
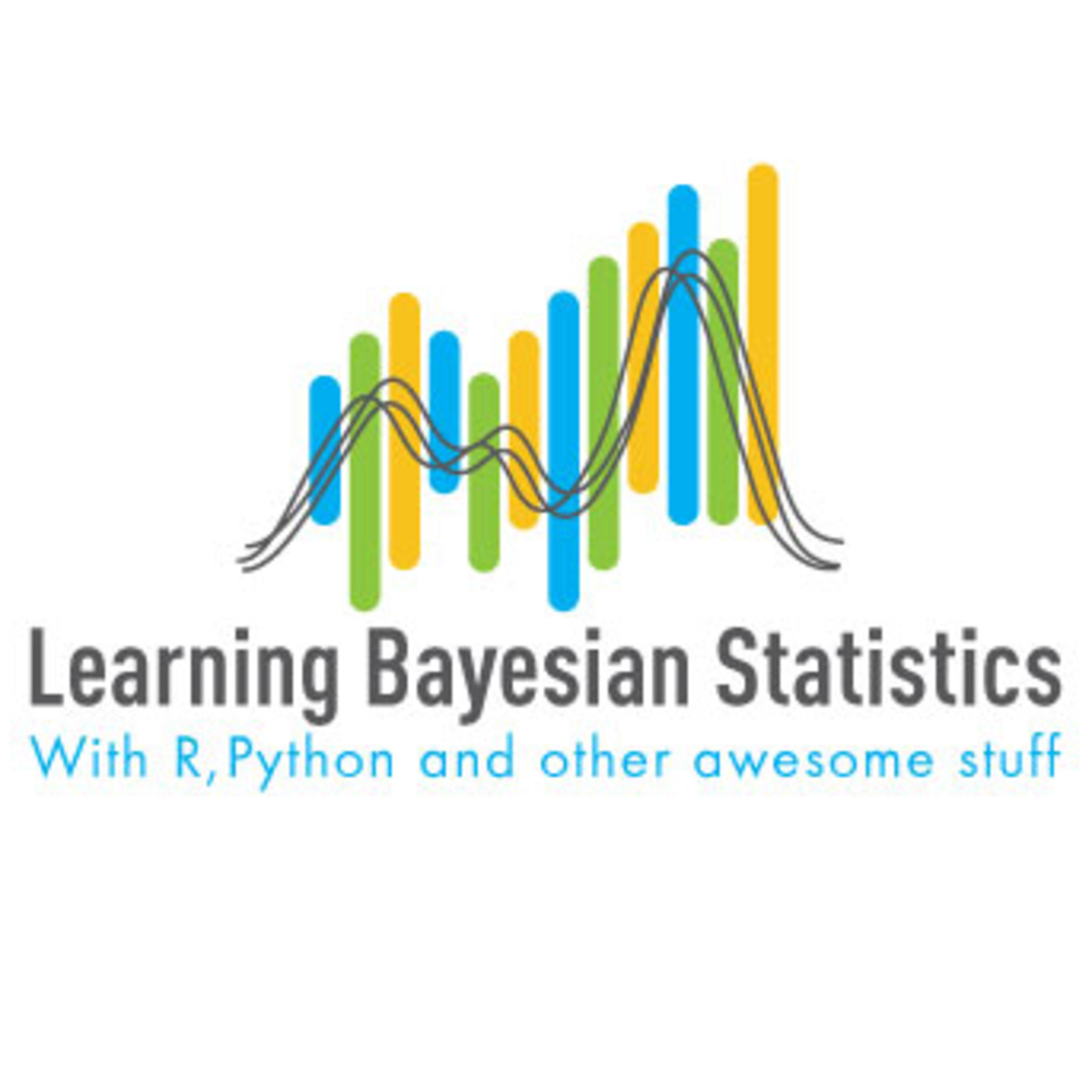
Learning Bayesian Statistics
Alexandre Andorra
Are you a researcher or data scientist / analyst / ninja? Do you want to learn Bayesian inference, stay up to date or simply want to understand what Bayesian inference is?
Then this podcast is for you! You'll hear from researchers and practitioners of all fields about how they use Bayesian statistics, and how in turn YOU can apply these methods in your modeling workflow.
When I started learning Bayesian methods, I really wished there were a podcast out there that could introduce me to the methods, the projects and the people who make all that possible.
So I created "Learning Bayesian Statistics", where you'll get to hear how Bayesian statistics are used to detect black matter in outer space, forecast elections or understand how diseases spread and can ultimately be stopped.
But this show is not only about successes -- it's also about failures, because that's how we learn best. So you'll often hear the guests talking about what *didn't* work in their projects, why, and how they overcame these challenges. Because, in the end, we're all lifelong learners!
My name is Alex Andorra by the way, and I live in Estonia. By day, I'm a data scientist and modeler at the https://www.pymc-labs.io/ (PyMC Labs) consultancy. By night, I don't (yet) fight crime, but I'm an open-source enthusiast and core contributor to the python packages https://docs.pymc.io/ (PyMC) and https://arviz-devs.github.io/arviz/ (ArviZ). I also love https://www.pollsposition.com/ (election forecasting) and, most importantly, Nutella. But I don't like talking about it – I prefer eating it.
So, whether you want to learn Bayesian statistics or hear about the latest libraries, books and applications, this podcast is for you -- just subscribe! You can also support the show and https://www.patreon.com/learnbayesstats (unlock exclusive Bayesian swag on Patreon)!
Then this podcast is for you! You'll hear from researchers and practitioners of all fields about how they use Bayesian statistics, and how in turn YOU can apply these methods in your modeling workflow.
When I started learning Bayesian methods, I really wished there were a podcast out there that could introduce me to the methods, the projects and the people who make all that possible.
So I created "Learning Bayesian Statistics", where you'll get to hear how Bayesian statistics are used to detect black matter in outer space, forecast elections or understand how diseases spread and can ultimately be stopped.
But this show is not only about successes -- it's also about failures, because that's how we learn best. So you'll often hear the guests talking about what *didn't* work in their projects, why, and how they overcame these challenges. Because, in the end, we're all lifelong learners!
My name is Alex Andorra by the way, and I live in Estonia. By day, I'm a data scientist and modeler at the https://www.pymc-labs.io/ (PyMC Labs) consultancy. By night, I don't (yet) fight crime, but I'm an open-source enthusiast and core contributor to the python packages https://docs.pymc.io/ (PyMC) and https://arviz-devs.github.io/arviz/ (ArviZ). I also love https://www.pollsposition.com/ (election forecasting) and, most importantly, Nutella. But I don't like talking about it – I prefer eating it.
So, whether you want to learn Bayesian statistics or hear about the latest libraries, books and applications, this podcast is for you -- just subscribe! You can also support the show and https://www.patreon.com/learnbayesstats (unlock exclusive Bayesian swag on Patreon)!
Episodes
Mentioned books

Apr 14, 2022 • 59min
#59 Bayesian Modeling in Civil Engineering, with Michael Faber
In large-scale one-off civil infrastructure, decision-making under uncertainty is part of the job, that’s just how it is. But, civil engineers don't get the luxury of building 10^6 versions of the bridge, offshore wind turbine or aeronautical structure to consider a relative frequency interpretation!And as you’ll hear, challenges don’t stop there: you also have to consider natural hazards such as earthquakes, rockfall and typhoons — in case you were wondering, civil engineering is not among the boring jobs!To talk about these original topics, I had the pleasure to host Michael Faber. Michael is a Professor at the Department of Built Environment at Aalborg University, Denmark, the President of the Joint Committee on Structural Safety and is a tremendously deep thinker on the Bayesian interpretation of probability as it pertains to the risk-informed management of big infrastructure.His research interests are directed on governance and management of risks, resilience and sustainability in the built environment — doing all that with Bayesian probabilistic modeling and applied Bayesian decision analysis, as you’ll hear.Our theme music is « Good Bayesian », by Baba Brinkman (feat MC Lars and Mega Ran). Check out his awesome work at https://bababrinkman.com/ !Thank you to my Patrons for making this episode possible!Yusuke Saito, Avi Bryant, Ero Carrera, Giuliano Cruz, Tim Gasser, James Wade, Tradd Salvo, Adam Bartonicek, William Benton, Alan O'Donnell, Mark Ormsby, James Ahloy, Robin Taylor, Thomas Wiecki, Chad Scherrer, Nathaniel Neitzke, Zwelithini Tunyiswa, Elea McDonnell Feit, Bertrand Wilden, James Thompson, Stephen Oates, Gian Luca Di Tanna, Jack Wells, Matthew Maldonado, Ian Costley, Ally Salim, Larry Gill, Joshua Duncan, Ian Moran, Paul Oreto, Colin Caprani, George Ho, Colin Carroll, Nathaniel Burbank, Michael Osthege, Rémi Louf, Clive Edelsten, Henri Wallen, Hugo Botha, Vinh Nguyen, Raul Maldonado, Marcin Elantkowski, Adam C. Smith, Will Kurt, Andrew Moskowitz, Hector Munoz, Marco Gorelli, Simon Kessell, Bradley Rode, Patrick Kelley, Rick Anderson, Casper de Bruin, Philippe Labonde, Matthew McAnear, Michael Hankin, Cameron Smith, Luis Iberico, Tomáš Frýda, Ryan Wesslen, Andreas Netti, Riley King, Aaron Jones, Yoshiyuki Hamajima, Sven De Maeyer, Michael DeCrescenzo, Fergal M, Mason Yahr, Naoya Kanai, Steven Rowland and Aubrey Clayton.Visit https://www.patreon.com/learnbayesstats to unlock exclusive Bayesian swag ;)Links from the show:Michael's profile on Aalborg University: https://vbn.aau.dk/en/persons/100493Michael's LinkedIn profile: https://www.linkedin.com/in/michael-havbro-faber-22898414/Statistics and Probability Theory - In Pursuit of Engineering Decision Support: https://link.springer.com/book/10.1007/978-94-007-4056-3Bayes in Civil Engineering - an abridged personal account of research and applications: https://www.linkedin.com/pulse/bayes-civil-engineering-michael-havbro-faberWebsite of the Joint Committee on Structural Safety (JCSS): https://www.jcss-lc.org/

Mar 21, 2022 • 1h 9min
#58 Bayesian Modeling and Computation, with Osvaldo Martin, Ravin Kumar and Junpeng Lao
You know when you have friends who wrote a book and pressure you to come on your podcast? That’s super annoying, right?Well that’s not what happened with Ravin Kumar, Osvaldo Martin and Junpeng Lao — I was the one who suggested doing a special episode about their new book, Bayesian Modeling and Computation in Python. And since they cannot say no to my soothing French accent, well, they didn’t say no…All of them were on the podcast already, so I’ll refer you to their solo episode for background on their background — aka backgroundception.Junpeng is a Data Scientist at Google, living in Zurich, Switzerland. Previously, he was a post-doc in Psychology and Cognitive Neuroscience. His current obsessions are time series and state space models. Osvaldo is a Researcher at CONICET in Argentina and the Department of Computer Science from Aalto University in Finland. He is especially motivated by the development and implementation of software tools for Bayesian statistics and probabilistic modeling.Ravin is a data scientist at Google, living in Los Angeles. Previously he worked at Sweetgreen and SpaceX. He became interested in Bayesian statistics when trying to quantify uncertainty in operations. He is especially interested in decision science in business settings.You’ll make your own opinion, but I like their book because uses a hands-on approach, focusing on the practice of applied statistics. And you get to see how to use diverse libraries, like PyMC, Tensorflow Probability, ArviZ, Bambi, and so on. You’ll see what I’m talking about in this episode.To top it off, the book is fully available online at bayesiancomputationbook.com. If you want a physical copy (because you love those guys and wanna support them), go to CRC website and enter the code ASA18 at checkout for a 30% discount.Our theme music is « Good Bayesian », by Baba Brinkman (feat MC Lars and Mega Ran). Check out his awesome work at https://bababrinkman.com/ !Thank you to my Patrons for making this episode possible!Yusuke Saito, Avi Bryant, Ero Carrera, Giuliano Cruz, Tim Gasser, James Wade, Tradd Salvo, Adam Bartonicek, William Benton, Alan O'Donnell, Mark Ormsby, James Ahloy, Robin Taylor, Thomas Wiecki, Chad Scherrer, Nathaniel Neitzke, Zwelithini Tunyiswa, Elea McDonnell Feit, Bertrand Wilden, James Thompson, Stephen Oates, Gian Luca Di Tanna, Jack Wells, Matthew Maldonado, Ian Costley, Ally Salim, Larry Gill, Joshua Duncan, Ian Moran, Paul Oreto, Colin Caprani, George Ho, Colin Carroll, Nathaniel Burbank, Michael Osthege, Rémi Louf, Clive Edelsten, Henri Wallen, Hugo Botha, Vinh Nguyen, Raul Maldonado, Marcin Elantkowski, Adam C. Smith, Will Kurt, Andrew Moskowitz, Hector Munoz, Marco Gorelli, Simon Kessell, Bradley Rode, Patrick Kelley, Rick Anderson, Casper de Bruin, Philippe Labonde, Matthew McAnear, Michael Hankin, Cameron Smith, Luis Iberico, Tomáš Frýda, Ryan Wesslen, Andreas Netti, Riley King, Aaron Jones, Yoshiyuki Hamajima, Sven De Maeyer, Michael DeCrescenzo, Fergal M, Mason Yahr, Naoya Kanai, Steven Rowland and Aubrey Clayton.Visit https://www.patreon.com/learnbayesstats to unlock exclusive Bayesian swag ;)Links from the show:Website of the book: https://bayesiancomputationbook.com/welcome.htmlLBS #1 -- Bayes, open-source and bioinformatics, with Osvaldo Martin: https://www.learnbayesstats.com/episode/1-bayes-open-source-and-bioinformatics-with-osvaldo-martinOsvaldo on Twitter: https://twitter.com/aloctavodiaLBS #26 -- What you'll learn & who you'll meet at the PyMC Conference, with Ravin Kumar & Quan Nguyen: https://www.learnbayesstats.com/episode/26-what-youll-learn-who-youll-meet-at-the-pymc-conference-with-ravin-kumar-quan-nguyenRavin's blog: https://ravinkumar.com/Ravin on Twitter: https://twitter.com/canyon289LBS #7 -- Designing a Probabilistic Programming Language & Debugging a Model, with Junpeng Lao: https://www.learnbayesstats.com/episode/7-designing-a-probabilistic-programming-language-debugging-a-model-with-junpeng-laoJunpeng on Twitter: https://twitter.com/junpenglaoMatchmaking Dinner #1, with Will Kurt and Junpeng Lao: https://www.patreon.com/posts/48360540Donate to PyMC: https://numfocus.org/pymc-bayesian-book-formDonate to ArviZ: https://numfocus.org/arviz-bayesian-book-form

Mar 3, 2022 • 1h 22min
#57 Forecasting French Elections, with… Mystery Guest
No, no, don't leave! You did not click on the wrong button. You are indeed on Alex Andorra’s podcast. The podcast that took the Bayesian world by a storm: “Learning Bayesian Statistics”, and that Barack Obama deemed “the best podcast in the whole galaxy” – or maybe Alex said that, I don’t remember.Alex made us discover new methods, new ideas, and mostly new people. But what do we really know about him? Does he even really exist? To find this out I put on my Frenchest beret, a baguette under my arm, and went undercover to try to find him.And I did ! So today for a special episode I, Rémi Louf, will be the one asking questions and making bad jokes with a French accent.Before letting him in, here’s what I got on him so far.By day, Alex is a Bayesian modeler at the PyMC Labs consultancy. By night, he doesn’t (yet) fight crime but he’s an open-source enthusiast and core contributor to PyMC and ArviZ.An always-learning statistician, Alex loves building models and studying elections and human behavior.When he’s not working, he loves hiking, exercising, meditating and reading nerdy books and novels. He also loves chocolate a bit too much, but he doesn’t like talking about it – he prefers eating it.Our theme music is « Good Bayesian », by Baba Brinkman (feat MC Lars and Mega Ran). Check out his awesome work at https://bababrinkman.com/ !Thank you to my Patrons for making this episode possible!Yusuke Saito, Avi Bryant, Ero Carrera, Giuliano Cruz, Tim Gasser, James Wade, Tradd Salvo, Adam Bartonicek, William Benton, Alan O'Donnell, Mark Ormsby, James Ahloy, Robin Taylor, Thomas Wiecki, Chad Scherrer, Nathaniel Neitzke, Zwelithini Tunyiswa, Elea McDonnell Feit, Bertrand Wilden, James Thompson, Stephen Oates, Gian Luca Di Tanna, Jack Wells, Matthew Maldonado, Ian Costley, Ally Salim, Larry Gill, Joshua Duncan, Ian Moran, Paul Oreto, Colin Caprani, George Ho, Colin Carroll, Nathaniel Burbank, Michael Osthege, Rémi Louf, Clive Edelsten, Henri Wallen, Hugo Botha, Vinh Nguyen, Raul Maldonado, Marcin Elantkowski, Adam C. Smith, Will Kurt, Andrew Moskowitz, Hector Munoz, Marco Gorelli, Simon Kessell, Bradley Rode, Patrick Kelley, Rick Anderson, Casper de Bruin, Philippe Labonde, Matthew McAnear, Michael Hankin, Cameron Smith, Luis Iberico, Tomáš Frýda, Ryan Wesslen, Andreas Netti, Riley King, Aaron Jones, Yoshiyuki Hamajima, Sven De Maeyer, Michael DeCrescenzo, Fergal M, Mason Yahr, Naoya Kanai, Steven Rowland and Aubrey Clayton.Visit https://www.patreon.com/learnbayesstats to unlock exclusive Bayesian swag ;)Links from the show:Alex on Twitter: https://twitter.com/alex_andorraAlex on GitHub: https://github.com/AlexAndorraAlex on LinkedIn: https://www.linkedin.com/in/aandorra-pollsposition/Intuitive Bayes Introductory Course: https://www.intuitivebayes.com/PyMC Labs consultancy: https://www.pymc-labs.io/PollsPosition GitHub repository: https://github.com/pollspositionFrench Presidents' popularity dashboard: https://www.pollsposition.com/popularityLearning Bayesian Statistics YouTube channel: https://www.youtube.com/channel/UCAwVseuhVrpJFfik_cMHrhQLove the podcast? Leave a review on Podchaser: https://www.podchaser.com/podcasts/learning-bayesian-statistics-932588

14 snips
Feb 16, 2022 • 1h 9min
#56 Causal & Probabilistic Machine Learning, with Robert Osazuwa Ness
Did you know there is a relationship between the size of firetrucks and the amount of damage down to a flat during a fire? The bigger the truck sent to put out the fire, the bigger the damages tend to be. The solution is simple: just send smaller firetrucks!Wait, that doesn’t sound right, does it? Our brain is a huge causal machine, so it can instinctively feel it’s not credible that size of truck and amount of damage done are causally related: there must be another variable explaining the correlation. Here, it’s of course the seriousness of the fire — even better, it’s the common cause of the two correlated variables.Your brain does that automatically, but what about your computer? How do you make sure it doesn’t just happily (and mistakenly) report the correlation? That’s when causal inference and machine learning enter the stage, as Robert Osazuwa Ness will tell us.Robert has a PhD in statistics from Purdue University. He currently works as a Research Scientist at Microsoft Research and a founder of altdeep.ai, which teaches live cohort-based courses on advanced topics in applied modeling. As you’ll hear, his research focuses on the intersection of causal and probabilistic machine learning. Maybe that’s why I invited him on the show… Well, who knows, causal inference is very hard!Our theme music is « Good Bayesian », by Baba Brinkman (feat MC Lars and Mega Ran). Check out his awesome work at https://bababrinkman.com/ !Thank you to my Patrons for making this episode possible!Yusuke Saito, Avi Bryant, Ero Carrera, Giuliano Cruz, Tim Gasser, James Wade, Tradd Salvo, Adam Bartonicek, William Benton, Alan O'Donnell, Mark Ormsby, James Ahloy, Robin Taylor, Thomas Wiecki, Chad Scherrer, Nathaniel Neitzke, Zwelithini Tunyiswa, Elea McDonnell Feit, Bertrand Wilden, James Thompson, Stephen Oates, Gian Luca Di Tanna, Jack Wells, Matthew Maldonado, Ian Costley, Ally Salim, Larry Gill, Joshua Duncan, Ian Moran, Paul Oreto, Colin Caprani, George Ho, Colin Carroll, Nathaniel Burbank, Michael Osthege, Rémi Louf, Clive Edelsten, Henri Wallen, Hugo Botha, Vinh Nguyen, Raul Maldonado, Marcin Elantkowski, Adam C. Smith, Will Kurt, Andrew Moskowitz, Hector Munoz, Marco Gorelli, Simon Kessell, Bradley Rode, Patrick Kelley, Rick Anderson, Casper de Bruin, Philippe Labonde, Matthew McAnear, Michael Hankin, Cameron Smith, Luis Iberico, Tomáš Frýda, Ryan Wesslen, Andreas Netti, Riley King, Aaron Jones, Yoshiyuki Hamajima, Sven De Maeyer, Michael DeCrescenzo, Fergal M, Mason Yahr, Naoya Kanai, Steven Rowland and Aubrey Clayton.Visit https://www.patreon.com/learnbayesstats to unlock exclusive Bayesian swag ;)Links from the show:Robert's webpage: https://www.microsoft.com/en-us/research/people/robertness/Robert on Twitter: https://twitter.com/osazuwaRobert on GitHub: https://github.com/robertnessRobert on LinkedIn: https://www.linkedin.com/in/osazuwa/Do-calculus enables causal reasoning with latent variable models, Arxiv: https://arxiv.org/abs/2102.06626Integrating Markov processes with structural causal modeling enables counterfactual inference in complex systems, NeurIPS Proceedings: https://proceedings.neurips.cc/paper/2019/hash/2d44e06a7038f2dd98f0f54c4be35e22-Abstract.htmlCausality 101 with Robert Ness, The TWIML AI Podcast: https://www.youtube.com/watch?v=UNEZztT5lpkCausal Modeling in Machine Learning, PyData Boston: https://www.youtube.com/watch?v=1BioSmE5m6sPyro -- Deep Universal Probabilistic Programming: http://pyro.ai/Statistical Rethinking website: http://xcelab.net/rm/statistical-rethinking/The Book of Why -- The New Science of Cause and Effect : https://www.goodreads.com/book/show/36204378-the-book-of-whyThe Theory That Would Not Die -- How Bayes' Rule Cracked the Enigma Code : https://www.goodreads.com/book/show/10672848-the-theory-that-would-not-die

Jan 31, 2022 • 1h 14min
#55 Neuropsychology, Illusions & Bending Reality, with Dominique Makowski
What’s the common point between fiction, fake news, illusions and meditation? They can all be studied with Bayesian statistics, of course!In this mind-bending episode, Dominique Makowski will for sure expand your horizon. Trained as a clinical neuropsychologist, he is currently working as a postdoc at the Clinical Brain Lab in Singapore, in which he leads the Reality Bending Team. What’s reality-bending you ask? Well, you’ll have to listen to the episode, but I can already tell you we’ll go through a journey in scientific methodology, history of art, religion, and philosophy — what else?Beyond that, Dominique tries to improve the access to advanced analysis techniques by developing open-source software and tools, like the NeuroKit Python package or the bayestestR package in R.Even better, he looks a lot like his figures of reference. Like Marcus Aurelius, he plays the piano and guitar. Like Sisyphus, he loves history of art and comparative mythology. And like Yoda, he is a wakeboard master.Our theme music is « Good Bayesian », by Baba Brinkman (feat MC Lars and Mega Ran). Check out his awesome work at https://bababrinkman.com/ !Thank you to my Patrons for making this episode possible!Yusuke Saito, Avi Bryant, Ero Carrera, Brian Huey, Giuliano Cruz, Tim Gasser, James Wade, Tradd Salvo, Adam Bartonicek, William Benton, Alan O'Donnell, Mark Ormsby, Demetri Pananos, James Ahloy, Robin Taylor, Thomas Wiecki, Chad Scherrer, Nathaniel Neitzke, Zwelithini Tunyiswa, Elea McDonnell Feit, Bertrand Wilden, James Thompson, Stephen Oates, Gian Luca Di Tanna, Jack Wells, Matthew Maldonado, Ian Costley, Ally Salim, Larry Gill, Joshua Duncan, Ian Moran, Paul Oreto, Colin Caprani, George Ho, Colin Carroll, Nathaniel Burbank, Michael Osthege, Rémi Louf, Clive Edelsten, Henri Wallen, Hugo Botha, Vinh Nguyen, Raul Maldonado, Marcin Elantkowski, Adam C. Smith, Will Kurt, Andrew Moskowitz, Hector Munoz, Marco Gorelli, Simon Kessell, Bradley Rode, Patrick Kelley, Rick Anderson, Casper de Bruin, Philippe Labonde, Matthew McAnear, Michael Hankin, Cameron Smith, Luis Iberico, Tomáš Frýda, Ryan Wesslen, Andreas Netti, Riley King, Aaron Jones, Daniel Lindroth, Yoshiyuki Hamajima, Sven De Maeyer and Michael DeCrescenzo.Visit https://www.patreon.com/learnbayesstats to unlock exclusive Bayesian swag ;)Links from the show:To follow:Dominique's website: https://dominiquemakowski.github.io/Dominique on Twitter: https://twitter.com/Dom_MakowskiDominique on GitHub: https://github.com/DominiqueMakowskiPackages:NeuroKit -- Python Toolbox for Neurophysiological Signal Processing: https://github.com/neuropsychology/NeuroKitbayestestR -- Become a Bayesian master you will: https://easystats.github.io/bayestestR/report -- From R to your manuscript: https://easystats.github.io/report/Research:The Reality Bending League :https://realitybending.github.io/research/What is Reality Bending: https://realitybending.github.io/post/2020-09-28-what_is_realitybending/Art:NeuropsyXart -- Neuroimaging methods to obtain visual representations of neurophysiological processes: https://dominiquemakowski.github.io/NeuropsyXart/

Jan 14, 2022 • 1h 9min
#54 Bayes in Theoretical Ecology, with Florian Hartig
Let’s be honest: evolution is awesome! I started reading Improbable Destinies: Fate, Chance, and the Future of Evolution, by Jonathan Losos, and I’m utterly fascinated. So I’m thrilled to welcome Florian Hartig on the show. Florian is a professor of Theoretical Ecology at the University of Regensburg, Germany. His research concentrates on theory, computer simulations, statistical methods and machine learning in ecology & evolution. He is also interested in open science and open software development, and maintains, among other projects, the R packages DHARMa and BayesianTools.Among other things, we talked about approximate Bayesian computation, best practices when building models and the big pain points that remain in the Bayesian pipeline.Most importantly, Florian’s main hobbies are whitewater kayaking, snowboarding, badminton and playing the guitar.Our theme music is « Good Bayesian », by Baba Brinkman (feat MC Lars and Mega Ran). Check out his awesome work at https://bababrinkman.com/ !Thank you to my Patrons for making this episode possible!Yusuke Saito, Avi Bryant, Ero Carrera, Brian Huey, Giuliano Cruz, Tim Gasser, James Wade, Tradd Salvo, Adam Bartonicek, William Benton, Alan O'Donnell, Mark Ormsby, Demetri Pananos, James Ahloy, Robin Taylor, Thomas Wiecki, Chad Scherrer, Nathaniel Neitzke, Zwelithini Tunyiswa, Elea McDonnell Feit, Bertrand Wilden, James Thompson, Stephen Oates, Gian Luca Di Tanna, Jack Wells, Matthew Maldonado, Ian Costley, Ally Salim, Larry Gill, Joshua Duncan, Ian Moran, Paul Oreto, Colin Caprani, George Ho, Colin Carroll, Nathaniel Burbank, Michael Osthege, Rémi Louf, Clive Edelsten, Henri Wallen, Hugo Botha, Vinh Nguyen, Raul Maldonado, Marcin Elantkowski, Adam C. Smith, Will Kurt, Andrew Moskowitz, Hector Munoz, Marco Gorelli, Simon Kessell, Bradley Rode, Patrick Kelley, Rick Anderson, Casper de Bruin, Philippe Labonde, Matthew McAnear, Michael Hankin, Cameron Smith, Luis Iberico, Tomáš Frýda, Ryan Wesslen, Andreas Netti, Riley King, Aaron Jones and Daniel Lindroth.Visit https://www.patreon.com/learnbayesstats to unlock exclusive Bayesian swag ;)Links from the show:Florian's website: https://theoreticalecology.wordpress.com/Florian on Twitter: https://twitter.com/florianhartigFlorian on GitHub: https://github.com/florianhartigDHARMa -- Residual Diagnostics for Hierarchical Regression Models: https://cran.r-project.org/web/packages/DHARMa/index.htmlBayesianTools -- General-Purpose MCMC and SMC Samplers and Tools for Bayesian Statistics: https://cran.r-project.org/web/packages/BayesianTools/index.htmlStatistical inference for stochastic simulation inference -- theory and application: https://onlinelibrary.wiley.com/doi/epdf/10.1111/j.1461-0248.2011.01640.xArviZ plot rank function: https://arviz-devs.github.io/arviz/api/generated/arviz.plot_rank.htmlRank-normalization, folding, and localization -- An improved R-hat for assessing convergence of MCMC: https://arxiv.org/abs/1903.08008LBS #51 Bernoulli's Fallacy & the Crisis of Modern Science, with Aubrey Clayton: https://www.learnbayesstats.com/episode/51-bernoullis-fallacy-crisis-modern-science-aubrey-claytonLBS #50 Ta(l)king Risks & Embracing Uncertainty, with David Spiegelhalter: https://www.learnbayesstats.com/episode/50-talking-risks-embracing-uncertainty-david-spiegelhalterLBS #44 Building Bayesian Models at scale, with Rémi Louf: https://www.learnbayesstats.com/episode/44-bayesian-models-at-scale-remi-loufLBS #35 The Past, Present & Future of BRMS, with Paul Bürkner: https://www.learnbayesstats.com/episode/35-past-present-future-brms-paul-burknerLBS #29 Model Assessment, Non-Parametric Models, And Much More, with Aki Vehtari: https://www.learnbayesstats.com/episode/model-assessment-non-parametric-models-aki-vehtariImprobable Destinies -- Fate, Chance, and the Future of Evolution: https://www.goodreads.com/book/show/33357463-improbable-destinies

Dec 28, 2021 • 56min
#53 Bayesian Stats for the Behavioral & Neural Sciences, with Todd Hudson
Get a 30% discount on Todd's book by entering the code BDABNS22 at checkout!The behavioral and neural sciences are a nerdy interest of mine, but I didn’t dedicate any episode to that topic yet. But life brings you gifts sometimes (especially around Christmas…), and here that gift is a book, Bayesian Data Analysis for the Behavioral and Neural Sciences, by Todd Hudson.Todd is a part of the faculty at New York University Grossman School of Medicine and also the New York University Tandon School of Engineering. He is a computational neuroscientist working in several areas including: early detection and grading of neurological disease; computational models of movement planning and learning; development of new computational and experimental techniques. He also co-founded Tactile Navigation Tools, which develops navigation aids for the visually impaired, and Third Eye Technologies, which develops low cost laboratory- and clinical-grade eyetracking technologies.As you’ll hear, Todd wanted his book to bypass the need for advanced mathematics normally considered a prerequisite for this type of material. Basically, he wants students to be able to write code and models and understand equations, even they are not specialized in writing those equations.We’ll also touch on some of the neural sciences examples he’s got in the book, as well as the two general algorithms he uses for model measurement and model selection.Ow, I almost forgot the most important: Todd loves beekeeping and gardening — he’s got 25 apple trees, 4 cherry trees, nectarines, figs, strawberries, etc!Our theme music is « Good Bayesian », by Baba Brinkman (feat MC Lars and Mega Ran). Check out his awesome work at https://bababrinkman.com/ !Thank you to my Patrons for making this episode possible!Yusuke Saito, Avi Bryant, Ero Carrera, Brian Huey, Giuliano Cruz, Tim Gasser, James Wade, Tradd Salvo, Adam Bartonicek, William Benton, Alan O'Donnell, Mark Ormsby, Demetri Pananos, James Ahloy, Robin Taylor, Thomas Wiecki, Chad Scherrer, Nathaniel Neitzke, Zwelithini Tunyiswa, Elea McDonnell Feit, Bertrand Wilden, James Thompson, Stephen Oates, Gian Luca Di Tanna, Jack Wells, Matthew Maldonado, Ian Costley, Ally Salim, Larry Gill, Joshua Duncan, Ian Moran, Paul Oreto, Colin Caprani, George Ho, Colin Carroll, Nathaniel Burbank, Michael Osthege, Rémi Louf, Clive Edelsten, Henri Wallen, Hugo Botha, Vinh Nguyen, Raul Maldonado, Marcin Elantkowski, Adam C. Smith, Will Kurt, Andrew Moskowitz, Hector Munoz, Marco Gorelli, Simon Kessell, Bradley Rode, Patrick Kelley, Rick Anderson, Casper de Bruin, Philippe Labonde, Matthew McAnear, Michael Hankin, Cameron Smith, Luis Iberico, Alejandro Morales, Tomáš Frýda, Ryan Wesslen, Andreas Netti, Riley King, Aaron Jones and Daniel Lindroth.Visit https://www.patreon.com/learnbayesstats to unlock exclusive Bayesian swag ;)Links from the show:30% discount on Todd's book by entering BDABNS22 at checkout: https://www.cambridge.org/it/academic/subjects/psychology/psychology-general-interest/bayesian-data-analysis-behavioral-and-neural-sciences-non-calculus-fundamentals?format=PB&isbn=9781108812900Book's webpage: https://www.hudsonlab.org/textbookFor blurbs on each chapter: https://www.hudsonlab.org/textbookresourcesCode used in each chapter: https://www.hudsonlab.org/textbook/f314aFor tutorials on Bayesian vs. classical frequentist data analysis: https://www.hudsonlab.org/datatutorialsTodd's research: https://www.hudsonlab.org/researchTodd's webpage: https://med.nyu.edu/faculty/todd-e-hudsonFor information on Todd's assistive technology work: feeltnt.com

Dec 9, 2021 • 58min
#52 Election forecasting models in Germany, with Marcus Gross
Did I mention I like survey data, especially in the context of electoral forecasting? Probably not, as I’m a pretty shy and reserved man. Why are you laughing?? Yeah, that’s true, I’m not that shy… but I did mention my interest for electoral forecasting already!And before doing a full episode where I’ll talk about French elections (yes, that’ll come at one point), let’s talk about one of France’s neighbors — Germany. Our German friends had federal elections a few weeks ago — consequential elections, since they had the hard task of replacing Angela Merkel, after 16 years in power.To talk about this election, I invited Marcus Gross on the show, because he worked on a Bayesian forecasting model to try and predict the results of this election — who will get elected as Chancellor, by how much and with which coalition?I was delighted to ask him about how the model works, how it accounts for the different sources of uncertainty — be it polling errors, unexpected turnout or media events — and, of course, how long it takes to sample (I think you’ll be surprised by the answer). We also talked about the other challenge of this kind of work: communication — how do you communicate uncertainty effectively? How do you differentiate motivated reasoning from useful feedback? What were the most common misconceptions about the model?Marcus studied statistics in Munich and Berlin, and did a PhD on survey statistics and measurement error models in economics and archeology. He worked as a data scientist at INWT, a consulting firm with projects in different business fields as well as the public sector. Now, he is working at FlixMobility.Our theme music is « Good Bayesian », by Baba Brinkman (feat MC Lars and Mega Ran). Check out his awesome work at https://bababrinkman.com/ !Thank you to my Patrons for making this episode possible!Yusuke Saito, Avi Bryant, Ero Carrera, Brian Huey, Giuliano Cruz, Tim Gasser, James Wade, Tradd Salvo, Adam Bartonicek, William Benton, Alan O'Donnell, Mark Ormsby, Demetri Pananos, James Ahloy, Robin Taylor, Thomas Wiecki, Chad Scherrer, Nathaniel Neitzke, Zwelithini Tunyiswa, Elea McDonnell Feit, Bertrand Wilden, James Thompson, Stephen Oates, Gian Luca Di Tanna, Jack Wells, Matthew Maldonado, Ian Costley, Ally Salim, Larry Gill, Joshua Duncan, Ian Moran, Paul Oreto, Colin Caprani, George Ho, Colin Carroll, Nathaniel Burbank, Michael Osthege, Rémi Louf, Clive Edelsten, Henri Wallen, Hugo Botha, Vinh Nguyen, Raul Maldonado, Marcin Elantkowski, Adam C. Smith, Will Kurt, Andrew Moskowitz, Hector Munoz, Marco Gorelli, Simon Kessell, Bradley Rode, Patrick Kelley, Rick Anderson, Casper de Bruin, Philippe Labonde, Matthew McAnear, Michael Hankin, Cameron Smith, Luis Iberico, Alejandro Morales, Tomáš Frýda, Ryan Wesslen, Andreas Netti, Riley King and Aaron Jones.Visit https://www.patreon.com/learnbayesstats to unlock exclusive Bayesian swag ;)Links from the show:German election forecast website: https://www.wer-gewinnt-die-wahl.de/enTwitter account of electoral model: https://twitter.com/GerElectionFcstGerman election model code: https://github.com/INWTlab/lsTerm-election-forecastLBS #27 -- Modeling the US Presidential Elections, with Andrew Gelman & Merlin Heidemanns: https://www.learnbayesstats.com/episode/27-modeling-the-us-presidential-elections-with-andrew-gelman-merlin-heidemanns

4 snips
Nov 22, 2021 • 1h 9min
#51 Bernoulli’s Fallacy & the Crisis of Modern Science, with Aubrey Clayton
Aubrey Clayton, a mathematician at Harvard Extension School, explores the shortcomings of standard statistical methods and their impact on various fields, from science to law. He discusses his book, "Bernoulli's Fallacy," revealing a critical error in logic that contributes to the replication crisis in modern science. Clayton highlights the transformative power of Bayesian thinking and shares insights from E.T. Jaynes, advocating for a shift in how we understand and apply probability. He emphasizes the necessity of incorporating prior knowledge to improve decision-making and scientific inference.

7 snips
Nov 6, 2021 • 1h 4min
#50 Ta(l)king Risks & Embracing Uncertainty, with David Spiegelhalter
Folks, this is the 50th episode of LBS — 50th! I never would have thought that there were so many Bayesian nerds in the world when I first interviewed Osvaldo Martin more than 2 years ago. To celebrate that random, crazy adventure, I wanted to do a special episode at any random point, and so it looks like it’s gonna be #50! This episode is special by its guest, not its number — although my guest knows a thing or two about numbers. Most recently, he wrote the book Covid by Numbers.A mathematical statistician dedicated to helping the general public understand risk, uncertainty and decision-making, he’s the author of several books on the topic actually, including The Art of Statistics. You may also know him from his podcast, Risky Talk, or his numerous appearances in newspapers, radio and TV shows.Did you guess who it is?Maybe you just know him as the reigning World Champion in Loop – a version of pool played on an elliptical table – and are just discovering now that he is a fantastic science communicator – something that turns out to be especially important for stats education in times of, let’s say, global pandemic for instance.He holds a PhD in Mathematical Statistics from the University of London and has been the Chair of the Winton Centre for Risk and Evidence Communication at Cambridge University since 2016. He was also the President of the famous Royal Statistical Society in 2017-2018.Most importantly, he was featured in BBC1’s Winter Wipeout in 2011 – seriously, go check it out on his website; it’s hilarious.So did you guess it yet? Yep, my guest for this episode is no other than Sir David Spiegelhalter — yes, there are Bayesian knights!Our theme music is « Good Bayesian », by Baba Brinkman (feat MC Lars and Mega Ran). Check out his awesome work at https://bababrinkman.com/ !Thank you to my Patrons for making this episode possible!Yusuke Saito, Avi Bryant, Ero Carrera, Brian Huey, Giuliano Cruz, Tim Gasser, James Wade, Tradd Salvo, Adam Bartonicek, William Benton, Alan O'Donnell, Mark Ormsby, Demetri Pananos, James Ahloy, Jon Berezowski, Robin Taylor, Thomas Wiecki, Chad Scherrer, Nathaniel Neitzke, Zwelithini Tunyiswa, Elea McDonnell Feit, Bertrand Wilden, James Thompson, Stephen Oates, Gian Luca Di Tanna, Jack Wells, Matthew Maldonado, Ian Costley, Ally Salim, Larry Gill, Joshua Duncan, Ian Moran, Paul Oreto, Colin Caprani, George Ho, Colin Carroll, Nathaniel Burbank, Michael Osthege, Rémi Louf, Clive Edelsten, Henri Wallen, Hugo Botha, Vinh Nguyen, Raul Maldonado, Marcin Elantkowski, Tim Radtke, Adam C. Smith, Will Kurt, Andrew Moskowitz, Hector Munoz, Marco Gorelli, Simon Kessell, Bradley Rode, Patrick Kelley, Rick Anderson, Casper de Bruin, Philippe Labonde, Matthew McAnear, Michael Hankin, Cameron Smith, Luis Iberico, Alejandro Morales and Tomáš Frýda.Visit https://www.patreon.com/learnbayesstats to unlock exclusive Bayesian swag ;)Links from the show:David's website: http://www.statslab.cam.ac.uk/~david/David on Twitter: https://twitter.com/d_spiegelThe Art of Statistics: https://dspiegel29.github.io/ArtofStatistics/Risky Talk podcast: https://riskytalk.libsyn.com/Winton Centre for Risk and Evidence Communication: https://wintoncentre.maths.cam.ac.uk/Frank Ramsey -- A Sheer Excess of Powers: https://www.amazon.fr/Frank-Ramsey-Sheer-Excess-Powers/dp/019875535XBBC Radio 4, David Spiegelhalter on Frank Ramsey: https://www.bbc.co.uk/programmes/m000q8pqDe Finetti's theorem: https://en.wikipedia.org/wiki/De_Finetti%27s_theoremLaplace's demon: https://en.wikipedia.org/wiki/Laplace%27s_demonGame of Loop: http://www.loop-the-game.com/scoop/