky share their experiences leading various data platform migrations, and the hard-won lessons that they learned so that you don't have to.
Announcements
Hello and welcome to the Data Engineering Podcast, the show about modern data management
Introducing RudderStack Profiles. RudderStack Profiles takes the SaaS guesswork and SQL grunt work out of building complete customer profiles so you can quickly ship actionable, enriched data to every downstream team. You specify the customer traits, then Profiles runs the joins and computations for you to create complete customer profiles. Get all of the details and try the new product today at dataengineeringpodcast.com/rudderstack
Modern data teams are using Hex to 10x their data impact. Hex combines a notebook style UI with an interactive report builder. This allows data teams to both dive deep to find insights and then share their work in an easy-to-read format to the whole org. In Hex you can use SQL, Python, R, and no-code visualization together to explore, transform, and model data. Hex also has AI built directly into the workflow to help you generate, edit, explain and document your code. The best data teams in the world such as the ones at Notion, AngelList, and Anthropic use Hex for ad hoc investigations, creating machine learning models, and building operational dashboards for the rest of their company. Hex makes it easy for data analysts and data scientists to collaborate together and produce work that has an impact. Make your data team unstoppable with Hex. Sign up today at dataengineeringpodcast.com/hex to get a 30-day free trial for your team!
Your host is Tobias Macey and today I'm interviewing Gleb Mezhanskiy and Rob Goretsky about when and how to think about migrating your data stack
Interview
Introduction
How did you get involved in the area of data management?
A migration can be anything from a minor task to a major undertaking. Can you start by describing what constitutes a migration for the purposes of this conversation?
Is it possible to completely avoid having to invest in a migration?
What are the signals that point to the need for a migration?
What are some of the sources of cost that need to be accounted for when considering a migration? (both in terms of doing one, and the costs of not doing one)
What are some signals that a migration is not the right solution for a perceived problem?
Once the decision has been made that a migration is necessary, what are the questions that the team should be asking to determine the technologies to move to and the sequencing of execution?
What are the preceding tasks that should be completed before starting the migration to ensure there is no breakage downstream of the changing component(s)?
What are some of the ways that a migration effort might fail?
What are the major pitfalls that teams need to be aware of as they work through a data platform migration?
What are the opportunities for automation during the migration process?
What are the most interesting, innovative, or unexpected ways that you have seen teams approach a platform migration?
What are the most interesting, unexpected, or challenging lessons that you have learned while working on data platform migrations?
What are some ways that the technologies and patterns that we use can be evolved to reduce the cost/impact/need for migraitons?
Contact Info
Gleb
LinkedIn
@glebmm on Twitter
Rob
LinkedIn
RobGoretsky on GitHub
Parting Question
From your perspective, what is the biggest gap in the tooling or technology for data management today?
Closing Announcements
Thank you for listening! Don't forget to check out our other shows. Podcast.__init__ covers the Python language, its community, and the innovative ways it is being used. The Machine Learning Podcast helps you go from idea to production with machine learning.
Visit the site to subscribe to the show, sign up for the mailing list, and read the show notes.
If you've learned something or tried out a project from the show then tell us about it! Email hosts@dataengineeringpodcast.com) with your story.
To help other people find the show please leave a review on Apple Podcasts and tell your friends and co-workers
Links
Datafold
Podcast Episode
Informatica
Airflow
Snowflake
Podcast Episode
Redshift
Eventbrite
Teradata
BigQuery
Trino
EMR == Elastic Map-Reduce
Shadow IT
Podcast Episode
Mode Analytics
Looker
Sunk Cost Fallacy
data-diff
Podcast Episode
SQLGlot
[Dagster](dhttps://dagster.io/)
dbt
The intro and outro music is from The Hug by The Freak Fandango Orchestra / CC BY-SASponsored By:Hex: 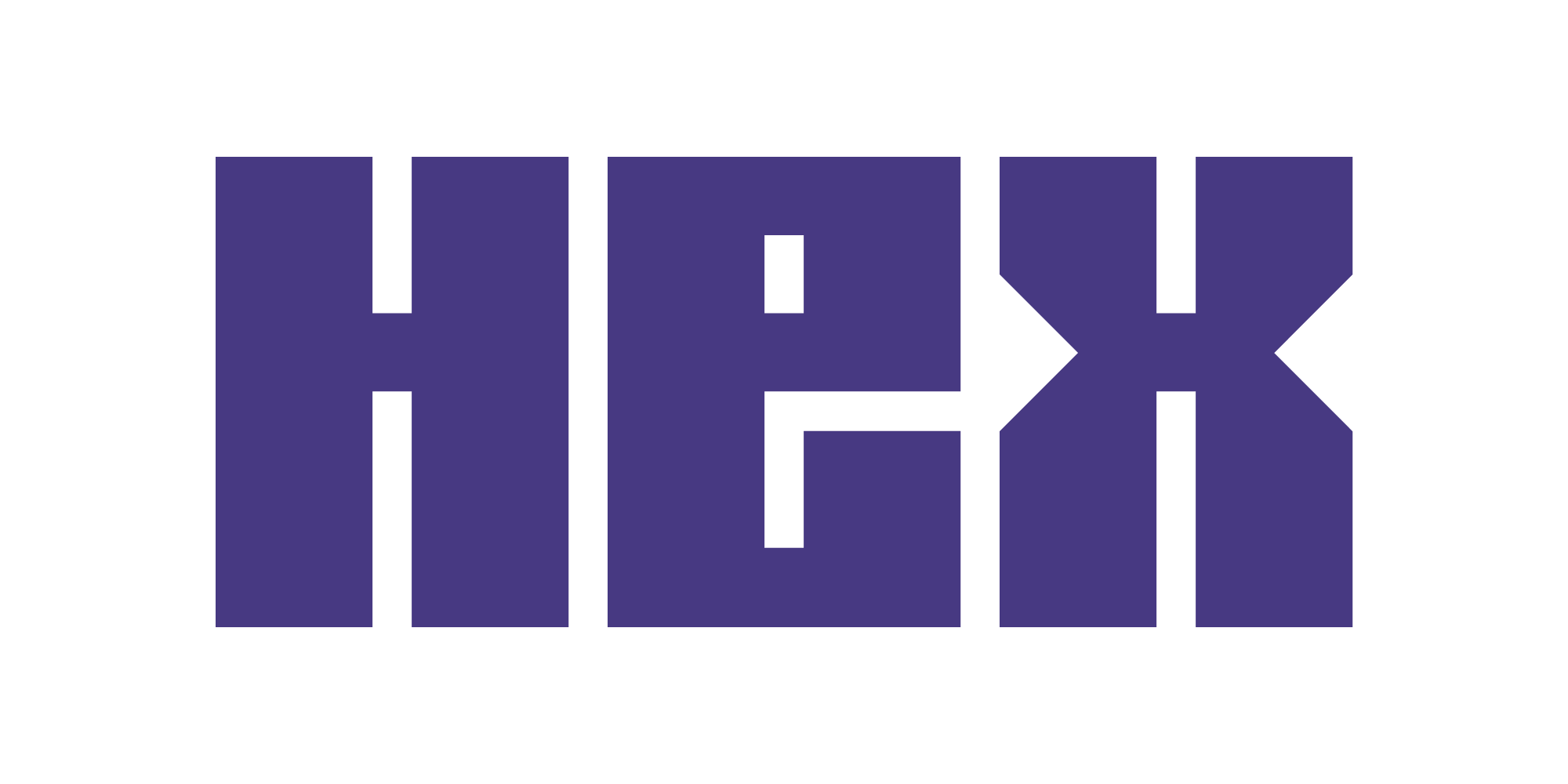
Hex is a collaborative workspace for data science and analytics. A single place for teams to explore, transform, and visualize data into beautiful interactive reports. Use SQL, Python, R, no-code and AI to find and share insights across your organization. Empower everyone in an organization to make an impact with data. Sign up today at [dataengineeringpodcast.com/hex](https://www.dataengineeringpodcast.com/hex} and get 30 days free!Rudderstack: 
Introducing RudderStack Profiles. RudderStack Profiles takes the SaaS guesswork and SQL grunt work out of building complete customer profiles so you can quickly ship actionable, enriched data to every downstream team. You specify the customer traits, then Profiles runs the joins and computations for you to create complete customer profiles. Get all of the details and try the new product today at [dataengineeringpodcast.com/rudderstack](https://www.dataengineeringpodcast.com/rudderstack)Support Data Engineering Podcast

Jul 24, 2023 • 41min
Build Real Time Applications With Operational Simplicity Using Dozer
Summary
Real-time data processing has steadily been gaining adoption due to advances in the accessibility of the technologies involved. Despite that, it is still a complex set of capabilities. To bring streaming data in reach of application engineers Matteo Pelati helped to create Dozer. In this episode he explains how investing in high performance and operationally simplified streaming with a familiar API can yield significant benefits for software and data teams together.
Announcements
Hello and welcome to the Data Engineering Podcast, the show about modern data management
Introducing RudderStack Profiles. RudderStack Profiles takes the SaaS guesswork and SQL grunt work out of building complete customer profiles so you can quickly ship actionable, enriched data to every downstream team. You specify the customer traits, then Profiles runs the joins and computations for you to create complete customer profiles. Get all of the details and try the new product today at dataengineeringpodcast.com/rudderstack
Modern data teams are using Hex to 10x their data impact. Hex combines a notebook style UI with an interactive report builder. This allows data teams to both dive deep to find insights and then share their work in an easy-to-read format to the whole org. In Hex you can use SQL, Python, R, and no-code visualization together to explore, transform, and model data. Hex also has AI built directly into the workflow to help you generate, edit, explain and document your code. The best data teams in the world such as the ones at Notion, AngelList, and Anthropic use Hex for ad hoc investigations, creating machine learning models, and building operational dashboards for the rest of their company. Hex makes it easy for data analysts and data scientists to collaborate together and produce work that has an impact. Make your data team unstoppable with Hex. Sign up today at dataengineeringpodcast.com/hex to get a 30-day free trial for your team!
Your host is Tobias Macey and today I'm interviewing Matteo Pelati about Dozer, an open source engine that includes data ingestion, transformation, and API generation for real-time sources
Interview
Introduction
How did you get involved in the area of data management?
Can you describe what Dozer is and the story behind it?
What was your decision process for building Dozer as open source?
As you note in the documentation, Dozer has overlap with a number of technologies that are aimed at different use cases. What was missing from each of them and the center of their Venn diagram that prompted you to build Dozer?
In addition to working in an interesting technological cross-section, you are also targeting a disparate group of personas. Who are you building Dozer for and what were the motivations for that vision?
What are the different use cases that you are focused on supporting?
What are the features of Dozer that enable engineers to address those uses, and what makes it preferable to existing alternative approaches?
Can you describe how Dozer is implemented?
How have the design and goals of the platform changed since you first started working on it?
What are the architectural "-ilities" that you are trying to optimize for?
What is involved in getting Dozer deployed and integrated into an existing application/data infrastructure?
How can teams who are using Dozer extend/integrate with Dozer?
What does the development/deployment workflow look like for teams who are building on top of Dozer?
What is your governance model for Dozer and balancing the open source project against your business goals?
What are the most interesting, innovative, or unexpected ways that you have seen Dozer used?
What are the most interesting, unexpected, or challenging lessons that you have learned while working on Dozer?
When is Dozer the wrong choice?
What do you have planned for the future of Dozer?
Contact Info
LinkedIn
@pelatimtt on Twitter
Parting Question
From your perspective, what is the biggest gap in the tooling or technology for data management today?
Closing Announcements
Thank you for listening! Don't forget to check out our other shows. Podcast.__init__ covers the Python language, its community, and the innovative ways it is being used. The Machine Learning Podcast helps you go from idea to production with machine learning.
Visit the site to subscribe to the show, sign up for the mailing list, and read the show notes.
If you've learned something or tried out a project from the show then tell us about it! Email hosts@dataengineeringpodcast.com) with your story.
To help other people find the show please leave a review on Apple Podcasts and tell your friends and co-workers
Links
Dozer
Data Robot
Netflix Bulldozer
CubeJS
Podcast Episode
JVM == Java Virtual Machine
Flink
Podcast Episode
Airbyte
Podcast Episode
Fivetran
Podcast Episode
Delta Lake
Podcast Episode
LMDB
Vector Database
LLM == Large Language Model
Rockset
Podcast Episode
Tinybird
Podcast Episode
Rust Language
Materialize
Podcast Episode
RisingWave
DuckDB
Podcast Episode
DataFusion
Polars
The intro and outro music is from The Hug by The Freak Fandango Orchestra / CC BY-SASponsored By:Hex: 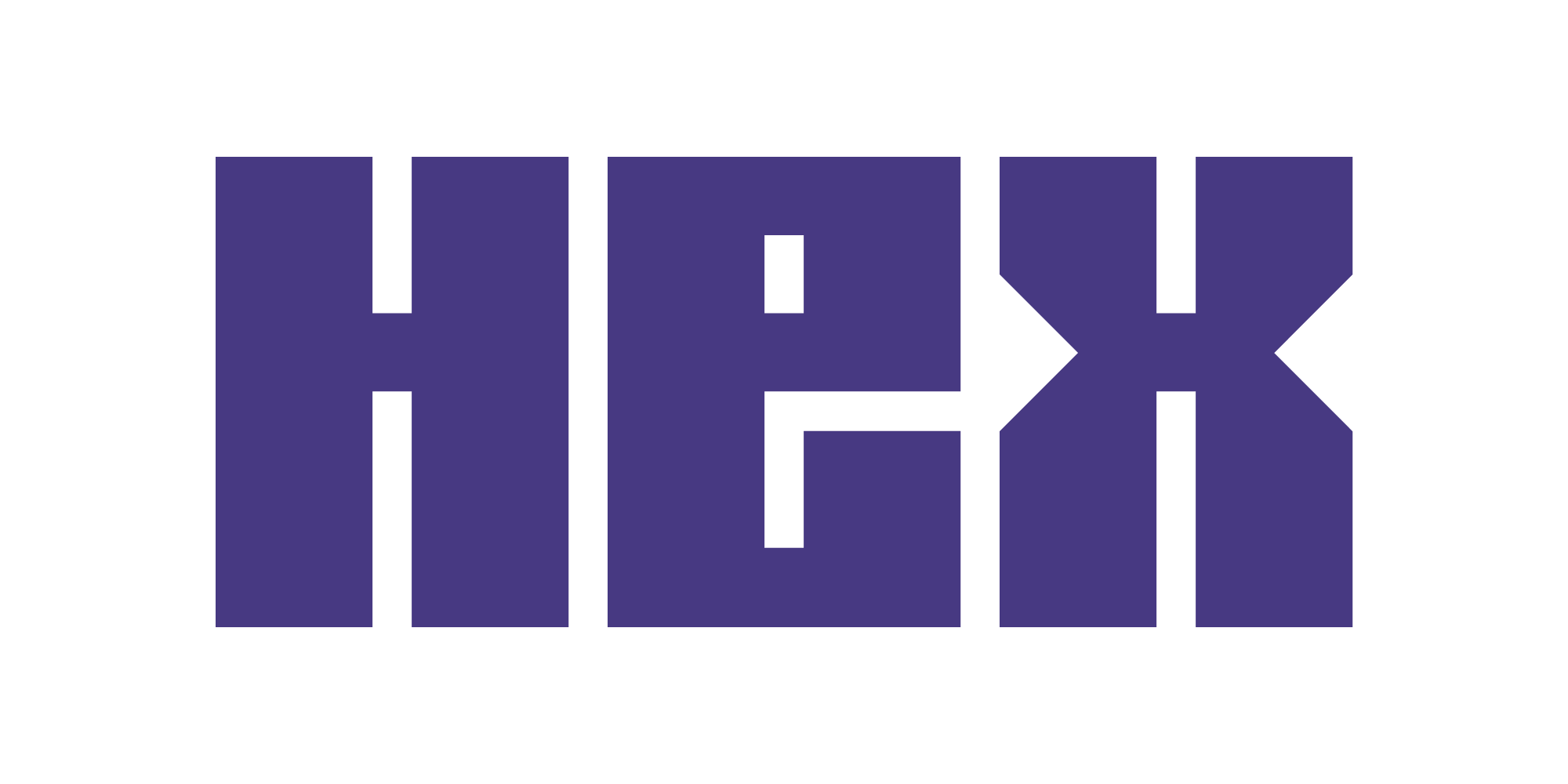
Hex is a collaborative workspace for data science and analytics. A single place for teams to explore, transform, and visualize data into beautiful interactive reports. Use SQL, Python, R, no-code and AI to find and share insights across your organization. Empower everyone in an organization to make an impact with data. Sign up today at [dataengineeringpodcast.com/hex](https://www.dataengineeringpodcast.com/hex} and get 30 days free!Rudderstack: 
Introducing RudderStack Profiles. RudderStack Profiles takes the SaaS guesswork and SQL grunt work out of building complete customer profiles so you can quickly ship actionable, enriched data to every downstream team. You specify the customer traits, then Profiles runs the joins and computations for you to create complete customer profiles. Get all of the details and try the new product today at [dataengineeringpodcast.com/rudderstack](https://www.dataengineeringpodcast.com/rudderstack)Support Data Engineering Podcast

12 snips
Jul 17, 2023 • 55min
Datapreneurs - How Todays Business Leaders Are Using Data To Define The Future
Summary
Data has been one of the most substantial drivers of business and economic value for the past few decades. Bob Muglia has had a front-row seat to many of the major shifts driven by technology over his career. In his recent book "Datapreneurs" he reflects on the people and businesses that he has known and worked with and how they relied on data to deliver valuable services and drive meaningful change.
Announcements
Hello and welcome to the Data Engineering Podcast, the show about modern data management
Introducing RudderStack Profiles. RudderStack Profiles takes the SaaS guesswork and SQL grunt work out of building complete customer profiles so you can quickly ship actionable, enriched data to every downstream team. You specify the customer traits, then Profiles runs the joins and computations for you to create complete customer profiles. Get all of the details and try the new product today at dataengineeringpodcast.com/rudderstack
Your host is Tobias Macey and today I'm interviewing Bob Muglia about his recent book about the idea of "Datapreneurs" and the role of data in the modern economy
Interview
Introduction
How did you get involved in the area of data management?
Can you describe what your concept of a "Datapreneur" is?
How is this distinct from the common idea of an entreprenur?
What do you see as the key inflection points in data technologies and their impacts on business capabilities over the past ~30 years?
In your role as the CEO of Snowflake you had a first-row seat for the rise of the "modern data stack". What do you see as the main positive and negative impacts of that paradigm?
What are the key issues that are yet to be solved in that ecosmnjjystem?
For technologists who are thinking about launching new ventures, what are the key pieces of advice that you would like to share?
What do you see as the short/medium/long-term impact of AI on the technical, business, and societal arenas?
What are the most interesting, innovative, or unexpected ways that you have seen business leaders use data to drive their vision?
What are the most interesting, unexpected, or challenging lessons that you have learned while working on the Datapreneurs book?
What are your key predictions for the future impact of data on the technical/economic/business landscapes?
Contact Info
LinkedIn
Parting Question
From your perspective, what is the biggest gap in the tooling or technology for data management today?
Closing Announcements
Thank you for listening! Don't forget to check out our other shows. Podcast.__init__ covers the Python language, its community, and the innovative ways it is being used. The Machine Learning Podcast helps you go from idea to production with machine learning.
Visit the site to subscribe to the show, sign up for the mailing list, and read the show notes.
If you've learned something or tried out a project from the show then tell us about it! Email hosts@dataengineeringpodcast.com) with your story.
To help other people find the show please leave a review on Apple Podcasts and tell your friends and co-workers
Links
Datapreneurs Book
SQL Server
Snowflake
Z80 Processor
Navigational Database
System R
Redshift
Microsoft Fabric
Databricks
Looker
Fivetran
Podcast Episode
Databricks Unity Catalog
RelationalAI
6th Normal Form
Pinecone Vector DB
Podcast Episode
Perplexity AI
The intro and outro music is from The Hug by The Freak Fandango Orchestra / CC BY-SASponsored By:Rudderstack: 
Introducing RudderStack Profiles. RudderStack Profiles takes the SaaS guesswork and SQL grunt work out of building complete customer profiles so you can quickly ship actionable, enriched data to every downstream team. You specify the customer traits, then Profiles runs the joins and computations for you to create complete customer profiles. Get all of the details and try the new product today at [dataengineeringpodcast.com/rudderstack](https://www.dataengineeringpodcast.com/rudderstack)Support Data Engineering Podcast
Remember Everything You Learn from Podcasts
Save insights instantly, chat with episodes, and build lasting knowledge - all powered by AI.