

Shining Some Light In The Black Box Of PostgreSQL Performance
Nov 6, 2023
Lukas Fittl, a database performance expert, discusses performance bottlenecks in PostgreSQL, tools like 'explain' in PostgreSQL, common optimization challenges, and the importance of tuning configuration parameters. He also shares insights on the development of PG analyze, enabling performance settings in PostgreSQL, and the evolution of database engines.
54:52
1 chevron_right 2 chevron_right 3 chevron_right 4 chevron_right 5 chevron_right 6 chevron_right 7 chevron_right
Introduction
00:00 • 3min
Understanding and Optimizing Database Performance
02:30 • 13min
Understanding and Addressing Performance Problems in PostgreSQL
15:53 • 5min
Tuning Configuration Parameters in PostgreSQL
21:05 • 7min
Developing PG analyze to Enhance PostgreSQL Query Performance
28:03 • 3min
Enabling Performance Settings in PostgreSQL for Better Diagnostics
30:48 • 4min
Lessons from the Evolution of Database Engines
34:48 • 20min
Summary
Databases are the core of most applications, but they are often treated as inscrutable black boxes. When an application is slow, there is a good probability that the database needs some attention. In this episode Lukas Fittl shares some hard-won wisdom about the causes and solution of many performance bottlenecks and the work that he is doing to shine some light on PostgreSQL to make it easier to understand how to keep it running smoothly.
Announcements
- Hello and welcome to the Data Engineering Podcast, the show about modern data management
- Introducing RudderStack Profiles. RudderStack Profiles takes the SaaS guesswork and SQL grunt work out of building complete customer profiles so you can quickly ship actionable, enriched data to every downstream team. You specify the customer traits, then Profiles runs the joins and computations for you to create complete customer profiles. Get all of the details and try the new product today at dataengineeringpodcast.com/rudderstack
- You shouldn't have to throw away the database to build with fast-changing data. You should be able to keep the familiarity of SQL and the proven architecture of cloud warehouses, but swap the decades-old batch computation model for an efficient incremental engine to get complex queries that are always up-to-date. With Materialize, you can! It’s the only true SQL streaming database built from the ground up to meet the needs of modern data products. Whether it’s real-time dashboarding and analytics, personalization and segmentation or automation and alerting, Materialize gives you the ability to work with fresh, correct, and scalable results — all in a familiar SQL interface. Go to dataengineeringpodcast.com/materialize today to get 2 weeks free!
- Data lakes are notoriously complex. For data engineers who battle to build and scale high quality data workflows on the data lake, Starburst powers petabyte-scale SQL analytics fast, at a fraction of the cost of traditional methods, so that you can meet all your data needs ranging from AI to data applications to complete analytics. Trusted by teams of all sizes, including Comcast and Doordash, Starburst is a data lake analytics platform that delivers the adaptability and flexibility a lakehouse ecosystem promises. And Starburst does all of this on an open architecture with first-class support for Apache Iceberg, Delta Lake and Hudi, so you always maintain ownership of your data. Want to see Starburst in action? Go to dataengineeringpodcast.com/starburst and get $500 in credits to try Starburst Galaxy today, the easiest and fastest way to get started using Trino.
- This episode is brought to you by Datafold – a testing automation platform for data engineers that finds data quality issues before the code and data are deployed to production. Datafold leverages data-diffing to compare production and development environments and column-level lineage to show you the exact impact of every code change on data, metrics, and BI tools, keeping your team productive and stakeholders happy. Datafold integrates with dbt, the modern data stack, and seamlessly plugs in your data CI for team-wide and automated testing. If you are migrating to a modern data stack, Datafold can also help you automate data and code validation to speed up the migration. Learn more about Datafold by visiting dataengineeringpodcast.com/datafold
- Your host is Tobias Macey and today I'm interviewing Lukas Fittl about optimizing your database performance and tips for tuning Postgres
Interview
- Introduction
- How did you get involved in the area of data management?
- What are the different ways that database performance problems impact the business?
- What are the most common contributors to performance issues?
- What are the useful signals that indicate performance challenges in the database?
- For a given symptom, what are the steps that you recommend for determining the proximate cause?
- What are the potential negative impacts to be aware of when tuning the configuration of your database?
- How does the database engine influence the methods used to identify and resolve performance challenges?
- Most of the database engines that are in common use today have been around for decades. How have the lessons learned from running these systems over the years influenced the ways to think about designing new engines or evolving the ones we have today?
- What are the most interesting, innovative, or unexpected ways that you have seen to address database performance?
- What are the most interesting, unexpected, or challenging lessons that you have learned while working on databases?
- What are your goals for the future of database engines?
Contact Info
- @LukasFittl on Twitter
Parting Question
- From your perspective, what is the biggest gap in the tooling or technology for data management today?
Closing Announcements
- Thank you for listening! Don't forget to check out our other shows. Podcast.__init__ covers the Python language, its community, and the innovative ways it is being used. The Machine Learning Podcast helps you go from idea to production with machine learning.
- Visit the site to subscribe to the show, sign up for the mailing list, and read the show notes.
- If you've learned something or tried out a project from the show then tell us about it! Email hosts@dataengineeringpodcast.com) with your story.
- To help other people find the show please leave a review on Apple Podcasts and tell your friends and co-workers
Links
- PGAnalyze
- Citus Data
- ORM == Object Relational Mapper
- N+1 Query
- Autovacuum
- Write-ahead Log
- pg_stat_io
- random_page_cost
- pgvector
- Vector Database
- Ottertune
- Citus Extension
- Hydra
- Clickhouse
- MyISAM
- MyRocks
- InnoDB
- Great Expectations
- OpenTelemetry
The intro and outro music is from The Hug by The Freak Fandango Orchestra / CC BY-SA
Sponsored By:
- Starburst: 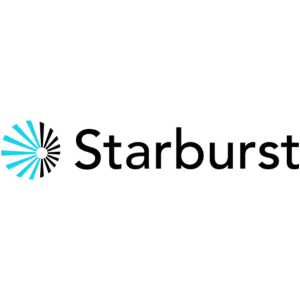 This episode is brought to you by Starburst - a data lake analytics platform for data engineers who are battling to build and scale high quality data pipelines on the data lake. Powered by Trino, Starburst runs petabyte-scale SQL analytics fast at a fraction of the cost of traditional methods, helping you meet all your data needs ranging from AI/ML workloads to data applications to complete analytics. Trusted by the teams at Comcast and Doordash, Starburst delivers the adaptability and flexibility a lakehouse ecosystem promises, while providing a single point of access for your data and all your data governance allowing you to discover, transform, govern, and secure all in one place. Starburst does all of this on an open architecture with first-class support for Apache Iceberg, Delta Lake and Hudi, so you always maintain ownership of your data. Want to see Starburst in action? Try Starburst Galaxy today, the easiest and fastest way to get started using Trino, and get $500 of credits free. [dataengineeringpodcast.com/starburst](https://www.dataengineeringpodcast.com/starburst)
- Rudderstack:  Introducing RudderStack Profiles. RudderStack Profiles takes the SaaS guesswork and SQL grunt work out of building complete customer profiles so you can quickly ship actionable, enriched data to every downstream team. You specify the customer traits, then Profiles runs the joins and computations for you to create complete customer profiles. Get all of the details and try the new product today at [dataengineeringpodcast.com/rudderstack](https://www.dataengineeringpodcast.com/rudderstack)
- Materialize: 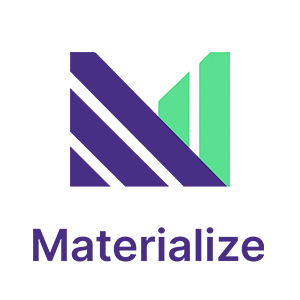 You shouldn't have to throw away the database to build with fast-changing data. Keep the familiar SQL, keep the proven architecture of cloud warehouses, but swap the decades-old batch computation model for an efficient incremental engine to get complex queries that are always up-to-date. That is Materialize, the only true SQL streaming database built from the ground up to meet the needs of modern data products: Fresh, Correct, Scalable — all in a familiar SQL UI. Built on Timely Dataflow and Differential Dataflow, open source frameworks created by cofounder Frank McSherry at Microsoft Research, Materialize is trusted by data and engineering teams at Ramp, Pluralsight, Onward and more to build real-time data products without the cost, complexity, and development time of stream processing. Go to [materialize.com](https://materialize.com/register/?utm_source=depodcast&utm_medium=paid&utm_campaign=early-access) today and get 2 weeks free!
- Datafold: 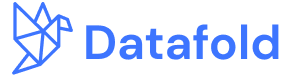 This episode is brought to you by Datafold – a testing automation platform for data engineers that finds data quality issues before the code and data are deployed to production. Datafold leverages data-diffing to compare production and development environments and column-level lineage to show you the exact impact of every code change on data, metrics, and BI tools, keeping your team productive and stakeholders happy. Datafold integrates with dbt, the modern data stack, and seamlessly plugs in your data CI for team-wide and automated testing. If you are migrating to a modern data stack, Datafold can also help you automate data and code validation to speed up the migration. Learn more about Datafold by visiting [dataengineeringpodcast.com/datafold](https://www.dataengineeringpodcast.com/datafold) today!