
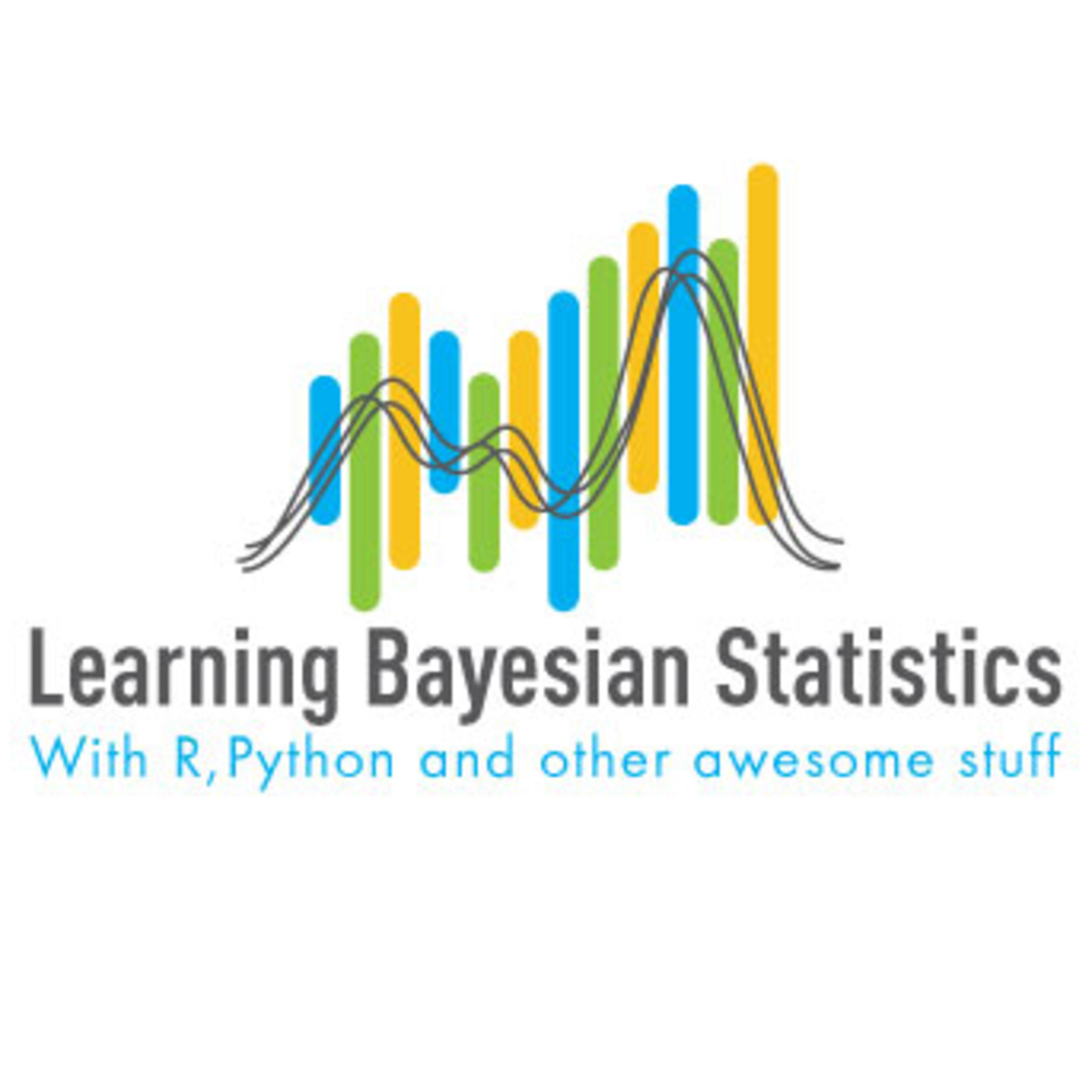
Learning Bayesian Statistics
Alexandre Andorra
Are you a researcher or data scientist / analyst / ninja? Do you want to learn Bayesian inference, stay up to date or simply want to understand what Bayesian inference is?
Then this podcast is for you! You'll hear from researchers and practitioners of all fields about how they use Bayesian statistics, and how in turn YOU can apply these methods in your modeling workflow.
When I started learning Bayesian methods, I really wished there were a podcast out there that could introduce me to the methods, the projects and the people who make all that possible.
So I created "Learning Bayesian Statistics", where you'll get to hear how Bayesian statistics are used to detect black matter in outer space, forecast elections or understand how diseases spread and can ultimately be stopped.
But this show is not only about successes -- it's also about failures, because that's how we learn best. So you'll often hear the guests talking about what *didn't* work in their projects, why, and how they overcame these challenges. Because, in the end, we're all lifelong learners!
My name is Alex Andorra by the way, and I live in Estonia. By day, I'm a data scientist and modeler at the https://www.pymc-labs.io/ (PyMC Labs) consultancy. By night, I don't (yet) fight crime, but I'm an open-source enthusiast and core contributor to the python packages https://docs.pymc.io/ (PyMC) and https://arviz-devs.github.io/arviz/ (ArviZ). I also love https://www.pollsposition.com/ (election forecasting) and, most importantly, Nutella. But I don't like talking about it – I prefer eating it.
So, whether you want to learn Bayesian statistics or hear about the latest libraries, books and applications, this podcast is for you -- just subscribe! You can also support the show and https://www.patreon.com/learnbayesstats (unlock exclusive Bayesian swag on Patreon)!
Then this podcast is for you! You'll hear from researchers and practitioners of all fields about how they use Bayesian statistics, and how in turn YOU can apply these methods in your modeling workflow.
When I started learning Bayesian methods, I really wished there were a podcast out there that could introduce me to the methods, the projects and the people who make all that possible.
So I created "Learning Bayesian Statistics", where you'll get to hear how Bayesian statistics are used to detect black matter in outer space, forecast elections or understand how diseases spread and can ultimately be stopped.
But this show is not only about successes -- it's also about failures, because that's how we learn best. So you'll often hear the guests talking about what *didn't* work in their projects, why, and how they overcame these challenges. Because, in the end, we're all lifelong learners!
My name is Alex Andorra by the way, and I live in Estonia. By day, I'm a data scientist and modeler at the https://www.pymc-labs.io/ (PyMC Labs) consultancy. By night, I don't (yet) fight crime, but I'm an open-source enthusiast and core contributor to the python packages https://docs.pymc.io/ (PyMC) and https://arviz-devs.github.io/arviz/ (ArviZ). I also love https://www.pollsposition.com/ (election forecasting) and, most importantly, Nutella. But I don't like talking about it – I prefer eating it.
So, whether you want to learn Bayesian statistics or hear about the latest libraries, books and applications, this podcast is for you -- just subscribe! You can also support the show and https://www.patreon.com/learnbayesstats (unlock exclusive Bayesian swag on Patreon)!
Episodes
Mentioned books

Oct 22, 2021 • 1h 13min
#49 The Present & Future of Baseball Analytics, with Ehsan Bokhari
It’s been a while since I did an episode about sports analytics, right? And you know it’s a field I love, so… let’s do that!For this episode, I was happy to host Ehsan Bokhari, not only because he’s a first-hour listener of the podcast and spread the word about it whenever he can, but mainly because he knows baseball analytics very well!Currently Senior Director of Strategic Decision Making with the Houston Astros, he previously worked there as Senior Director of Player Evaluation and Director of R&D. And before that, he was Senior Director at the Los Angeles Dodgers from the 2015 to the 2018 season.Among other things, we talked about what his job looks like, how Bayesian the field is, which pushbacks he gets, and what the future of baseball analytics look like to him.Ehsan also has an interesting background, coming from both psychology and mathematics. Indeed, he received a PhD in quantitative psychology and an MS in statistics at the University of Illinois in 2014.Maybe most importantly, he loves reading non-fiction and spending time with his almost three-year-old son — who he read Bayesian Probability for Babies to, of course.Our theme music is « Good Bayesian », by Baba Brinkman (feat MC Lars and Mega Ran). Check out his awesome work at https://bababrinkman.com/ !Thank you to my Patrons for making this episode possible!Yusuke Saito, Avi Bryant, Ero Carrera, Brian Huey, Giuliano Cruz, Tim Gasser, James Wade, Tradd Salvo, Adam Bartonicek, William Benton, Alan O'Donnell, Mark Ormsby, Demetri Pananos, James Ahloy, Jon Berezowski, Robin Taylor, Thomas Wiecki, Chad Scherrer, Nathaniel Neitzke, Zwelithini Tunyiswa, Elea McDonnell Feit, Bertrand Wilden, James Thompson, Stephen Oates, Gian Luca Di Tanna, Jack Wells, Matthew Maldonado, Ian Costley, Ally Salim, Larry Gill, Joshua Duncan, Ian Moran, Paul Oreto, Colin Caprani, George Ho, Colin Carroll, Nathaniel Burbank, Michael Osthege, Rémi Louf, Clive Edelsten, Henri Wallen, Hugo Botha, Vinh Nguyen, Raul Maldonado, Marcin Elantkowski, Tim Radtke, Adam C. Smith, Will Kurt, Andrew Moskowitz, Hector Munoz, Marco Gorelli, Simon Kessell, Bradley Rode, Patrick Kelley, Rick Anderson, Casper de Bruin, Philippe Labonde, Matthew McAnear, Michael Hankin, Cameron Smith, Luis Iberico, and Alejandro Morales.Visit https://www.patreon.com/learnbayesstats to unlock exclusive Bayesian swag ;)Links from the show:Ehsan on LinkedIn: https://www.linkedin.com/in/ebokhari/Bayesian Bagging to Generate Uncertainty Intervals -- A Catcher Framing Story: https://www.baseballprospectus.com/news/article/38289/bayesian-bagging-generate-uncertainty-intervals-catcher-framing-story/ Jim Albert's Bayesball blog: https://bayesball.github.io/Simulation of empirical Bayesian methods, using baseball statistics: http://varianceexplained.org/r/simulation-bayes-baseball/Detection and Characterization of Cluster Substructure -- Fuzzy c-Lines: https://epubs.siam.org/doi/abs/10.1137/0140029Tensor rank decomposition: https://en.wikipedia.org/wiki/Tensor_rank_decompositionStatistical Prediction versus Clinical Prediction -- Improving What Works: https://meehl.umn.edu/sites/meehl.umn.edu/files/files/155dfm1993.pdfClinical Versus Actuarial Judgment: https://meehl.umn.edu/sites/meehl.umn.edu/files/files/138cstixdawesfaustmeehl.pdfClinical Versus Statistical Prediction: https://citeseerx.ist.psu.edu/viewdoc/download?doi=10.1.1.693.6031&rep=rep1&type=pdf

Oct 8, 2021 • 1h 1min
#48 Mixed Effects Models & Beautiful Plots, with TJ Mahr
In episode 40, we already got a glimpse of how useful Bayesian stats are in the speech and communication sciences. To talk about the frontiers of this field (and, as it happens, about best practices to make beautiful plots and pictures), I invited TJ Mahr on the show.A speech pathologist turned data scientist, TJ earned his PhD in communication sciences and disorders in Madison, Wisconsin. On paper, he was studying speech development, word recognition and word learning in preschoolers, but over the course of his graduate training, he discovered that he really, really likes programming and working with data – we’ll of course talk about that in the show!In short, TJ wrangles data, crunches numbers, plots pictures, and fits models to study how children learn to speak and communicate. On his website, he often writes about Bayesian models, mixed effects models, functional programming in R, or how to plot certain kinds of data.He also got very into the deck-building game “Slay the Spire” this year, and his favorite youtube channel is a guy who restores paintings.Our theme music is « Good Bayesian », by Baba Brinkman (feat MC Lars and Mega Ran). Check out his awesome work at https://bababrinkman.com/ !Thank you to my Patrons for making this episode possible!Yusuke Saito, Avi Bryant, Ero Carrera, Brian Huey, Giuliano Cruz, Tim Gasser, James Wade, Tradd Salvo, Adam Bartonicek, William Benton, Alan O'Donnell, Mark Ormsby, Demetri Pananos, James Ahloy, Jon Berezowski, Robin Taylor, Thomas Wiecki, Chad Scherrer, Nathaniel Neitzke, Zwelithini Tunyiswa, Elea McDonnell Feit, Bertrand Wilden, James Thompson, Stephen Oates, Gian Luca Di Tanna, Jack Wells, Matthew Maldonado, Ian Costley, Ally Salim, Larry Gill, Joshua Duncan, Ian Moran, Paul Oreto, Colin Caprani, George Ho, Colin Carroll, Nathaniel Burbank, Michael Osthege, Rémi Louf, Clive Edelsten, Henri Wallen, Hugo Botha, Vinh Nguyen, Raul Maldonado, Marcin Elantkowski, Tim Radtke, Adam C. Smith, Will Kurt, Andrew Moskowitz, Hector Munoz, Marco Gorelli, Simon Kessell, Bradley Rode, Patrick Kelley, Rick Anderson, Casper de Bruin, Philippe Labonde, Matthew McAnear, Michael Hankin, Cameron Smith, and Luis Iberico.Visit https://www.patreon.com/learnbayesstats to unlock exclusive Bayesian swag ;)Links from the show:TJ's website: https://www.tjmahr.com/TJ on Twitter: https://twitter.com/tjmahrTJ on GitHub: https://github.com/tjmahrLBS #40, Bayesian Stats for the Speech & Language Sciences: https://www.learnbayesstats.com/episode/40-bayesian-stats-speech-language-sciences-allison-hilger-timo-roettgerRandom Effects and Penalized Splines: https://www.tjmahr.com/random-effects-penalized-splines-same-thing/Bayes’s theorem in three panels: https://www.tjmahr.com/bayes-theorem-in-three-panels/Another mixed effects model visualization: https://www.tjmahr.com/another-mixed-effects-model-visualization/Anatomy of a logistic growth curve: https://www.tjmahr.com/anatomy-of-a-logistic-growth-curve/R Users Will Now Inevitably Become Bayesians: https://thinkinator.com/2016/01/12/r-users-will-now-inevitably-become-bayesians/Wisconsin Intelligibility, Speech, and Communication Laboratory: https://kidspeech.wisc.edu/Longitudinal Growth in Intelligibility of Connected Speech From 2 to 8 Years in Children With Cerebral Palsy: https://pubs.asha.org/doi/abs/10.1044/2020_JSLHR-20-00181Statistics for Hackers: https://speakerdeck.com/jakevdp/statistics-for-hackersStructure and interpretation of computer programs: https://mitpress.mit.edu/sites/default/files/sicp/index.htmlLectures for structure and interpretation of computer programs from 1986 (that are still very good): https://www.youtube.com/playlist?list=PLE18841CABEA24090bayesplot: https://mc-stan.org/bayesplot/ggdist: https://mjskay.github.io/ggdist/brms: https://paul-buerkner.github.io/brms/targets: https://books.ropensci.org/targets/mgcv: https://cran.r-project.org/web/packages/mgcv/index.htmllme4: https://cran.r-project.org/web/packages/lme4/index.html

Sep 21, 2021 • 1h 16min
#47 Bayes in Physics & Astrophysics, with JJ Ruby
The field of physics has brought tremendous advances to modern Bayesian statistics, especially inspiring the current algorithms enabling all of us to enjoy the Bayesian power on our own laptops.I did receive some physicians already on the show, like Michael Betancourt in episode 6, but in my legendary ungratefulness I hadn’t dedicated a whole episode to talk about physics yet.Well that’s now taken care of, thanks to JJ Ruby. Apart from having really good tastes (he’s indeed a fan of this very podcast), JJ is currently a postdoctoral fellow for the Center for Matter at Atomic Pressures at the University of Rochester, and will soon be starting as a Postdoctoral Scholar at Lawrence Livermore National Laboratory, a U.S. Department of Energy National Laboratory.JJ did his undergraduate work in Astrophysics and Planetary Science at Villanova University, outside of Philadelphia, and completed his master’s degree and PhD in Physics at the University of Rochester, in New York.JJ studies high energy density physics and focuses on using Bayesian techniques to extract information from large scale physics experiments with highly integrated measurements.In his freetime, he enjoys playing sports including baseball, basketball, and golf.Our theme music is « Good Bayesian », by Baba Brinkman (feat MC Lars and Mega Ran). Check out his awesome work at https://bababrinkman.com/ !Thank you to my Patrons for making this episode possible!Yusuke Saito, Avi Bryant, Ero Carrera, Brian Huey, Giuliano Cruz, Tim Gasser, James Wade, Tradd Salvo, Adam Bartonicek, William Benton, Alan O'Donnell, Mark Ormsby, Demetri Pananos, James Ahloy, Jon Berezowski, Robin Taylor, Thomas Wiecki, Chad Scherrer, Nathaniel Neitzke, Zwelithini Tunyiswa, Elea McDonnell Feit, Bertrand Wilden, James Thompson, Stephen Oates, Gian Luca Di Tanna, Jack Wells, Matthew Maldonado, Ian Costley, Ally Salim, Larry Gill, Joshua Duncan, Ian Moran, Paul Oreto, Colin Caprani, George Ho, Colin Carroll, Nathaniel Burbank, Michael Osthege, Rémi Louf, Clive Edelsten, Henri Wallen, Hugo Botha, Vinh Nguyen, Raul Maldonado, Marcin Elantkowski, Tim Radtke, Adam C. Smith, Will Kurt, Andrew Moskowitz, Hector Munoz, Marco Gorelli, Simon Kessell, Bradley Rode, Patrick Kelley, Rick Anderson, Casper de Bruin, Philippe Labonde, Matthew McAnear, Michael Hankin and Cameron Smith.Visit https://www.patreon.com/learnbayesstats to unlock exclusive Bayesian swag ;)Links from the show:Center for Matter at Atomic Pressures: https://www.rochester.edu/cmap/Laboratory for Laser Energetics: https://www.lle.rochester.edu/index.php/about-the-laboratory-for-laser-energetics/Lawrence Livermore National Laboratory: https://www.llnl.gov/JJ's thesis -- Bayesian Inference of Fundamental Physics at Extreme Conditions: https://www.lle.rochester.edu/media/publications/documents/theses/Ruby.pdfRecent Fusion Breakthrough: https://www.llnl.gov/news/national-ignition-facility-experiment-puts-researchers-threshold-fusion-ignitionLBS #6, A principled Bayesian workflow, with Michael Betancourt: https://www.learnbayesstats.com/episode/6-a-principled-bayesian-workflow-with-michael-betancourt20 Best Statistics Podcasts of 2021: https://welpmagazine.com/20-best-statistics-podcasts-of-2021/E.T. Jaynes, Probability Theory -- The Logic of Science: https://www.goodreads.com/book/show/151848.Probability_TheoryD.S. Sivia, Data Analysis -- A Bayesian Tutorial: http://aprsa.villanova.edu/files/sivia.pdfS. Chandrasekhar -- An Introduction to the Study of Stellar Structure: https://www.amazon.com/Introduction-Study-Stellar-Structure-Astronomy/dp/0486604136Fun quote (from preface in Jaynes' book): Therefore we think that in the future, workers in all the quantitative sciences will be obliged, as a matter of practical necessity, to use probability theory in the manner expounded here. This trend is already well under way in several fields, ranging from econometrics to astronomy to magnetic resonance spectroscopy; but to make progress in a new area it is necessary to develop a healthy disrespect for tradition and authority, which have retarded progress throughout the 20th century.

Aug 30, 2021 • 1h 13min
#46 Silly & Empowering Statistics, with Chelsea Parlett-Pelleriti
You wanna know something funny? A sentence from this episode became a meme. And people even made stickers out of it! Ok, that’s not true. But if someone could pull off something like that, it would surely be Chelsea Parlett-Pelleriti.Indeed, Chelsea’s research focuses on using statistics and machine learning on behavioral data, but her more general goal is to empower people to be able to do their own statistical analyses, through consulting, education, and, as you may have seen, stats memes on Twitter.A full-time teacher, researcher and statistical consultant, Chelsea earned an MsC and PhD in Computational and Data Science in 2021 from Chapman University. Her courses include R, intro to programming (in Python), and data science.In a nutshell, Chelsea is, by her own admission, an avid lover of anything silly or statistical. Hopefully, this episode turned out to be both at once! I’ll let you be the judge of that…Our theme music is « Good Bayesian », by Baba Brinkman (feat MC Lars and Mega Ran). Check out his awesome work at https://bababrinkman.com/ !Thank you to my Patrons for making this episode possible!Yusuke Saito, Avi Bryant, Ero Carrera, Brian Huey, Giuliano Cruz, Tim Gasser, James Wade, Tradd Salvo, Adam Bartonicek, William Benton, Alan O'Donnell, Mark Ormsby, Demetri Pananos, James Ahloy, Jon Berezowski, Robin Taylor, Thomas Wiecki, Chad Scherrer, Nathaniel Neitzke, Zwelithini Tunyiswa, Elea McDonnell Feit, Bertrand Wilden, James Thompson, Stephen Oates, Gian Luca Di Tanna, Jack Wells, Matthew Maldonado, Ian Costley, Ally Salim, Larry Gill, Joshua Duncan, Ian Moran, Paul Oreto, Colin Caprani, George Ho, Colin Carroll, Nathaniel Burbank, Michael Osthege, Rémi Louf, Clive Edelsten, Henri Wallen, Hugo Botha, Vinh Nguyen, Raul Maldonado, Marcin Elantkowski, Tim Radtke, Adam C. Smith, Will Kurt, Andrew Moskowitz, Hector Munoz, Marco Gorelli, Simon Kessell, Bradley Rode, Patrick Kelley, Rick Anderson, Casper de Bruin and Philippe Labonde.Visit https://www.patreon.com/learnbayesstats to unlock exclusive Bayesian swag ;)Links from the show:Chelsea's website: https://cmparlettpelleriti.github.io/index.htmlChelsea on Twitter: https://twitter.com/ChelseaParlettMichael Betancourt's sparsity case study: https://betanalpha.github.io/assets/case_studies/modeling_sparsity.htmlLBS #31 -- Bayesian Cognitive Modeling & Decision-Making, with Michael Lee: https://www.learnbayesstats.com/episode/31-bayesian-cognitive-modeling-michael-leeProjection predictive variable selection R package: https://mc-stan.org/projpred/SelectiveInference R package: https://cran.r-project.org/web/packages/selectiveInference/selectiveInference.pdfStatistical learning and selective inference: https://www.pnas.org/content/112/25/7629LBS #29 -- Model Assessment, Non-Parametric Models, with Aki Vehtari: https://www.learnbayesstats.com/episode/model-assessment-non-parametric-models-aki-vehtariLBS #35 -- The Past, Present & Future of BRMS, with Paul Bürkner: https://www.learnbayesstats.com/episode/35-past-present-future-brms-paul-burknerBRMS R Package: https://paul-buerkner.github.io/brms/Bayesian Item Response Modeling in R with BRMS and Stan: https://arxiv.org/pdf/1905.09501.pdfBAyesian Model-Building Interface (Bambi) in PythonBAyesian Model-Building Interface (Bambi) in Python: https://bambinos.github.io/bambi/main/index.htmlZero-one-inflated beta regression: https://twitter.com/SolomonKurz/status/1395056477459648521Ordinal Regression Models in Psychology: https://www.researchgate.net/publication/331335573_Ordinal_Regression_Models_in_Psychology_A_TutorialLBS #38 -- How to Become a Good Bayesian (& Rap Artist), with Baba Brinkman: https://www.learnbayesstats.com/episode/38-how-to-become-good-bayesian-rap-artist-baba-brinkman

Aug 10, 2021 • 1h 9min
#45 Biostats & Clinical Trial Design, with Frank Harrell
As a podcaster, I discovered that there are guests for which the hardest is to know when to stop the conversation. They could talk for hours and that would make for at least 10 fantastic episodes. Frank Harrell is one of those guests. To me, our conversation was both fascinating — thanks to Frank’s expertise and the width and depth of topics we touched on — and frustrating — I still had a gazillion questions for him!But rest assured, we talked about intent to treat and randomization, proportional odds, clinical trial design, bio stats and covid19, and even which mistakes you should do to learn Bayes stats — yes, you heard right, which mistakes. Anyway, I can’t tell you everything here — you’ll just have to listen to the episode!A long time Bayesian, Frank is a Professor of Biostatistics in the School of Medicine at Vanderbilt University. His numerous research interests include predictive models and model validation, Bayesian clinical trial design and Bayesian models, drug development, and clinical research.He holds a PhD in biostatistics from the University of North Carolina, and did his Bachelor in mathematics at the University of Alabama in Birmingham.Our theme music is « Good Bayesian », by Baba Brinkman (feat MC Lars and Mega Ran). Check out his awesome work at https://bababrinkman.com/ !Thank you to my Patrons for making this episode possible!Yusuke Saito, Avi Bryant, Ero Carrera, Brian Huey, Giuliano Cruz, Tim Gasser, James Wade, Tradd Salvo, Adam Bartonicek, William Benton, Alan O'Donnell, Mark Ormsby, Demetri Pananos, James Ahloy, Jon Berezowski, Robin Taylor, Thomas Wiecki, Chad Scherrer, Nathaniel Neitzke, Zwelithini Tunyiswa, Elea McDonnell Feit, Bertrand Wilden, James Thompson, Stephen Oates, Gian Luca Di Tanna, Jack Wells, Matthew Maldonado, Ian Costley, Ally Salim, Larry Gill, Joshua Duncan, Ian Moran, Paul Oreto, Colin Caprani, George Ho, Colin Carroll, Nathaniel Burbank, Michael Osthege, Rémi Louf, Clive Edelsten, Henri Wallen, Hugo Botha, Vinh Nguyen, Raul Maldonado, Marcin Elantkowski, Tim Radtke, Adam C. Smith, Will Kurt, Andrew Moskowitz, Hector Munoz, Marco Gorelli, Simon Kessell, Bradley Rode, Patrick Kelley, Rick Anderson, Casper de Bruin and Philippe Labonde.Visit https://www.patreon.com/learnbayesstats to unlock exclusive Bayesian swag ;)Links from the show:Frank's website and courses: https://hbiostat.org/Frank's blog: https://www.fharrell.com/Frank on Twitter: https://twitter.com/f2harrellCOVID-19 Randomized Clinical Trial Design: https://hbiostat.org/proj/covid19/Frank on GitHub: https://github.com/harrelfeRegression Modeling Strategies repository: https://github.com/harrelfe/rmsBiostatistics for Biomedical Research repository: https://github.com/harrelfe/bbrBayesian Approaches to Randomized Trials, Spiegelhalter et al.: http://hbiostat.org/papers/Bayes/spi94bay.pdfStatistical Rethinking, Richard McElreath: http://xcelab.net/rm/statistical-rethinking/LBS #20, Regression and Other Stories, with Andrew Gelman, Jennifer Hill & Aki Vehtari: https://www.learnbayesstats.com/episode/20-regression-and-other-stories-with-andrew-gelman-jennifer-hill-aki-vehtariDavid Spiegelhalter, The Art of Statistics -- Learning from Data: https://www.amazon.fr/Art-Statistics-Learning-Data/dp/0241398630Confidence intervals vs. Bayesian intervals, E.T. Jaynes: https://bayes.wustl.edu/etj/articles/confidence.pdf

Jul 22, 2021 • 1h 15min
#44 Building Bayesian Models at scale, with Rémi Louf
Episode sponsored by Paperpile: paperpile.comGet 20% off until December 31st with promo code GOODBAYESIAN21Bonjour my dear Bayesians! Yes, it was bound to happen one day — and this day has finally come. Here is the first ever 100% French speaking ‘Learn Bayes Stats’ episode! Who is to blame, you ask? Well, who better than Rémi Louf?Rémi currently works as a senior data scientist at Ampersand, a big media marketing company in the US. He is the author and maintainer of several open source libraries, including MCX and BlackJAX. He holds a PhD in statistical Physics, a Masters in physics from the Ecole Normale Supérieure and a Masters in Philosophy from Oxford University.I think I know what you’re wondering: how the hell do you go from physics to philosophy to Bayesian stats?? Glad you asked, as it was my first question to Rémi! He’ll also tell us why he created MXC and BlackJax, what his main challenges are when working on open-source projects, and what the future of PPLs looks like to him.Our theme music is « Good Bayesian », by Baba Brinkman (feat MC Lars and Mega Ran). Check out his awesome work at https://bababrinkman.com/ !Thank you to my Patrons for making this episode possible!Yusuke Saito, Avi Bryant, Ero Carrera, Brian Huey, Giuliano Cruz, Tim Gasser, James Wade, Tradd Salvo, Adam Bartonicek, William Benton, Alan O'Donnell, Mark Ormsby, Demetri Pananos, James Ahloy, Jon Berezowski, Robin Taylor, Thomas Wiecki, Chad Scherrer, Nathaniel Neitzke, Zwelithini Tunyiswa, Elea McDonnell Feit, Bertrand Wilden, James Thompson, Stephen Oates, Gian Luca Di Tanna, Jack Wells, Matthew Maldonado, Ian Costley, Ally Salim, Larry Gill, Joshua Duncan, Ian Moran, Paul Oreto, Colin Caprani, George Ho, Colin Carroll, Nathaniel Burbank, Michael Osthege, Rémi Louf, Clive Edelsten, Henri Wallen, Hugo Botha, Vinh Nguyen, Raul Maldonado, Marcin Elantkowski, Tim Radtke, Adam C. Smith, Will Kurt, Andrew Moskowitz, Hector Munoz, Marco Gorelli, Simon Kessell, Bradley Rode, Patrick Kelley, Rick Anderson, Casper de Bruin and Philippe Labonde.Visit https://www.patreon.com/learnbayesstats to unlock exclusive Bayesian swag ;)Links from the show:Rémi on GitHub: https://github.com/rloufRémi on Twitter: https://twitter.com/remiloufRémi's website: https://rlouf.github.io/BlackJAX -- Fast & modular sampling library: https://github.com/blackjax-devs/blackjaxMCX -- Probabilistic programs on CPU & GPU, powered by JAX: https://github.com/rlouf/mcxaeppl, Tools for a PPL in Aesara: https://github.com/aesara-devs/aepplFrench Presidents' popularity dashboard: https://www.pollsposition.com/popularityHow to model presidential approval (in French): https://anchor.fm/pollspolitics/episodes/10-Comment-Modliser-la-Popularit-e121jh2LBS #23, Bayesian Stats in Business & Marketing, with Elea McDonnel Feit: https://www.learnbayesstats.com/episode/23-bayesian-stats-in-business-and-marketing-analytics-with-elea-mcdonnel-feitLBS #30, Symbolic Computation & Dynamic Linear Models, with Brandon Willard: https://www.learnbayesstats.com/episode/symbolic-computation-dynamic-linear-models-brandon-willard

Jul 8, 2021 • 1h 22min
#43 Modeling Covid19, with Michael Osthege & Thomas Vladeck
Episode sponsored by Paperpile: paperpile.comGet 20% off until December 31st with promo code GOODBAYESIAN21I don’t know if you’ve heard, but there is a virus that took over most of the world in the past year? I haven’t dedicated any episode to Covid yet. First because research was moving a lot — and fast. And second because modeling Covid is very, very hard.But we know more about it now, so I thought it was a good time to pause and ponder — how does the virus circulate? How can we model it and, ultimately, defeat it? What are the challenges in doing so?To talk about that, I had the chance to host Michael Osthege and Thomas Vladeck, who both were part of the team who developed the Rt-live model, a Bayesian model to infer the reproductive rate of Covid19 in the general population. As you’ll hear, modeling the evolution of this virus is challenging, fascinating, and a perfect fit for Bayesian modeling! It truly is a wonderful example of Bayesian generative modeling.Tom is the Managing Director of Gradient Metrics, a quantitative market research firm, and a Co-Founder of Recast, a media mix model for modern brands.Michael is a PhD student in laboratory automation and bioprocess optimization at the Forschungszentrum Jülich in Germany, and a fellow PyMC core-developer. As he works a lot on the coming brand new version 4, we’ll take this opportunity to talk about the current developments and where the project is headed.Our theme music is « Good Bayesian », by Baba Brinkman (feat MC Lars and Mega Ran). Check out his awesome work at https://bababrinkman.com/ !Thank you to my Patrons for making this episode possible!Yusuke Saito, Avi Bryant, Ero Carrera, Brian Huey, Giuliano Cruz, Tim Gasser, James Wade, Tradd Salvo, Adam Bartonicek, William Benton, Alan O'Donnell, Mark Ormsby, Demetri Pananos, James Ahloy, Jon Berezowski, Robin Taylor, Thomas Wiecki, Chad Scherrer, Nathaniel Neitzke, Zwelithini Tunyiswa, Elea McDonnell Feit, Bertrand Wilden, James Thompson, Stephen Oates, Gian Luca Di Tanna, Jack Wells, Matthew Maldonado, Ian Costley, Ally Salim, Larry Gill, Joshua Duncan, Ian Moran, Paul Oreto, Colin Caprani, George Ho, Colin Carroll, Nathaniel Burbank, Michael Osthege, Rémi Louf, Clive Edelsten, Henri Wallen, Jonathan Sedar, Hugo Botha, Vinh Nguyen, Raul Maldonado, Marcin Elantkowski, Tim Radtke, Adam C. Smith, Will Kurt, Andrew Moskowitz, Hector Munoz, Marco Gorelli, Simon Kessell, Bradley Rode and Patrick Kelley.Visit https://www.patreon.com/learnbayesstats to unlock exclusive Bayesian swag ;)Links from the show:Tom on Twitter: https://twitter.com/tvladeckTom's newsletter: https://tvladeck.substack.com/Michael on Twitter: https://twitter.com/theCakeMichael on GitHub: https://github.com/michaelosthegeRt Live dashboard: https://rtlive.de/global.htmlRt Live model tutorial: https://github.com/rtcovidlive/rtlive-global/blob/master/notebooks/Tutorial_model.ipynbRt Live model code: https://github.com/rtcovidlive/rtlive-globalEstimating Rt: https://staff.math.su.se/hoehle/blog/2020/04/15/effectiveR0.htmlGreat resource on terminology: https://royalsociety.org/-/media/policy/projects/set-c/set-covid-19-R-estimates.pdf?la=en-GB&hash=FDFFC11968E5D247D8FF641930680BD6Using Hierarchical Multinomial Regression to Predict Elections in Paris districts: https://www.youtube.com/watch?v=EYdIzSYwbSwLBS #34, Multilevel Regression, Post-stratification & Missing Data, with Lauren Kennedy: https://www.learnbayesstats.com/episode/34-multilevel-regression-post-stratification-missing-data-lauren-kennedymrmp - Multilevel Regression and Marginal Poststratification: https://rdrr.io/github/jwyatt85/MRmP/man/mrmp.htmlAutomating daily runs for rt.live’s COVID-19 data using Airflow & ECS: https://medium.com/@mikekrieger/automating-daily-runs-for-rt-lives-covid-19-data-dcda26ed2e2eLBS #23, Bayesian Stats in Business and Marketing Analytics, with Elea McDonnel Feit: https://www.learnbayesstats.com/episode/23-bayesian-stats-in-business-and-marketing-analytics-with-elea-mcdonnel-feit

4 snips
Jun 24, 2021 • 1h 6min
#42 How to Teach and Learn Bayesian Stats, with Mine Dogucu
Episode sponsored by Paperpile: paperpile.comGet 20% off until December 31st with promo code GOODBAYESIAN21We often talk about applying Bayesian statistics on this podcast. But how do we teach them? What’s the best way to introduce them from a young age and make sure the skills students learn in the stats class are transferable?Well, lucky us, Mine Dogucu’s research tackles precisely those topics!An Assistant Professor of Teaching in the Department of Statistics at University of California Irvine, Mine is both an educator with an interest in statistics, and an applied statistician with experience in educational research.Her work focuses on modern pedagogical approaches in the statistics curriculum, making data science education more accessible. In particular, she teaches an undergraduate Bayesian course, and is the coauthor of the upcoming book Bayes Rules! An Introduction to Bayesian Modeling with R.In other words, Mine is not only interested in teaching, but also in how best to teach statistics – how to engage students in remote classes, how to get to know them, how to best record and edit remote courses, etc. She writes about these topics on her blog, DataPedagogy.com.She also works on accessibility and inclusion, as well as a study that investigates how popular Bayesian courses are at the undergraduate level in the US — that should be fun to talk about!Mine did her Master’s at Bogazici University in Istanbul, Turkey, and then her PhD in Quantitative Research, Evaluation, and Measurement at Ohio State University.Our theme music is « Good Bayesian », by Baba Brinkman (feat MC Lars and Mega Ran). Check out his awesome work at https://bababrinkman.com/ !Thank you to my Patrons for making this episode possible!Yusuke Saito, Avi Bryant, Ero Carrera, Brian Huey, Giuliano Cruz, Tim Gasser, James Wade, Tradd Salvo, Adam Bartonicek, William Benton, Alan O'Donnell, Mark Ormsby, Demetri Pananos, James Ahloy, Jon Berezowski, Robin Taylor, Thomas Wiecki, Chad Scherrer, Nathaniel Neitzke, Zwelithini Tunyiswa, Elea McDonnell Feit, Bertrand Wilden, James Thompson, Stephen Oates, Gian Luca Di Tanna, Jack Wells, Matthew Maldonado, Ian Costley, Ally Salim, Larry Gill, Joshua Duncan, Ian Moran, Paul Oreto, Colin Caprani, George Ho, Colin Carroll, Nathaniel Burbank, Michael Osthege, Rémi Louf, Clive Edelsten, Henri Wallen, Jonathan Sedar, Hugo Botha, Vinh Nguyen, Raul Maldonado, Marcin Elantkowski, Tim Radtke, Adam C. Smith, Will Kurt, Andrew Moskowitz, John Johnson, Hector Munoz, Marco Gorelli, Simon Kessell, Bradley Rode and Patrick Kelley.Visit https://www.patreon.com/learnbayesstats to unlock exclusive Bayesian swag ;)Links from the show:Mine's website: https://mdogucu.ics.uci.edu/index.htmlMine's blog: https://www.datapedagogy.com/Mine on Twitter: https://twitter.com/MineDogucuMine on GitHub: https://github.com/mdogucuBayes Rules! An Introduction to Bayesian Modeling with R: https://www.bayesrulesbook.com/R package for Supplemental Materials for the Bayes Rules! Book: https://github.com/bayes-rules/bayesrulesStats 115 - Introduction to Bayesian Data Analysis: https://www.stats115.com/Undergraduate Bayesian Education Network: https://undergrad-bayes.netlify.app/network.htmlWorkshop "Teaching Bayesian Statistics at the Undergraduate Level": https://www.causeweb.org/cause/uscots/uscots21/workshop/4

Jun 14, 2021 • 1h 4min
#41 Thinking Bayes, with Allen Downey
Let’s think Bayes, shall we? And who better to do that than the author of the well known book, Think Bayes — Allen Downey himself! Since the second edition was just released, the timing couldn’t be better!Allen is a professor at Olin College and the author of books related to software and data science, including Think Python, Think Bayes, and Think Complexity. His blog, Probably Overthinking It, features articles on Bayesian probability and statistics. He holds a Ph.D. from U.C. Berkeley, and bachelors and masters degrees from MIT.In this special episode, Allen and I talked about his background, how he came to the stats and teaching worlds, and why he wanted to write this book in the first place. He’ll tell us who this book is written for, what’s new in the second edition, and which mistakes his students most commonly make when starting to learn Bayesian stats. We also talked about some types of models, their usefulness and their weaknesses, but I’ll let you discover that.Now for another good news: 5 Patrons of the show will get Think Bayes for free! To qualify, you just need to go the form I linked to in the 'Learn Bayes Stats' Slack channel or the Patreon page and enter your email address. That’s it. After a week or so, Allen and I will choose 5 winners at random, who will receive the book for free!If you’re not a Patron yet, make sure to check out patreon.com/learnbayesstats if you don’t want to miss out on these goodies!And even if you’re not a Patron, I love you dear listeners, so you all get a discount when you go buy the book at https://www.learnbayesstats.com/buy-think-bayes (unfortunately, this only applies for purchases in the US and Canada).Our theme music is « Good Bayesian », by Baba Brinkman (feat MC Lars and Mega Ran). Check out his awesome work at https://bababrinkman.com/ !Thank you to my Patrons for making this episode possible!Yusuke Saito, Avi Bryant, Ero Carrera, Brian Huey, Giuliano Cruz, Tim Gasser, James Wade, Tradd Salvo, Adam Bartonicek, William Benton, Alan O'Donnell, Mark Ormsby, Demetri Pananos, James Ahloy, Jon Berezowski, Robin Taylor, Thomas Wiecki, Chad Scherrer, Nathaniel Neitzke, Zwelithini Tunyiswa, Elea McDonnell Feit, Bertrand Wilden, James Thompson, Stephen Oates, Gian Luca Di Tanna, Jack Wells, Matthew Maldonado, Ian Costley, Ally Salim, Larry Gill, Joshua Duncan, Ian Moran, Paul Oreto, Colin Caprani, George Ho, Colin Carroll, Nathaniel Burbank, Michael Osthege, Rémi Louf, Clive Edelsten, Henri Wallen, Jonathan Sedar, Hugo Botha, Vinh Nguyen, Raul Maldonado, Marcin Elantkowski, Tim Radtke, Adam C. Smith, Will Kurt, Andrew Moskowitz, John Johnson and Hector Munoz.Visit https://www.patreon.com/learnbayesstats to unlock exclusive Bayesian swag ;)Links from the show:Give LBS a 5-star rating on Podchaser: https://www.podchaser.com/learnbayesstatsBuy Think Bayes at a 40% discount with the code LBS40 (expires on July 31; only applies for purchases in the US and Canada): https://www.learnbayesstats.com/buy-think-bayesThink Bayes 2 online: http://allendowney.github.io/ThinkBayes2/index.htmlAllen's blog: https://www.allendowney.com/blog/Allen on Twitter: https://twitter.com/allendowneyAllen on GitHub: https://github.com/AllenDowneyInformation theory, inference and learning algorithms, David MacKay: https://www.inference.org.uk/itila/Statistical Rethinking, Richard McElreath: http://xcelab.net/rm/statistical-rethinking/Doing Bayesian Data Analysis, John Kruschke: https://sites.google.com/site/doingbayesiandataanalysis/homeProbabilistic Programming & Bayesian Methods for Hackers, Cam Davidson-Pilon: http://camdavidsonpilon.github.io/Probabilistic-Programming-and-Bayesian-Methods-for-Hackers/LBS #14, Hidden Markov Models & Statistical Ecology, with Vianey Leos-Barajas: https://www.learnbayesstats.com/episode/14-hidden-markov-models-statistical-ecology-with-vianey-leos-barajasThe Prosecutor's fallacy: https://en.wikipedia.org/wiki/Prosecutor%27s_fallacyConfidence intervals vs. Bayesian intervals, E.T. Jaynes: https://bayes.wustl.edu/etj/articles/confidence.pdfSuperforecasting, The Art and Science of Prediction, Philip Tetlock: https://en.wikipedia.org/wiki/Superforecasting:_The_Art_and_Science_of_Prediction

May 28, 2021 • 1h 6min
#40 Bayesian Stats for the Speech & Language Sciences, with Allison Hilger and Timo Roettger
We all know about these accidental discoveries — penicillin, the heating power of microwaves, or the famous (and delicious) tarte tatin. I don’t know why, but I just love serendipity. And, as you’ll hear, this episode is deliciously full of it…Thanks to Allison Hilger and Timo Roettger, we’ll discover the world of linguistics, how Bayesian stats are helpful there, and how Paul Bürkner’s BRMS package has been instrumental in this field. To my surprise — and perhaps yours — the speech and language sciences are pretty quantitative and computational!As she recently discovered Bayesian stats, Allison will also tell us about the challenges she’s faced from advisors and reviewers during her PhD at Northwestern University, and the advice she’d have for people in the same situation.Allison is now an Assistant Professor at the University of Colorado Boulder. The overall goal in her research is to improve our understanding of motor speech control processes, in order to inform effective speech therapy treatments for improved speech naturalness and intelligibility. Allison also worked clinically as a speech-language pathologist in Chicago for a year. As a new Colorado resident, her new hobbies include hiking, skiing, and biking — and then reading or going to dog parks when she’s to tired.Holding a PhD in linguistics from the University of Cologne, Germany, Timo is an Associate Professor for linguistics at the University of Oslo, Norway. Timo tries to understand how people communicate their intentions using speech – how are speech signals retrieved; how do people learn and generalize? Timo is also committed to improving methodologies across the language sciences in light of the replication crisis, with a strong emphasis on open science.Most importantly, Timo loves hiking, watching movies or, even better, watching people play video games!Our theme music is « Good Bayesian », by Baba Brinkman (feat MC Lars and Mega Ran). Check out his awesome work at https://bababrinkman.com/ !Thank you to my Patrons for making this episode possible!Yusuke Saito, Avi Bryant, Ero Carrera, Brian Huey, Giuliano Cruz, Tim Gasser, James Wade, Tradd Salvo, Adam Bartonicek, William Benton, Alan O'Donnell, Mark Ormsby, Demetri Pananos, James Ahloy, Jon Berezowski, Robin Taylor, Thomas Wiecki, Chad Scherrer, Nathaniel Neitzke, Zwelithini Tunyiswa, Elea McDonnell Feit, Bertrand Wilden, James Thompson, Stephen Oates, Gian Luca Di Tanna, Jack Wells, Matthew Maldonado, Ian Costley, Ally Salim, Larry Gill, Joshua Duncan, Ian Moran, Paul Oreto, Colin Caprani, George Ho, Colin Carroll, Nathaniel Burbank, Michael Osthege, Rémi Louf, Clive Edelsten, Henri Wallen, Jonathan Sedar, Hugo Botha, Vinh Nguyen, Raul Maldonado, Marcin Elantkowski, Tim Radtke, Adam C. Smith, Will Kurt and Andrew Moskowitz.Visit https://www.patreon.com/learnbayesstats to unlock exclusive Bayesian swag ;)Links from the show:Allison's website: https://allisonhilger.com/Allison on Twitter: https://twitter.com/drahilgerAllison's motor speech lab: https://www.colorado.edu/lab/motor-speech/Timo's website: https://www.simplpoints.com/Timo on Twitter: https://twitter.com/TimoRoettgerBayesian regression modeling (for factorial designs) -- A tutorial: https://psyarxiv.com/cdxv3An Introduction to Bayesian Multilevel Models Using brms -- A Case Study of Gender Effects on Vowel Variability in Standard Indonesian: https://biblio.ugent.be/publication/8624552/file/8624553.pdfLongitudinal Growth in Intelligibility of Connected Speech From 2 to 8 Years in Children With Cerebral Palsy -- A Novel Bayesian Approach: https://pubs.asha.org/doi/10.1044/2020_JSLHR-20-00181LBS #35 The Past, Present & Future of BRMS, with Paul Bürkner: https://www.learnbayesstats.com/episode/35-past-present-future-brms-paul-burknerLBS #16 Bayesian Statistics the Fun Way, with Will Kurt: https://www.learnbayesstats.com/episode/16-bayesian-statistics-the-fun-way-with-will-kurtWill Kurt's Bayesian Statistics The Fun Way: https://nostarch.com/learnbayesLBS #20 Regression and Other Stories, with Andrew Gelman, Jennifer Hill & Aki Vehtari: https://www.learnbayesstats.com/episode/20-regression-and-other-stories-with-andrew-gelman-jennifer-hill-aki-vehtariRegression and Other Stories examples: https://avehtari.github.io/ROS-Examples/