
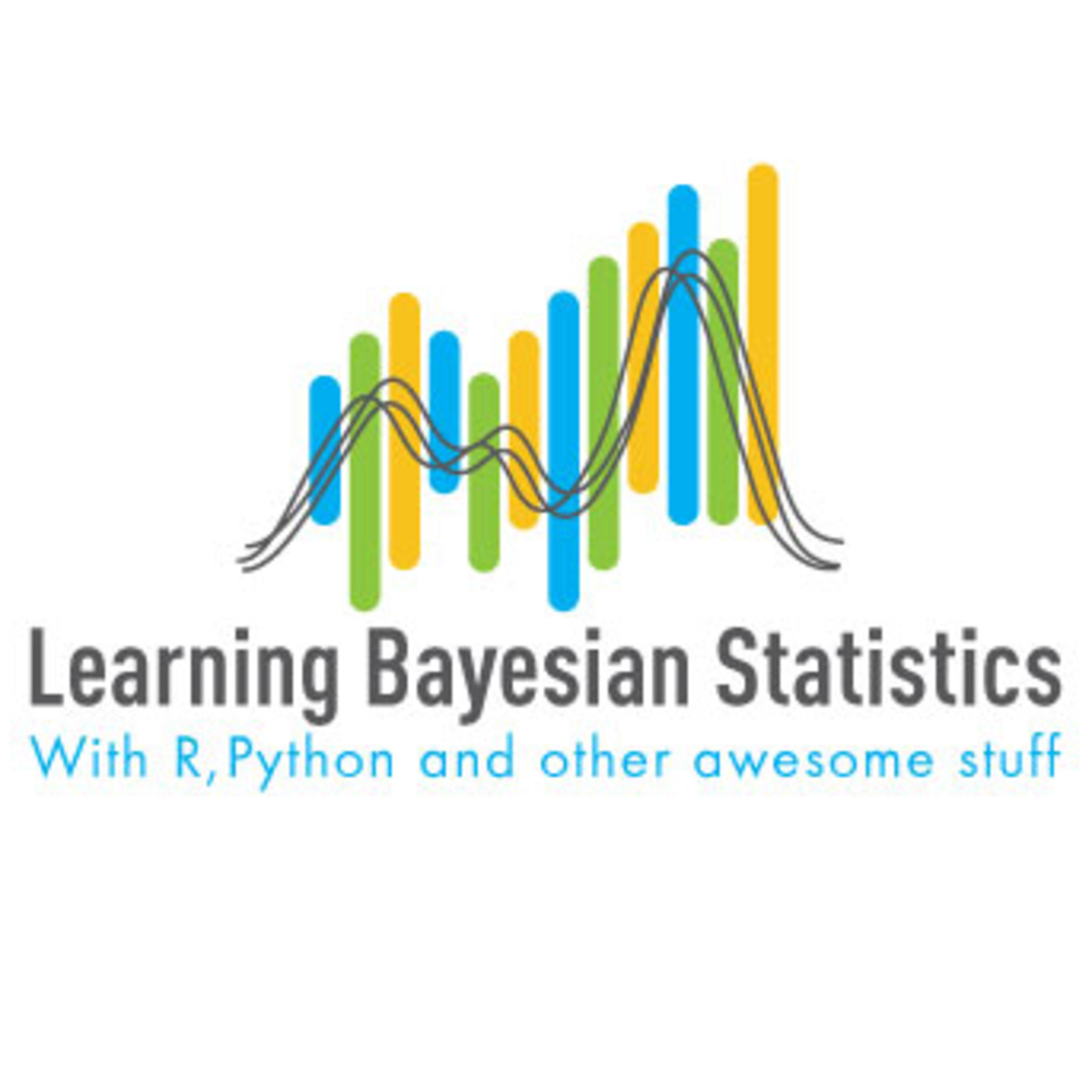
Learning Bayesian Statistics
Alexandre Andorra
Are you a researcher or data scientist / analyst / ninja? Do you want to learn Bayesian inference, stay up to date or simply want to understand what Bayesian inference is?
Then this podcast is for you! You'll hear from researchers and practitioners of all fields about how they use Bayesian statistics, and how in turn YOU can apply these methods in your modeling workflow.
When I started learning Bayesian methods, I really wished there were a podcast out there that could introduce me to the methods, the projects and the people who make all that possible.
So I created "Learning Bayesian Statistics", where you'll get to hear how Bayesian statistics are used to detect black matter in outer space, forecast elections or understand how diseases spread and can ultimately be stopped.
But this show is not only about successes -- it's also about failures, because that's how we learn best. So you'll often hear the guests talking about what *didn't* work in their projects, why, and how they overcame these challenges. Because, in the end, we're all lifelong learners!
My name is Alex Andorra by the way, and I live in Estonia. By day, I'm a data scientist and modeler at the https://www.pymc-labs.io/ (PyMC Labs) consultancy. By night, I don't (yet) fight crime, but I'm an open-source enthusiast and core contributor to the python packages https://docs.pymc.io/ (PyMC) and https://arviz-devs.github.io/arviz/ (ArviZ). I also love https://www.pollsposition.com/ (election forecasting) and, most importantly, Nutella. But I don't like talking about it – I prefer eating it.
So, whether you want to learn Bayesian statistics or hear about the latest libraries, books and applications, this podcast is for you -- just subscribe! You can also support the show and https://www.patreon.com/learnbayesstats (unlock exclusive Bayesian swag on Patreon)!
Then this podcast is for you! You'll hear from researchers and practitioners of all fields about how they use Bayesian statistics, and how in turn YOU can apply these methods in your modeling workflow.
When I started learning Bayesian methods, I really wished there were a podcast out there that could introduce me to the methods, the projects and the people who make all that possible.
So I created "Learning Bayesian Statistics", where you'll get to hear how Bayesian statistics are used to detect black matter in outer space, forecast elections or understand how diseases spread and can ultimately be stopped.
But this show is not only about successes -- it's also about failures, because that's how we learn best. So you'll often hear the guests talking about what *didn't* work in their projects, why, and how they overcame these challenges. Because, in the end, we're all lifelong learners!
My name is Alex Andorra by the way, and I live in Estonia. By day, I'm a data scientist and modeler at the https://www.pymc-labs.io/ (PyMC Labs) consultancy. By night, I don't (yet) fight crime, but I'm an open-source enthusiast and core contributor to the python packages https://docs.pymc.io/ (PyMC) and https://arviz-devs.github.io/arviz/ (ArviZ). I also love https://www.pollsposition.com/ (election forecasting) and, most importantly, Nutella. But I don't like talking about it – I prefer eating it.
So, whether you want to learn Bayesian statistics or hear about the latest libraries, books and applications, this podcast is for you -- just subscribe! You can also support the show and https://www.patreon.com/learnbayesstats (unlock exclusive Bayesian swag on Patreon)!
Episodes
Mentioned books

14 snips
Oct 15, 2024 • 1h 13min
#117 Unveiling the Power of Bayesian Experimental Design, with Desi Ivanova
Desi Ivanova, a distinguished research fellow in machine learning at Oxford, dives into the fascinating world of Bayesian experimental design. She discusses how optimal experiment design is crucial for effective data gathering and uncertainty reduction. Desi sheds light on computational challenges and innovations like amortized Bayesian inference. The conversation also touches on real-world applications of these designs in healthcare and technology and the promising future advancements with AI that could reshape research methodologies.

Oct 2, 2024 • 1h 33min
#116 Mastering Soccer Analytics, with Ravi Ramineni
Proudly sponsored by PyMC Labs, the Bayesian Consultancy. Book a call, or get in touch!My Intuitive Bayes Online Courses1:1 Mentorship with meOur theme music is « Good Bayesian », by Baba Brinkman (feat MC Lars and Mega Ran). Check out his awesome work!Visit our Patreon page to unlock exclusive Bayesian swag ;)Takeaways:Building an athlete management system and a scouting and recruitment platform are key goals in football analytics.The focus is on informing training decisions, preventing injuries, and making smart player signings.Avoiding false positives in player evaluations is crucial, and data analysis plays a significant role in making informed decisions.There are similarities between different football teams, and the sport has social and emotional aspects. Transitioning from on-premises SQL servers to cloud-based systems is a significant endeavor in football analytics.Analytics is a tool that aids the decision-making process and helps mitigate biases. The impact of analytics in soccer can be seen in the decline of long-range shots.Collaboration and trust between analysts and decision-makers are crucial for successful implementation of analytics.The limitations of available data in football analytics hinder the ability to directly measure decision-making on the field. Analyzing the impact of coaches in sports analytics is challenging due to the difficulty of separating their effect from other factors. Current data limitations make it hard to evaluate coaching performance accurately.Predictive metrics and modeling play a crucial role in soccer analytics, especially in predicting the career progression of young players.Improving tracking data and expanding its availability will be a significant focus in the future of soccer analytics.Chapters:00:00 Introduction to Ravi and His Role at Seattle Sounders 06:30 Building an Analytics Department15:00 The Impact of Analytics on Player Recruitment and Performance 28:00 Challenges and Innovations in Soccer Analytics 42:00 Player Health, Injury Prevention, and Training 55:00 The Evolution of Data-Driven Strategies01:10:00 Future of Analytics in SportsThank you to my Patrons for making this episode possible!Yusuke Saito, Avi Bryant, Ero Carrera, Giuliano Cruz, Tim Gasser, James Wade, Tradd Salvo, William Benton, James Ahloy, Robin Taylor,, Chad Scherrer, Zwelithini Tunyiswa, Bertrand Wilden, James Thompson, Stephen Oates, Gian Luca Di Tanna, Jack Wells, Matthew Maldonado, Ian Costley, Ally Salim, Larry Gill, Ian Moran, Paul Oreto, Colin Caprani, Colin Carroll, Nathaniel Burbank, Michael Osthege, Rémi Louf, Clive Edelsten, Henri Wallen, Hugo Botha, Vinh Nguyen, Marcin Elantkowski, Adam C. Smith, Will Kurt, Andrew Moskowitz, Hector Munoz, Marco Gorelli, Simon Kessell, Bradley Rode, Patrick Kelley, Rick Anderson, Casper de Bruin, Philippe Labonde, Michael Hankin, Cameron Smith, Tomáš Frýda, Ryan Wesslen, Andreas Netti, Riley King, Yoshiyuki Hamajima, Sven De Maeyer, Michael DeCrescenzo, Fergal M, Mason Yahr, Naoya Kanai, Steven Rowland, Aubrey Clayton, Jeannine Sue, Omri Har Shemesh, Scott Anthony Robson, Robert Yolken, Or Duek, Pavel Dusek, Paul Cox, Andreas Kröpelin, Raphaël R, Nicolas Rode, Gabriel Stechschulte, Arkady, Kurt TeKolste, Gergely Juhasz, Marcus Nölke, Maggi Mackintosh, Grant Pezzolesi, Avram Aelony, Joshua Meehl, Javier Sabio, Kristian Higgins, Alex Jones, Gregorio Aguilar, Matt Rosinski, Bart Trudeau, Luis Fonseca, Dante Gates, Matt Niccolls, Maksim Kuznecov, Michael Thomas, Luke Gorrie, Cory Kiser, Julio, Edvin Saveljev, Frederick Ayala, Jeffrey Powell, Gal Kampel, Adan Romero, Will Geary, Blake Walters, Jonathan Morgan, Francesco Madrisotti, Ivy Huang and Gary Clarke.Links from the show:LBS Sports Analytics playlist: https://www.youtube.com/playlist?list=PL7RjIaSLWh5kDiPVMUSyhvFaXL3NoXOe4Ravi on Linkedin: https://www.linkedin.com/in/ravi-ramineni-3798374/Ravi on Twitter: https://x.com/analyseFootyDecisions in Football - The Power of Compounding | StatsBomb Conference 2023: https://www.youtube.com/watch?v=D7CXtwDg9lMThe Signal and the Noise: https://www.amazon.com/Signal-Noise-Many-Predictions-Fail-but/dp/0143125087PreliZ – A tool-box for prior elicitation: https://preliz.readthedocs.io/en/latest/Ravi talking on Ted Knutson's podcast: https://open.spotify.com/episode/1exLBfyFf0d1dm2IaXkd2vMore about Ravi's work at the Seattle Sounders: https://www.trumedianetworks.com/expected-value-podcast/ravi-ramineniTranscriptThis is an automatic transcript and may therefore contain errors. Please get in touch if you're willing to correct them.

Sep 17, 2024 • 1h 40min
#115 Using Time Series to Estimate Uncertainty, with Nate Haines
Nate Haines, Head of Data Science Research at Ledger Investing and a PhD from Ohio State University, dives into the fascinating world of Bayesian statistics in insurance. He discusses how state space models can forecast loss ratios and the challenges of working with limited data. Haines introduces Bayesian model stacking for blending predictions, showcasing the BayesBlend Python package. He also explores the impact of external factors like economic conditions on insurance forecasting and the importance of simulation-based calibration in ensuring model integrity.

Sep 5, 2024 • 1h 2min
#114 From the Field to the Lab – A Journey in Baseball Science, with Jacob Buffa
Proudly sponsored by PyMC Labs, the Bayesian Consultancy. Book a call, or get in touch!My Intuitive Bayes Online Courses1:1 Mentorship with meOur theme music is « Good Bayesian », by Baba Brinkman (feat MC Lars and Mega Ran). Check out his awesome work!Visit our Patreon page to unlock exclusive Bayesian swag ;)Takeaways:Education and visual communication are key in helping athletes understand the impact of nutrition on performance.Bayesian statistics are used to analyze player performance and injury risk.Integrating diverse data sources is a challenge but can provide valuable insights.Understanding the specific needs and characteristics of athletes is crucial in conditioning and injury prevention. The application of Bayesian statistics in baseball science requires experts in Bayesian methods.Traditional statistical methods taught in sports science programs are limited.Communicating complex statistical concepts, such as Bayesian analysis, to coaches and players is crucial.Conveying uncertainties and limitations of the models is essential for effective utilization.Emerging trends in baseball science include the use of biomechanical information and computer vision algorithms.Improving player performance and injury prevention are key goals for the future of baseball science.Chapters:00:00 The Role of Nutrition and Conditioning05:46 Analyzing Player Performance and Managing Injury Risks12:13 Educating Athletes on Dietary Choices18:02 Emerging Trends in Baseball Science29:49 Hierarchical Models and Player Analysis36:03 Challenges of Working with Limited Data39:49 Effective Communication of Statistical Concepts47:59 Future Trends: Biomechanical Data Analysis and Computer Vision AlgorithmsThank you to my Patrons for making this episode possible!Yusuke Saito, Avi Bryant, Ero Carrera, Giuliano Cruz, Tim Gasser, James Wade, Tradd Salvo, William Benton, James Ahloy, Robin Taylor,, Chad Scherrer, Zwelithini Tunyiswa, Bertrand Wilden, James Thompson, Stephen Oates, Gian Luca Di Tanna, Jack Wells, Matthew Maldonado, Ian Costley, Ally Salim, Larry Gill, Ian Moran, Paul Oreto, Colin Caprani, Colin Carroll, Nathaniel Burbank, Michael Osthege, Rémi Louf, Clive Edelsten, Henri Wallen, Hugo Botha, Vinh Nguyen, Marcin Elantkowski, Adam C. Smith, Will Kurt, Andrew Moskowitz, Hector Munoz, Marco Gorelli, Simon Kessell, Bradley Rode, Patrick Kelley, Rick Anderson, Casper de Bruin, Philippe Labonde, Michael Hankin, Cameron Smith, Tomáš Frýda, Ryan Wesslen, Andreas Netti, Riley King, Yoshiyuki Hamajima, Sven De Maeyer, Michael DeCrescenzo, Fergal M, Mason Yahr, Naoya Kanai, Steven Rowland, Aubrey Clayton, Jeannine Sue, Omri Har Shemesh, Scott Anthony Robson, Robert Yolken, Or Duek, Pavel Dusek, Paul Cox, Andreas Kröpelin, Raphaël R, Nicolas Rode, Gabriel Stechschulte, Arkady, Kurt TeKolste, Gergely Juhasz, Marcus Nölke, Maggi Mackintosh, Grant Pezzolesi, Avram Aelony, Joshua Meehl, Javier Sabio, Kristian Higgins, Alex Jones, Gregorio Aguilar, Matt Rosinski, Bart Trudeau, Luis Fonseca, Dante Gates, Matt Niccolls, Maksim Kuznecov, Michael Thomas, Luke Gorrie, Cory Kiser, Julio, Edvin Saveljev, Frederick Ayala, Jeffrey Powell, Gal Kampel, Adan Romero, Will Geary, Blake Walters, Jonathan Morgan and Francesco Madrisotti.Links from the show:LBS Sports Analytics playlist: https://www.youtube.com/playlist?list=PL7RjIaSLWh5kDiPVMUSyhvFaXL3NoXOe4Jacob on Linkedin: https://www.linkedin.com/in/jacob-buffa-46bb7481/Jacob on Twitter: https://x.com/EBA_BuffaThe Book – Playing The Percentages In Baseball: https://www.amazon.com/Book-Playing-Percentages-Baseball/dp/1494260174Future Value – The Battle for Baseball's Soul and How Teams Will Find the Next Superstar: https://www.amazon.com/Future-Value-Battle-Baseballs-Superstar/dp/1629377678The MVP Machine – How Baseball's New Nonconformists Are Using Data to Build Better Players: https://www.amazon.com/MVP-Machine-Baseballs-Nonconformists-Players/dp/1541698940Transcript:This is an automatic transcript and may therefore contain errors. Please get in touch if you're willing to correct them.

7 snips
Aug 22, 2024 • 1h 31min
#113 A Deep Dive into Bayesian Stats, with Alex Andorra, ft. the Super Data Science Podcast
In this engaging discussion, John Crone, the host of the Super Data Science Podcast, dives deep into the world of Bayesian statistics. He emphasizes its power in addressing complex problems and managing uncertainty. The conversation covers practical applications across various fields, the importance of effective communication in presenting results, and the transformative role of tools like PyMC and Bambi for beginners. John shares valuable insights on the evolving nature of scientific knowledge and the relevance of podcasts for staying informed in the data science landscape.

Aug 7, 2024 • 1h 27min
#112 Advanced Bayesian Regression, with Tomi Capretto
Tomi Capretto, an innovative educator in Bayesian statistics, discusses his creative approach to teaching using an M&M classroom exercise to make complex concepts tangible. He shares insights on the real-world applications of Bayesian methods at PyMC Labs and the importance of community contributions to open-source software like Bambi. Tomi emphasizes the challenges of shifting students from frequentist to Bayesian thought and the future of user-friendly Bayesian tools, aiming to make statistical methods accessible to a broader audience.

Jul 24, 2024 • 1h 26min
#111 Nerdinsights from the Football Field, with Patrick Ward
Guest Patrick Ward discusses applying Bayesian statistics in sports analytics, challenges in communicating concepts to non-statistical decision-makers, predicting training load impact on athlete performance, analyzing player tracking data, and creating a comprehensive patient model in team sports.

5 snips
Jul 10, 2024 • 1h 12min
#110 Unpacking Bayesian Methods in AI with Sam Duffield
Expert Sam Duffield discusses leveraging Bayesian methods in AI, focusing on mini-batch techniques, approximate inference, thermodynamic computing, and the Posteriors python package. He simplifies complex concepts for non-expert audiences and highlights the role of temperature in Bayesian models, stochastic gradient MCMC, and uncertainty quantification for improved predictions.

4 snips
Jun 25, 2024 • 1h 11min
#109 Prior Sensitivity Analysis, Overfitting & Model Selection, with Sonja Winter
Sonja Winter, an Assistant Professor at the University of Missouri specializing in Bayesian methods for educational research, dives into intriguing discussions. She elaborates on the importance of prior sensitivity analysis for robust findings and how Bayesian techniques elegantly address missing data issues. The conversation also tackles the challenges of overfitting in structural equation modeling, emphasizing the need for caution in model selection. Winter's insights highlight the transformative potential of Bayesian approaches in navigating complex educational data.

Jun 14, 2024 • 1h 18min
#108 Modeling Sports & Extracting Player Values, with Paul Sabin
Proudly sponsored by PyMC Labs, the Bayesian Consultancy. Book a call, or get in touch!My Intuitive Bayes Online Courses1:1 Mentorship with meOur theme music is « Good Bayesian », by Baba Brinkman (feat MC Lars and Mega Ran). Check out his awesome work!Visit our Patreon page to unlock exclusive Bayesian swag ;)TakeawaysConvincing non-stats stakeholders in sports analytics can be challenging, but building trust and confirming their prior beliefs can help in gaining acceptance.Combining subjective beliefs with objective data in Bayesian analysis leads to more accurate forecasts.The availability of massive data sets has revolutionized sports analytics, allowing for more complex and accurate models.Sports analytics models should consider factors like rest, travel, and altitude to capture the full picture of team performance.The impact of budget on team performance in American sports and the use of plus-minus models in basketball and American football are important considerations in sports analytics.The future of sports analytics lies in making analysis more accessible and digestible for everyday fans.There is a need for more focus on estimating distributions and variance around estimates in sports analytics.AI tools can empower analysts to do their own analysis and make better decisions, but it's important to ensure they understand the assumptions and structure of the data.Measuring the value of certain positions, such as midfielders in soccer, is a challenging problem in sports analytics.Game theory plays a significant role in sports strategies, and optimal strategies can change over time as the game evolves.Chapters00:00 Introduction and Overview09:27 The Power of Bayesian Analysis in Sports Modeling16:28 The Revolution of Massive Data Sets in Sports Analytics31:03 The Impact of Budget in Sports Analytics39:35 Introduction to Sports Analytics52:22 Plus-Minus Models in American Football01:04:11 The Future of Sports AnalyticsThank you to my Patrons for making this episode possible!Yusuke Saito, Avi Bryant, Ero Carrera, Giuliano Cruz, Tim Gasser, James Wade, Tradd Salvo, William Benton, James Ahloy, Robin Taylor,, Chad Scherrer, Zwelithini Tunyiswa, Bertrand Wilden, James Thompson, Stephen Oates, Gian Luca Di Tanna, Jack Wells, Matthew Maldonado, Ian Costley, Ally Salim, Larry Gill, Ian Moran, Paul Oreto, Colin Caprani, Colin Carroll, Nathaniel Burbank, Michael Osthege, Rémi Louf, Clive Edelsten, Henri Wallen, Hugo Botha, Vinh Nguyen, Marcin Elantkowski, Adam C. Smith, Will Kurt, Andrew Moskowitz, Hector Munoz, Marco Gorelli, Simon Kessell, Bradley Rode, Patrick Kelley, Rick Anderson, Casper de Bruin, Philippe Labonde, Michael Hankin, Cameron Smith, Tomáš Frýda, Ryan Wesslen, Andreas Netti, Riley King, Yoshiyuki Hamajima, Sven De Maeyer, Michael DeCrescenzo, Fergal M, Mason Yahr, Naoya Kanai, Steven Rowland, Aubrey Clayton, Jeannine Sue, Omri Har Shemesh, Scott Anthony Robson, Robert Yolken, Or Duek, Pavel Dusek, Paul Cox, Andreas Kröpelin, Raphaël R, Nicolas Rode, Gabriel Stechschulte, Arkady, Kurt TeKolste, Gergely Juhasz, Marcus Nölke, Maggi Mackintosh, Grant Pezzolesi, Avram Aelony, Joshua Meehl, Javier Sabio, Kristian Higgins, Alex Jones, Gregorio Aguilar, Matt Rosinski, Bart Trudeau, Luis Fonseca, Dante Gates, Matt Niccolls, Maksim Kuznecov, Michael Thomas, Luke Gorrie, Cory Kiser, Julio, Edvin Saveljev, Frederick Ayala, Jeffrey Powell, Gal Kampel, Adan Romero, Will Geary, Blake Walters, Jonathan Morgan and Francesco Madrisotti.Links from the show:LBS Sports Analytics playlist: https://www.youtube.com/playlist?list=PL7RjIaSLWh5kDiPVMUSyhvFaXL3NoXOe4Paul’s website: https://sabinanalytics.com/Paul on GitHub: https://github.com/sabinanalytics Paul on Linkedin: https://www.linkedin.com/in/rpaulsabin/Paul on Twitter: https://twitter.com/SabinAnalyticsPaul on Google Scholar: https://scholar.google.com/citations?user=wAezxZ4AAAAJ&hl=enSoccer Power Ratings & Projections: https://sabinanalytics.com/ratings/soccer/Estimating player value in American football using plus–minus models: https://www.degruyter.com/document/doi/10.1515/jqas-2020-0033/htmlWorld Football R Package: https://github.com/JaseZiv/worldfootballRTranscriptThis is an automatic transcript and may therefore contain errors. Please get in touch if you're willing to correct them.