
Data Engineering Podcast
Reducing The Barrier To Entry For Building Stream Processing Applications With Decodable
Podcast summary created with Snipd AI
Quick takeaways
- Decodable is a stream processing platform that simplifies the complex workflows involved in stream processing and data distribution between various systems, making it easier for organizations to adopt stream processing and integrate it seamlessly into their data infrastructure.
- The stream processing layer and the data warehouse are complementary components in optimizing performance, data latency, and cost efficiency in data workflows.
- Decodable offers support for complex processing, state management, and sophisticated data enrichment within the stream processing layer, reducing latency and providing richer data for operational use cases and system integrations.
Deep dives
Stream processing platform for operational systems
Decodable is a stream processing platform based on Apache Flink and Debesium, designed to collect data from operational systems like databases and event streaming platforms, and process it in real time. It offers a range of capabilities such as data enrichment, filtering, joining, and aggregating using SQL or Java APIs. The platform aims to simplify the complex workflows involved in stream processing and data distribution between various systems, including microservices, databases, and analytical database systems. Decodable addresses the challenges of complex event processing, late arriving data, and state management, making it easier for organizations to adopt stream processing and integrate it seamlessly into their data infrastructure.
The role of event streaming and stream processing
The podcast discusses the importance of event streaming and stream processing in modern data management. Event streaming is defined as the durable storage and movement of data in real time, while stream processing involves the processing and connectivity of that data. The podcast emphasizes the natural aggregation points in the data platform: operational databases, event streaming platforms, and data warehouses. It proposes that the stream processing layer is the ideal component for data collection, cleansing, and distribution, while the data warehouse is better suited for further data refinement and enrichment. By leveraging both the event streaming layer and the data warehouse, organizations can optimize performance, data latency, and cost efficiency in their data workflows.
Enrichment using event streaming and stream processing
The podcast explores the concept of enrichment in event streaming and stream processing workflows. It highlights the benefits of performing data enrichment within the stream processing layer rather than relying solely on the data warehouse. Enrichment can involve joining data from various operational systems, such as databases and event streaming platforms, to enhance the information being processed. Decodable offers support for complex processing, state management, and sophisticated data enrichment, allowing organizations to perform enrichment directly within the stream processing layer. By leveraging SQL capabilities and integrating with various source and destination systems, decodable enables efficient and real-time enrichment, reducing latency and providing richer data for operational use cases and system integrations.
Building a Safe and Secure Cloud Service
The focus of this podcast episode is on the challenges and considerations of building a cloud service. The speaker highlights the importance of offering robust security measures and resource isolation when allowing customers to upload arbitrary code to the platform. The speaker explains the effort put into finding a safe, performant, and cost-effective solution for providing resource isolation and security. The episode also touches on secondary concerns such as managing access to certain parts of the system and ensuring data quality. Overall, the episode emphasizes the importance of resource management, security, and safety in the context of a cloud service.
Improving Developer Experience and Workflow
Another significant topic discussed in this podcast episode is the developer experience in using the decodable platform. The speaker discusses the challenge of creating a seamless and user-friendly experience for users with different workflows and preferences. Decodable aims to provide APIs, command line tools, and a user interface to cater to different personas, such as SQL-focused users, data engineers, and application developers. The goal is to fit into users' existing workflows and provide them with the tools and interfaces that make their work easier. The speaker acknowledges that there is still room for improvement and mentions future plans to enhance developer experience, particularly in areas like operational flows, reprocessing of data, and bootstrapping new infrastructure. Overall, the episode highlights the importance of prioritizing developer experience and enabling users to be productive in their day-to-day tasks.
Summary
Building streaming applications has gotten substantially easier over the past several years. Despite this, it is still operationally challenging to deploy and maintain your own stream processing infrastructure. Decodable was built with a mission of eliminating all of the painful aspects of developing and deploying stream processing systems for engineering teams. In this episode Eric Sammer discusses why more companies are including real-time capabilities in their products and the ways that Decodable makes it faster and easier.
Announcements
- Hello and welcome to the Data Engineering Podcast, the show about modern data management
- Introducing RudderStack Profiles. RudderStack Profiles takes the SaaS guesswork and SQL grunt work out of building complete customer profiles so you can quickly ship actionable, enriched data to every downstream team. You specify the customer traits, then Profiles runs the joins and computations for you to create complete customer profiles. Get all of the details and try the new product today at dataengineeringpodcast.com/rudderstack
- This episode is brought to you by Datafold – a testing automation platform for data engineers that finds data quality issues before the code and data are deployed to production. Datafold leverages data-diffing to compare production and development environments and column-level lineage to show you the exact impact of every code change on data, metrics, and BI tools, keeping your team productive and stakeholders happy. Datafold integrates with dbt, the modern data stack, and seamlessly plugs in your data CI for team-wide and automated testing. If you are migrating to a modern data stack, Datafold can also help you automate data and code validation to speed up the migration. Learn more about Datafold by visiting dataengineeringpodcast.com/datafold
- You shouldn't have to throw away the database to build with fast-changing data. You should be able to keep the familiarity of SQL and the proven architecture of cloud warehouses, but swap the decades-old batch computation model for an efficient incremental engine to get complex queries that are always up-to-date. With Materialize, you can! It’s the only true SQL streaming database built from the ground up to meet the needs of modern data products. Whether it’s real-time dashboarding and analytics, personalization and segmentation or automation and alerting, Materialize gives you the ability to work with fresh, correct, and scalable results — all in a familiar SQL interface. Go to dataengineeringpodcast.com/materialize today to get 2 weeks free!
- As more people start using AI for projects, two things are clear: It’s a rapidly advancing field, but it’s tough to navigate. How can you get the best results for your use case? Instead of being subjected to a bunch of buzzword bingo, hear directly from pioneers in the developer and data science space on how they use graph tech to build AI-powered apps. . Attend the dev and ML talks at NODES 2023, a free online conference on October 26 featuring some of the brightest minds in tech. Check out the agenda and register today at Neo4j.com/NODES.
- Your host is Tobias Macey and today I'm interviewing Eric Sammer about starting your stream processing journey with Decodable
Interview
- Introduction
- How did you get involved in the area of data management?
- Can you describe what Decodable is and the story behind it?
- What are the notable changes to the Decodable platform since we last spoke? (October 2021)
- What are the industry shifts that have influenced the product direction?
- What are the problems that customers are trying to solve when they come to Decodable?
- When you launched your focus was on SQL transformations of streaming data. What was the process for adding full Java support in addition to SQL?
- What are the developer experience challenges that are particular to working with streaming data?
- How have you worked to address that in the Decodable platform and interfaces?
- As you evolve the technical and product direction, what is your heuristic for balancing the unification of interfaces and system integration against the ability to swap different components or interfaces as new technologies are introduced?
- What are the most interesting, innovative, or unexpected ways that you have seen Decodable used?
- What are the most interesting, unexpected, or challenging lessons that you have learned while working on Decodable?
- When is Decodable the wrong choice?
- What do you have planned for the future of Decodable?
Contact Info
Parting Question
- From your perspective, what is the biggest gap in the tooling or technology for data management today?
Closing Announcements
- Thank you for listening! Don't forget to check out our other shows. Podcast.__init__ covers the Python language, its community, and the innovative ways it is being used. The Machine Learning Podcast helps you go from idea to production with machine learning.
- Visit the site to subscribe to the show, sign up for the mailing list, and read the show notes.
- If you've learned something or tried out a project from the show then tell us about it! Email hosts@dataengineeringpodcast.com) with your story.
- To help other people find the show please leave a review on Apple Podcasts and tell your friends and co-workers
Links
- Decodable
- Understanding the Apache Flink Journey
- Flink
- Debezium
- Kafka
- Redpanda
- Kinesis
- PostgreSQL
- Snowflake
- Databricks
- Startree
- Pinot
- Rockset
- Druid
- InfluxDB
- Samza
- Storm
- Pulsar
- ksqlDB
- dbt
- GitHub Actions
- Airbyte
- Singer
- Splunk
- Outbox Pattern
The intro and outro music is from The Hug by The Freak Fandango Orchestra / CC BY-SA
Sponsored By:
- Neo4J: 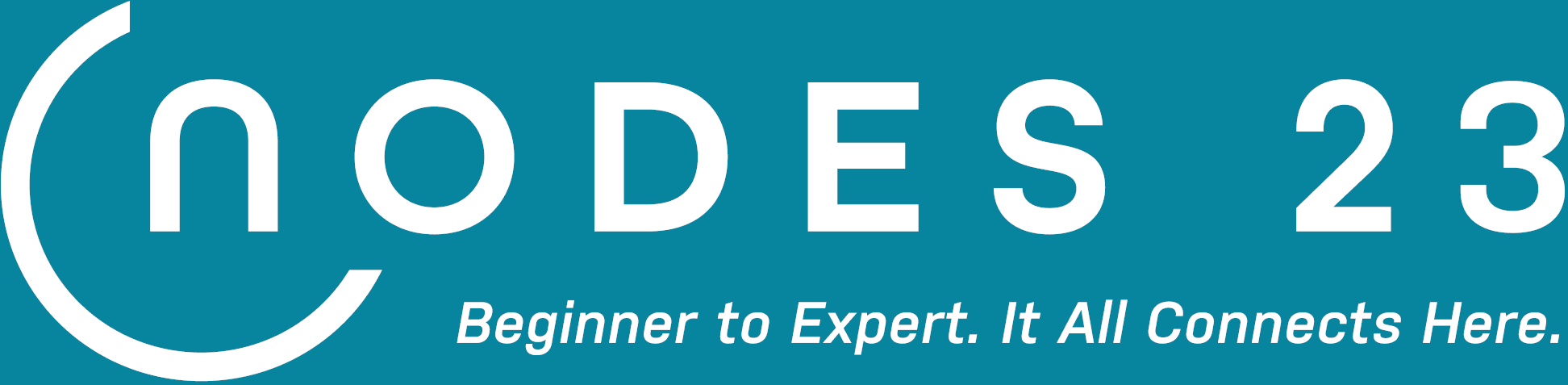 NODES 2023 is a free online conference focused on graph-driven innovations with content for all skill levels. Its 24 hours are packed with 90 interactive technical sessions from top developers and data scientists across the world covering a broad range of topics and use cases. The event tracks: - Intelligent Applications: APIs, Libraries, and Frameworks – Tools and best practices for creating graph-powered applications and APIs with any software stack and programming language, including Java, Python, and JavaScript - Machine Learning and AI – How graph technology provides context for your data and enhances the accuracy of your AI and ML projects (e.g.: graph neural networks, responsible AI) - Visualization: Tools, Techniques, and Best Practices – Techniques and tools for exploring hidden and unknown patterns in your data and presenting complex relationships (knowledge graphs, ethical data practices, and data representation) Don’t miss your chance to hear about the latest graph-powered implementations and best practices for free on October 26 at NODES 2023. Go to [Neo4j.com/NODES](https://Neo4j.com/NODES) today to see the full agenda and register!
- Rudderstack:  Introducing RudderStack Profiles. RudderStack Profiles takes the SaaS guesswork and SQL grunt work out of building complete customer profiles so you can quickly ship actionable, enriched data to every downstream team. You specify the customer traits, then Profiles runs the joins and computations for you to create complete customer profiles. Get all of the details and try the new product today at [dataengineeringpodcast.com/rudderstack](https://www.dataengineeringpodcast.com/rudderstack)
- Materialize: 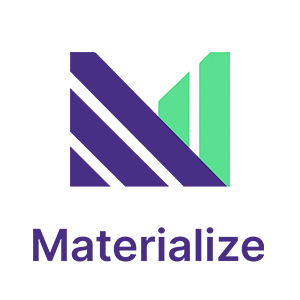 You shouldn't have to throw away the database to build with fast-changing data. Keep the familiar SQL, keep the proven architecture of cloud warehouses, but swap the decades-old batch computation model for an efficient incremental engine to get complex queries that are always up-to-date. That is Materialize, the only true SQL streaming database built from the ground up to meet the needs of modern data products: Fresh, Correct, Scalable — all in a familiar SQL UI. Built on Timely Dataflow and Differential Dataflow, open source frameworks created by cofounder Frank McSherry at Microsoft Research, Materialize is trusted by data and engineering teams at Ramp, Pluralsight, Onward and more to build real-time data products without the cost, complexity, and development time of stream processing. Go to [materialize.com](https://materialize.com/register/?utm_source=depodcast&utm_medium=paid&utm_campaign=early-access) today and get 2 weeks free!
- Datafold: 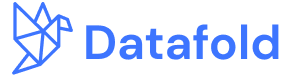 This episode is brought to you by Datafold – a testing automation platform for data engineers that finds data quality issues before the code and data are deployed to production. Datafold leverages data-diffing to compare…