
Find Out About The Technology Behind The Latest PFAD In Analytical Database Development
Data Engineering Podcast
Choosing Arrow for InfluxDB Data Handling
The transition to Arrow as the persistence format for InfluxDB was driven by the need for efficient compression and compatibility with third-party systems. Additionally, Arrow was selected as the in-memory data structure to enable fast analytics and support high cardinality and time series data queries, unlike the slower analytics queries experienced with previous versions of InfluxDB. This choice of Arrow as the in-memory format paved the way for improved analytical capabilities and integration with various query engines, including a consideration of DuckDB.
Summary
Building a database engine requires a substantial amount of engineering effort and time investment. Over the decades of research and development into building these software systems there are a number of common components that are shared across implementations. When Paul Dix decided to re-write the InfluxDB engine he found the Apache Arrow ecosystem ready and waiting with useful building blocks to accelerate the process. In this episode he explains how he used the combination of Apache Arrow, Flight, Datafusion, and Parquet to lay the foundation of the newest version of his time-series database.
Announcements
- Hello and welcome to the Data Engineering Podcast, the show about modern data management
- Dagster offers a new approach to building and running data platforms and data pipelines. It is an open-source, cloud-native orchestrator for the whole development lifecycle, with integrated lineage and observability, a declarative programming model, and best-in-class testability. Your team can get up and running in minutes thanks to Dagster Cloud, an enterprise-class hosted solution that offers serverless and hybrid deployments, enhanced security, and on-demand ephemeral test deployments. Go to dataengineeringpodcast.com/dagster today to get started. Your first 30 days are free!
- Data lakes are notoriously complex. For data engineers who battle to build and scale high quality data workflows on the data lake, Starburst powers petabyte-scale SQL analytics fast, at a fraction of the cost of traditional methods, so that you can meet all your data needs ranging from AI to data applications to complete analytics. Trusted by teams of all sizes, including Comcast and Doordash, Starburst is a data lake analytics platform that delivers the adaptability and flexibility a lakehouse ecosystem promises. And Starburst does all of this on an open architecture with first-class support for Apache Iceberg, Delta Lake and Hudi, so you always maintain ownership of your data. Want to see Starburst in action? Go to dataengineeringpodcast.com/starburst and get $500 in credits to try Starburst Galaxy today, the easiest and fastest way to get started using Trino.
- Join us at the top event for the global data community, Data Council Austin. From March 26-28th 2024, we'll play host to hundreds of attendees, 100 top speakers and dozens of startups that are advancing data science, engineering and AI. Data Council attendees are amazing founders, data scientists, lead engineers, CTOs, heads of data, investors and community organizers who are all working together to build the future of data and sharing their insights and learnings through deeply technical talks. As a listener to the Data Engineering Podcast you can get a special discount off regular priced and late bird tickets by using the promo code dataengpod20. Don't miss out on our only event this year! Visit dataengineeringpodcast.com/data-council and use code dataengpod20 to register today!
- Your host is Tobias Macey and today I'm interviewing Paul Dix about his investment in the Apache Arrow ecosystem and how it led him to create the latest PFAD in database design
Interview
- Introduction
- How did you get involved in the area of data management?
- Can you start by describing the FDAP stack and how the components combine to provide a foundational architecture for database engines?
- This was the core of your recent re-write of the InfluxDB engine. What were the design goals and constraints that led you to this architecture?
- Each of the architectural components are well engineered for their particular scope. What is the engineering work that is involved in building a cohesive platform from those components?
- One of the major benefits of using open source components is the network effect of ecosystem integrations. That can also be a risk when the community vision for the project doesn't align with your own goals. How have you worked to mitigate that risk in your specific platform?
- Can you describe the operational/architectural aspects of building a full data engine on top of the FDAP stack?
- What are the elements of the overall product/user experience that you had to build to create a cohesive platform?
- What are some of the other tools/technologies that can benefit from some or all of the pieces of the FDAP stack?
- What are the pieces of the Arrow ecosystem that are still immature or need further investment from the community?
- What are the most interesting, innovative, or unexpected ways that you have seen parts or all of the FDAP stack used?
- What are the most interesting, unexpected, or challenging lessons that you have learned while working on/with the FDAP stack?
- When is the FDAP stack the wrong choice?
- What do you have planned for the future of the InfluxDB IOx engine and the FDAP stack?
Contact Info
Parting Question
- From your perspective, what is the biggest gap in the tooling or technology for data management today?
Closing Announcements
- Thank you for listening! Don't forget to check out our other shows. Podcast.__init__ covers the Python language, its community, and the innovative ways it is being used. The Machine Learning Podcast helps you go from idea to production with machine learning.
- Visit the site to subscribe to the show, sign up for the mailing list, and read the show notes.
- If you've learned something or tried out a project from the show then tell us about it! Email hosts@dataengineeringpodcast.com) with your story.
Links
- FDAP Stack Blog Post
- Apache Arrow
- DataFusion
- Arrow Flight
- Apache Parquet
- InfluxDB
- Influx Data
- Rust Language
- DuckDB
- ClickHouse
- Voltron Data
- Velox
- Iceberg
- Trino
- ODBC == Open DataBase Connectivity
- GeoParquet
- ORC == Optimized Row Columnar
- Avro
- Protocol Buffers
- gRPC
The intro and outro music is from The Hug by The Freak Fandango Orchestra / CC BY-SA
Sponsored By:
- Starburst: 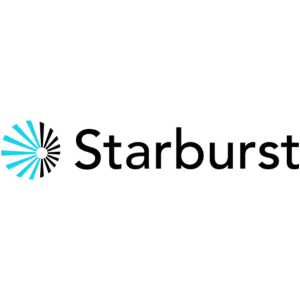 This episode is brought to you by Starburst - a data lake analytics platform for data engineers who are battling to build and scale high quality data pipelines on the data lake. Powered by Trino, Starburst runs petabyte-scale SQL analytics fast at a fraction of the cost of traditional methods, helping you meet all your data needs ranging from AI/ML workloads to data applications to complete analytics. Trusted by the teams at Comcast and Doordash, Starburst delivers the adaptability and flexibility a lakehouse ecosystem promises, while providing a single point of access for your data and all your data governance allowing you to discover, transform, govern, and secure all in one place. Starburst does all of this on an open architecture with first-class support for Apache Iceberg, Delta Lake and Hudi, so you always maintain ownership of your data. Want to see Starburst in action? Try Starburst Galaxy today, the easiest and fastest way to get started using Trino, and get $500 of credits free. [dataengineeringpodcast.com/starburst](https://www.dataengineeringpodcast.com/starburst)
- Data Council: 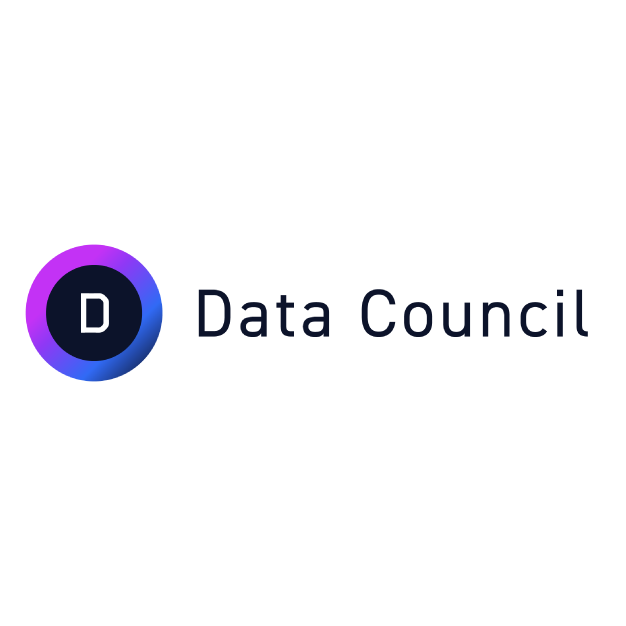 Join us at the top event for the global data community, Data Council Austin. From March 26-28th 2024, we'll play host to hundreds of attendees, 100 top speakers and dozens of startups that are advancing data science, engineering and AI. Data Council attendees are amazing founders, data scientists, lead engineers, CTOs, heads of data, investors and community organizers who are all working together to build the future of data and sharing their insights and learnings through deeply technical talks. As a listener to the Data Engineering Podcast you can get a special discount off regular priced and late bird tickets by using the promo code dataengpod20. Don't miss out on our only event this year! Visit [dataengineeringpodcast.com/data-council](https://www.dataengineeringpodcast.com/data-council) and use code **dataengpod20** to register today! Promo Code: dataengpod20
- Dagster: 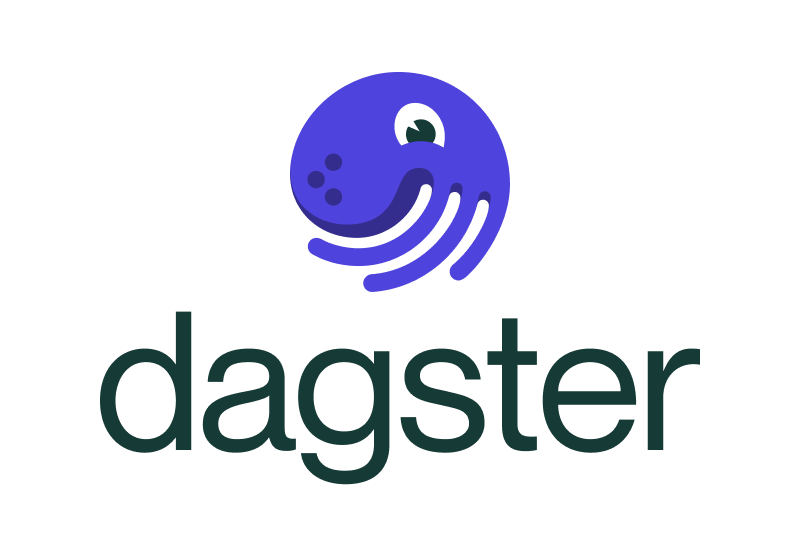 Data teams are tasked with helping organizations deliver on the premise of data, and with ML and AI maturing rapidly, expectations have never been this high. However data engineers are challenged by both technical complexity and organizational complexity, with heterogeneous technologies to adopt, multiple data disciplines converging, legacy systems to support, and costs to manage. Dagster is an open-source orchestration solution that helps data teams reign in this complexity and build data platforms that provide unparalleled observability, and testability, all while fostering collaboration across the enterprise. With enterprise-grade hosting on Dagster Cloud, you gain even more capabilities, adding cost management, security, and CI support to further boost your teams' productivity. Go to [dagster.io](https://dagster.io/lp/dagster-cloud-trial?source=data-eng-podcast) today to get your first 30 days free!