
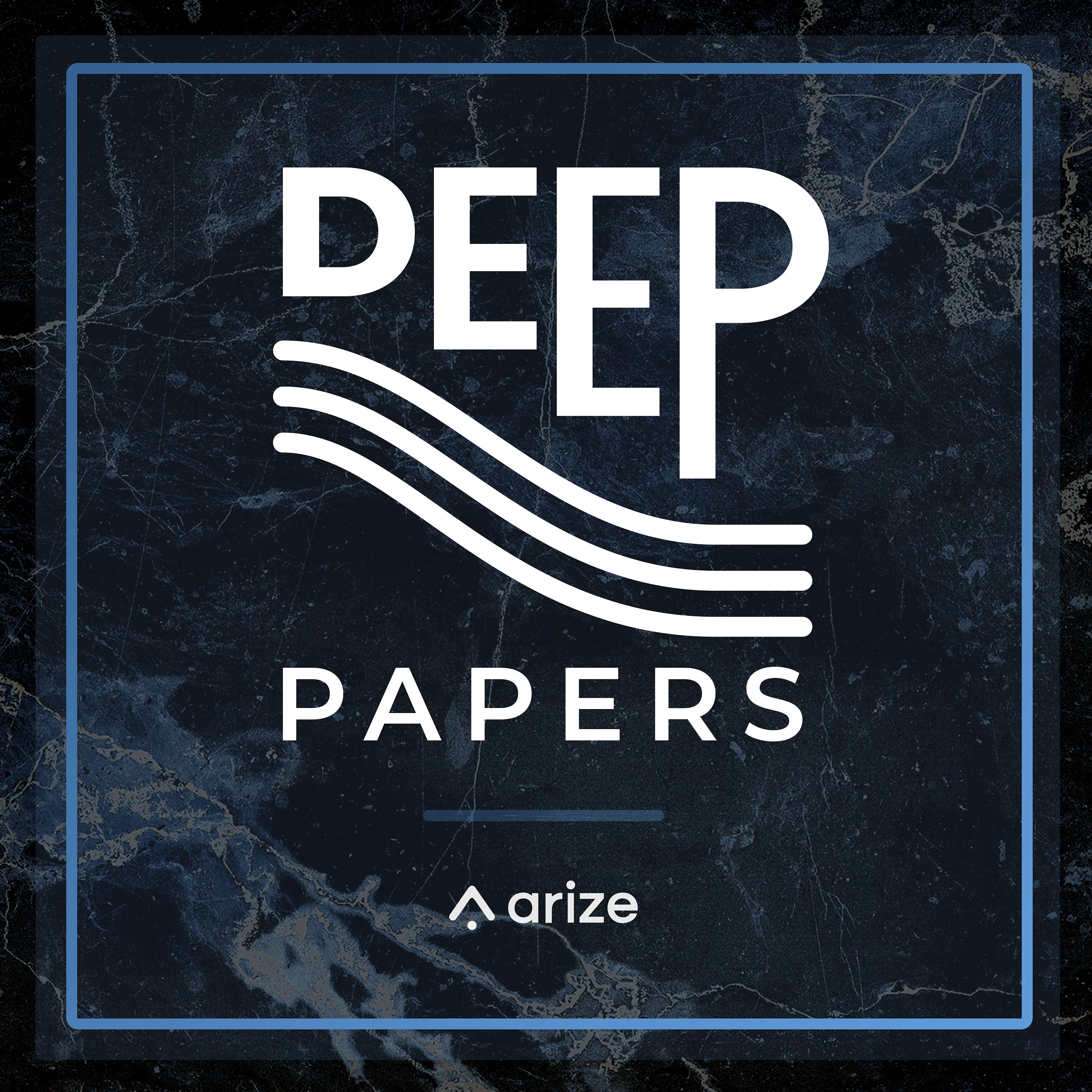
Deep Papers
Arize AI
Deep Papers is a podcast series featuring deep dives on today’s most important AI papers and research. Hosted by Arize AI founders and engineers, each episode profiles the people and techniques behind cutting-edge breakthroughs in machine learning.
Episodes
Mentioned books

Sep 27, 2024 • 42min
Exploring OpenAI's o1-preview and o1-mini
OpenAI recently released its o1-preview, which they claim outperforms GPT-4o on a number of benchmarks. These models are designed to think more before answering and handle complex tasks better than their other models, especially science and math questions. We take a closer look at their latest crop of o1 models, and we also highlight some research our team did to see how they stack up against Claude Sonnet 3.5--using a real world use case. Read it on our blog: https://arize.com/blog/exploring-openai-o1-preview-and-o1-miniLearn more about AI observability and evaluation, join the Arize AI Slack community or get the latest on LinkedIn and X.

Sep 19, 2024 • 27min
Breaking Down Reflection Tuning: Enhancing LLM Performance with Self-Learning
A recent announcement on X boasted a tuned model with pretty outstanding performance, and claimed these results were achieved through Reflection Tuning. However, people were unable to reproduce the results. We dive into some recent drama in the AI community as a jumping off point for a discussion about Reflection 70B.In 2023, there was a paper written about Reflection Tuning that this new model (Reflection 70B) draws concepts from. Reflection tuning is an optimization technique where models learn to improve their decision-making processes by “reflecting” on past actions or predictions. This method enables models to iteratively refine their performance by analyzing mistakes and successes, thus improving both accuracy and adaptability over time. By incorporating a feedback loop, reflection tuning can address model weaknesses more dynamically, helping AI systems become more robust in real-world applications where uncertainty or changing environments are prevalent.Dat Ngo (AI Solutions Architect at Arize), talks to Rohan Pandey (Founding Engineer at Reworkd) about Reflection 70B, Reflection Tuning, the recent drama, and the importance of double checking your research.Learn more about AI observability and evaluation, join the Arize AI Slack community or get the latest on LinkedIn and X.

Sep 11, 2024 • 43min
Composable Interventions for Language Models
This week, we're excited to be joined by Kyle O'Brien, Applied Scientist at Microsoft, to discuss his most recent paper, Composable Interventions for Language Models. Kyle and his team present a new framework, composable interventions, that allows for the study of multiple interventions applied sequentially to the same language model. The discussion will cover their key findings from extensive experiments, revealing how different interventions—such as knowledge editing, model compression, and machine unlearning—interact with each other.Read it on the blog: https://arize.com/blog/composable-interventions-for-language-models/Learn more about AI observability and evaluation, join the Arize AI Slack community or get the latest on LinkedIn and X.

Aug 16, 2024 • 39min
Judging the Judges: Evaluating Alignment and Vulnerabilities in LLMs-as-Judges
This week’s paper presents a comprehensive study of the performance of various LLMs acting as judges. The researchers leverage TriviaQA as a benchmark for assessing objective knowledge reasoning of LLMs and evaluate them alongside human annotations which they find to have a high inter-annotator agreement. The study includes nine judge models and nine exam-taker models – both base and instruction-tuned. They assess the judge models’ alignment across different model sizes, families, and judge prompts to answer questions about the strengths and weaknesses of this paradigm, and what potential biases it may hold.Read it on the blog: https://arize.com/blog/judging-the-judges-llm-as-a-judge/Learn more about AI observability and evaluation, join the Arize AI Slack community or get the latest on LinkedIn and X.

Aug 6, 2024 • 45min
Breaking Down Meta's Llama 3 Herd of Models
Meta just released Llama 3.1 405B–according to them, it’s “the first openly available model that rivals the top AI models when it comes to state-of-the-art capabilities in general knowledge, steerability, math, tool use, and multilingual translation.” Will the latest Llama herd ignite new applications and modeling paradigms like synthetic data generation? Will it enable the improvement and training of smaller models, as well as model distillation? Meta thinks so. We’ll take a look at what they did here, talk about open source, and decide if we want to believe the hype.Read it on the blog: https://arize.com/blog/breaking-down-meta-llama-3/Learn more about AI observability and evaluation, join the Arize AI Slack community or get the latest on LinkedIn and X.

Jul 23, 2024 • 34min
DSPy Assertions: Computational Constraints for Self-Refining Language Model Pipelines
Chaining language model (LM) calls as composable modules is fueling a new way of programming, but ensuring LMs adhere to important constraints requires heuristic “prompt engineering.” The paper this week introduces LM Assertions, a programming construct for expressing computational constraints that LMs should satisfy. The researchers integrated their constructs into the recent DSPy programming model for LMs and present new strategies that allow DSPy to compile programs with LM Assertions into more reliable and accurate systems. They also propose strategies to use assertions at inference time for automatic self-refinement with LMs. They reported on four diverse case studies for text generation and found that LM Assertions improve not only compliance with imposed rules but also downstream task performance, passing constraints up to 164% more often and generating up to 37% more higher-quality responses.We discuss this paper with Cyrus Nouroozi, DSPY key contributor. Read it on the blog: https://arize.com/blog/dspy-assertions-computational-constraints/Learn more about AI observability and evaluation, join the Arize AI Slack community or get the latest on LinkedIn and X.

6 snips
Jun 28, 2024 • 44min
RAFT: Adapting Language Model to Domain Specific RAG
Sai Kolasani, a researcher at UC Berkeley’s RISE Lab and Arize AI Intern, discusses RAFT, a method to adapt language models for domain-specific question-answering. RAFT improves models' reasoning by training them to ignore distractor documents, enhancing performance in specialized domains like PubMed and HotpotQA. The podcast explores RAFT's chain-of-thought-style response, data curation, and optimizing performance in domain-specific tasks.

Jun 14, 2024 • 44min
LLM Interpretability and Sparse Autoencoders: Research from OpenAI and Anthropic
Delve into recent research on LLM interpretability with k-sparse autoencoders from OpenAI and sparse autoencoder scaling laws from Anthropic. Explore the implications for understanding neural activity and extracting interpretable features from language models.

May 30, 2024 • 48min
Trustworthy LLMs: A Survey and Guideline for Evaluating Large Language Models' Alignment
We break down the paper--Trustworthy LLMs: A Survey and Guideline for Evaluating Large Language Models' Alignment.Ensuring alignment (aka: making models behave in accordance with human intentions) has become a critical task before deploying LLMs in real-world applications. However, a major challenge faced by practitioners is the lack of clear guidance on evaluating whether LLM outputs align with social norms, values, and regulations. To address this issue, this paper presents a comprehensive survey of key dimensions that are crucial to consider when assessing LLM trustworthiness. The survey covers seven major categories of LLM trustworthiness: reliability, safety, fairness, resistance to misuse, explainability and reasoning, adherence to social norms, and robustness.The measurement results indicate that, in general, more aligned models tend to perform better in terms of overall trustworthiness. However, the effectiveness of alignment varies across the different trustworthiness categories considered. By shedding light on these key dimensions of LLM trustworthiness, this paper aims to provide valuable insights and guidance to practitioners in the field. Understanding and addressing these concerns will be crucial in achieving reliable and ethically sound deployment of LLMs in various applications.Read more about Trustworthy LLMs: A Survey and Guideline for Evaluating Large Language Models' AlignmentLearn more about AI observability and evaluation, join the Arize AI Slack community or get the latest on LinkedIn and X.

May 13, 2024 • 45min
Breaking Down EvalGen: Who Validates the Validators?
This podcast delves into the complexities of using Large Language Models for evaluation, highlighting the need for human validation in aligning LLM-generated evaluators with user preferences. Topics include developing criteria for acceptable LLM outputs, evaluating email responses, evolving evaluation criteria, template management, LLM validation, and the iterative process of building effective evaluation criteria.