
Data Engineering Podcast
This show goes behind the scenes for the tools, techniques, and difficulties associated with the discipline of data engineering. Databases, workflows, automation, and data manipulation are just some of the topics that you will find here.
Latest episodes

Dec 19, 2022 • 47min
Making Sense Of The Technical And Organizational Considerations Of Data Contracts
Summary
One of the reasons that data work is so challenging is because no single person or team owns the entire process. This introduces friction in the process of collecting, processing, and using data. In order to reduce the potential for broken pipelines some teams have started to adopt the idea of data contracts. In this episode Abe Gong brings his experiences with the Great Expectations project and community to discuss the technical and organizational considerations involved in implementing these constraints to your data workflows.
Announcements
Hello and welcome to the Data Engineering Podcast, the show about modern data management
When you're ready to build your next pipeline, or want to test out the projects you hear about on the show, you'll need somewhere to deploy it, so check out our friends at Linode. With their new managed database service you can launch a production ready MySQL, Postgres, or MongoDB cluster in minutes, with automated backups, 40 Gbps connections from your application hosts, and high throughput SSDs. Go to dataengineeringpodcast.com/linode today and get a $100 credit to launch a database, create a Kubernetes cluster, or take advantage of all of their other services. And don't forget to thank them for their continued support of this show!
Atlan is the metadata hub for your data ecosystem. Instead of locking your metadata into a new silo, unleash its transformative potential with Atlan's active metadata capabilities. Push information about data freshness and quality to your business intelligence, automatically scale up and down your warehouse based on usage patterns, and let the bots answer those questions in Slack so that the humans can focus on delivering real value. Go to dataengineeringpodcast.com/atlan today to learn more about how Atlan’s active metadata platform is helping pioneering data teams like Postman, Plaid, WeWork & Unilever achieve extraordinary things with metadata and escape the chaos.
Struggling with broken pipelines? Stale dashboards? Missing data? If this resonates with you, you’re not alone. Data engineers struggling with unreliable data need look no further than Monte Carlo, the leading end-to-end Data Observability Platform! Trusted by the data teams at Fox, JetBlue, and PagerDuty, Monte Carlo solves the costly problem of broken data pipelines. Monte Carlo monitors and alerts for data issues across your data warehouses, data lakes, dbt models, Airflow jobs, and business intelligence tools, reducing time to detection and resolution from weeks to just minutes. Monte Carlo also gives you a holistic picture of data health with automatic, end-to-end lineage from ingestion to the BI layer directly out of the box. Start trusting your data with Monte Carlo today! Visit dataengineeringpodcast.com/montecarlo to learn more.
Your host is Tobias Macey and today I'm interviewing Abe Gong about the technical and organizational implementation of data contracts
Interview
Introduction
How did you get involved in the area of data management?
Can you describe what your conception of a data contract is?
What are some of the ways that you have seen them implemented?
How has your work on Great Expectations influenced your thinking on the strategic and tactical aspects of adopting/implementing data contracts in a given team/organization?
What does the negotiation process look like for identifying what needs to be included in a contract?
What are the interfaces/integration points where data contracts are most useful/necessary?
What are the discussions that need to happen when deciding when/whether a contract "violation" is a blocking action vs. issuing a notification?
At what level of detail/granularity are contracts most helpful?
At the technical level, what does the implementation/integration/deployment of a contract look like?
What are the most interesting, innovative, or unexpected ways that you have seen data contracts used?
What are the most interesting, unexpected, or challenging lessons that you have learned while working on data contracts/great expectations?
When are data contracts the wrong choice?
What do you have planned for the future of data contracts in great expectations?
Contact Info
LinkedIn
@AbeGong on Twitter
Website
Parting Question
From your perspective, what is the biggest gap in the tooling or technology for data management today?
Closing Announcements
Thank you for listening! Don't forget to check out our other shows. Podcast.__init__ covers the Python language, its community, and the innovative ways it is being used. The Machine Learning Podcast helps you go from idea to production with machine learning.
Visit the site to subscribe to the show, sign up for the mailing list, and read the show notes.
If you've learned something or tried out a project from the show then tell us about it! Email hosts@dataengineeringpodcast.com) with your story.
To help other people find the show please leave a review on Apple Podcasts and tell your friends and co-workers
Links
Great Expectations
Podcast Episode
Progressive Typing
Pioneers, Settlers, Town Planners
Pydantic
Podcast.__init__ Episode
Typescript
Duck Typing
Flyte
Podcast Episode
Dagster
Podcast Episode
Trino
The intro and outro music is from The Hug by The Freak Fandango Orchestra / CC BY-SASponsored By:MonteCarlo: 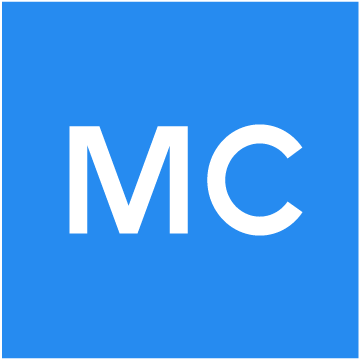
Struggling with broken pipelines? Stale dashboards? Missing data?
If this resonates with you, you’re not alone. Data engineers struggling with unreliable data need look no further than Monte Carlo, the leading end-to-end Data Observability Platform!
Trusted by the data teams at Fox, JetBlue, and PagerDuty, Monte Carlo solves the costly problem of broken data pipelines. Monte Carlo monitors and alerts for data issues across your data warehouses, data lakes, dbt models, Airflow jobs, and business intelligence tools, reducing time to detection and resolution from weeks to just minutes. Monte Carlo also gives you a holistic picture of data health with automatic, end-to-end lineage from ingestion to the BI layer directly out of the box. Start trusting your data with Monte Carlo today!
Visit [dataengineeringpodcast.com/montecarlo](https://www.dataengineeringpodcast.com/montecarlo) to learn more.Linode: 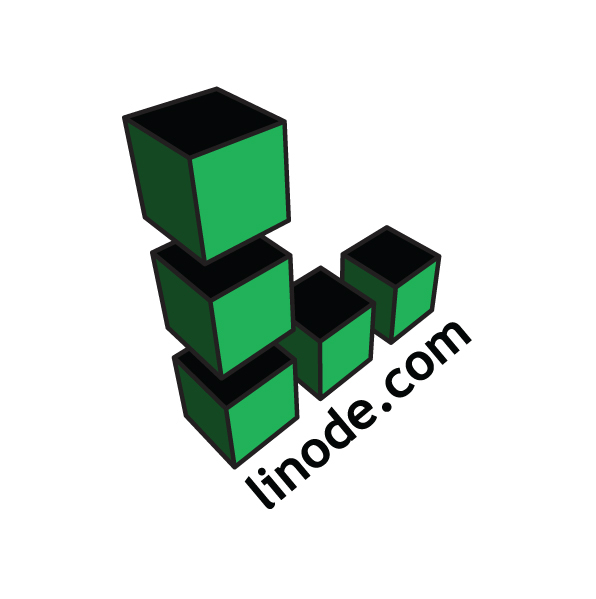
Your data platform needs to be scalable, fault tolerant, and performant, which means that you need the same from your cloud provider. Linode has been powering production systems for over 17 years, and now they’ve launched a fully managed Kubernetes platform. With the combined power of the Kubernetes engine for flexible and scalable deployments, and features like dedicated CPU instances, GPU instances, and object storage you’ve got everything you need to build a bulletproof data pipeline. If you go to: [dataengineeringpodcast.com/linode](https://www.dataengineeringpodcast.com/linode) today you’ll even get a $100 credit to use on building your own cluster, or object storage, or reliable backups, or… And while you’re there don’t forget to thank them for being a long-time supporter of the Data Engineering Podcast!Atlan: 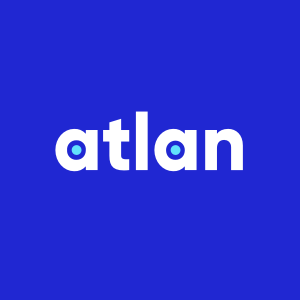
Have you ever woken up to a crisis because a number on a dashboard is broken and no one knows why? Or sent out frustrating slack messages trying to find the right data set? Or tried to understand what a column name means?
Our friends at Atlan started out as a data team themselves and faced all this collaboration chaos themselves, and started building Atlan as an internal tool for themselves. Atlan is a collaborative workspace for data-driven teams, like Github for engineering or Figma for design teams. By acting as a virtual hub for data assets ranging from tables and dashboards to SQL snippets & code, Atlan enables teams to create a single source of truth for all their data assets, and collaborate across the modern data stack through deep integrations with tools like Snowflake, Slack, Looker and more.
Go to [dataengineeringpodcast.com/atlan](https://www.dataengineeringpodcast.com/atlan) and sign up for a free trial. If you’re a data engineering podcast listener, you get credits worth $3000 on an annual subscription.Support Data Engineering Podcast

18 snips
Dec 19, 2022 • 1h 5min
Revisit The Fundamental Principles Of Working With Data To Avoid Getting Caught In The Hype Cycle
Summary
The data ecosystem has seen a constant flurry of activity for the past several years, and it shows no signs of slowing down. With all of the products, techniques, and buzzwords being discussed it can be easy to be overcome by the hype. In this episode Juan Sequeda and Tim Gasper from data.world share their views on the core principles that you can use to ground your work and avoid getting caught in the hype cycles.
Announcements
Hello and welcome to the Data Engineering Podcast, the show about modern data management
When you're ready to build your next pipeline, or want to test out the projects you hear about on the show, you'll need somewhere to deploy it, so check out our friends at Linode. With their new managed database service you can launch a production ready MySQL, Postgres, or MongoDB cluster in minutes, with automated backups, 40 Gbps connections from your application hosts, and high throughput SSDs. Go to dataengineeringpodcast.com/linode today and get a $100 credit to launch a database, create a Kubernetes cluster, or take advantage of all of their other services. And don't forget to thank them for their continued support of this show!
Modern data teams are dealing with a lot of complexity in their data pipelines and analytical code. Monitoring data quality, tracing incidents, and testing changes can be daunting and often takes hours to days or even weeks. By the time errors have made their way into production, it’s often too late and damage is done. Datafold built automated regression testing to help data and analytics engineers deal with data quality in their pull requests. Datafold shows how a change in SQL code affects your data, both on a statistical level and down to individual rows and values before it gets merged to production. No more shipping and praying, you can now know exactly what will change in your database! Datafold integrates with all major data warehouses as well as frameworks such as Airflow & dbt and seamlessly plugs into CI workflows. Visit dataengineeringpodcast.com/datafold today to book a demo with Datafold.
RudderStack helps you build a customer data platform on your warehouse or data lake. Instead of trapping data in a black box, they enable you to easily collect customer data from the entire stack and build an identity graph on your warehouse, giving you full visibility and control. Their SDKs make event streaming from any app or website easy, and their extensive library of integrations enable you to automatically send data to hundreds of downstream tools. Sign up free at dataengineeringpodcast.com/rudder
Build Data Pipelines. Not DAGs. That’s the spirit behind Upsolver SQLake, a new self-service data pipeline platform that lets you build batch and streaming pipelines without falling into the black hole of DAG-based orchestration. All you do is write a query in SQL to declare your transformation, and SQLake will turn it into a continuous pipeline that scales to petabytes and delivers up to the minute fresh data. SQLake supports a broad set of transformations, including high-cardinality joins, aggregations, upserts and window operations. Output data can be streamed into a data lake for query engines like Presto, Trino or Spark SQL, a data warehouse like Snowflake or Redshift., or any other destination you choose. Pricing for SQLake is simple. You pay $99 per terabyte ingested into your data lake using SQLake, and run unlimited transformation pipelines for free. That way data engineers and data users can process to their heart’s content without worrying about their cloud bill. For data engineering podcast listeners, we’re offering a 30 day trial with unlimited data, so go to dataengineeringpodcast.com/upsolver today and see for yourself how to avoid DAG hell.
Your host is Tobias Macey and today I'm interviewing Juan Sequeda and Tim Gasper about their views on the role of the data mesh paradigm for driving re-assessment of the foundational principles of data systems
Interview
Introduction
How did you get involved in the area of data management?
What are the areas of the data ecosystem that you see the most turmoil and confusion?
The past couple of years have brought a lot of attention to the idea of the "modern data stack". How has that influenced the ways that your and your customers' teams think about what skills they need to be effective?
The other topic that is introducing a lot of confusion and uncertainty is the "data mesh". How has that changed the ways that teams think about who is involved in the technical and design conversations around data in an organization?
Now that we, as an industry, have reached a new generational inflection about how data is generated, processed, and used, what are some of the foundational principles that have proven their worth?
What are some of the new lessons that are showing the greatest promise?
data modeling
data platform/infrastructure
data collaboration
data governance/security/privacy
How does your work at data.world work support these foundational practices?
What are some of the ways that you work with your teams and customers to help them stay informed on industry practices?
What is your process for understanding the balance between hype and reality as you encounter new ideas/technologies?
What are some of the notable changes that have happened in the data.world product and market since I last had Bryon on the show in 2017?
What are the most interesting, innovative, or unexpected ways that you have seen data.world used?
What are the most interesting, unexpected, or challenging lessons that you have learned while working on data.world?
When is data.world the wrong choice?
What do you have planned for the future of data.world?
Contact Info
Juan
LinkedIn
@juansequeda on Twitter
Website
Tim
LinkedIn
@TimGasper on Twitter
Website
Parting Question
From your perspective, what is the biggest gap in the tooling or technology for data management today?
Closing Announcements
Thank you for listening! Don't forget to check out our other shows. Podcast.__init__ covers the Python language, its community, and the innovative ways it is being used. The Machine Learning Podcast helps you go from idea to production with machine learning.
Visit the site to subscribe to the show, sign up for the mailing list, and read the show notes.
If you've learned something or tried out a project from the show then tell us about it! Email hosts@dataengineeringpodcast.com) with your story.
To help other people find the show please leave a review on Apple Podcasts and tell your friends and co-workers
Links
data.world
Podcast Episode
Gartner Hype Cycle
Data Mesh
Modern Data Stack
DataOps
Data Observability
Data & AI Landscape
DataDog
RDF == Resource Description Framework
SPARQL
Moshe Vardi
Star Schema
Data Vault
Podcast Episode
BPMN == Business Process Modeling Notation
The intro and outro music is from The Hug by The Freak Fandango Orchestra / CC BY-SASponsored By:Upsolver: 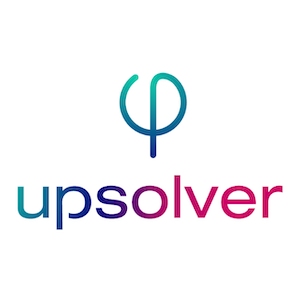
Build Real-Time Pipelines. Not Endless DAGs!
Creating real-time ETL pipelines is extremely time-consuming and engineering intensive. Why? Because when we attempt to shoehorn a 30-year old batch process into a real-time pipeline, we create an orchestration hell that makes every pipeline a data engineering project.
Every pipeline is composed of transformation logic (the what) and orchestration (the how). If you run daily batches, orchestration is simple and there’s plenty of time to recover from failures. However, real-time pipelines with per-hour or per-minute batches make orchestration intricate and data engineers find themselves burdened with building Direct Acyclic Graphs (DAGs), in tools like Apache Airflow, with 10s to 100s of steps intended to address all success and failure modes, task dependencies and maintain temporary data copies.
Ori Rafael, CEO and co-founder of Upsolver, will unpack this problem that bottlenecks real-time analytics delivery, and describe a new approach that completely eliminates the need for orchestration, so you can remove Airflow from your development critical path and deliver reliable production pipelines quickly.
Go to [dataengineeringpodcast.com/upsolver](dataengineeringpodcast.com/upsolver) to start your 30 day trial with unlimited data, and see for yourself how to avoid DAG hell.Datafold: 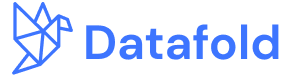
Datafold helps you deal with data quality in your pull request. It provides automated regression testing throughout your schema and pipelines so you can address quality issues before they affect production. No more shipping and praying, you can now know exactly what will change in your database ahead of time.
Datafold integrates with all major data warehouses as well as frameworks such as Airflow & dbt and seamlessly plugs into CI, so in a few minutes you can get from 0 to automated testing of your analytical code. Visit our site at [dataengineeringpodcast.com/datafold](https://www.dataengineeringpodcast.com/datafold)
today to book a demo with Datafold.Rudderstack: 
RudderStack provides all your customer data pipelines in one platform. You can collect, transform, and route data across your entire stack with its event streaming, ETL, and reverse ETL pipelines.
RudderStack’s warehouse-first approach means it does not store sensitive information, and it allows you to leverage your existing data warehouse/data lake infrastructure to build a single source of truth for every team.
RudderStack also supports real-time use cases. You can Implement RudderStack SDKs once, then automatically send events to your warehouse and 150+ business tools, and you’ll never have to worry about API changes again.
Visit [dataengineeringpodcast.com/rudderstack](https://www.dataengineeringpodcast.com/rudderstack) to sign up for free today, and snag a free T-Shirt just for being a Data Engineering Podcast listener.Linode: 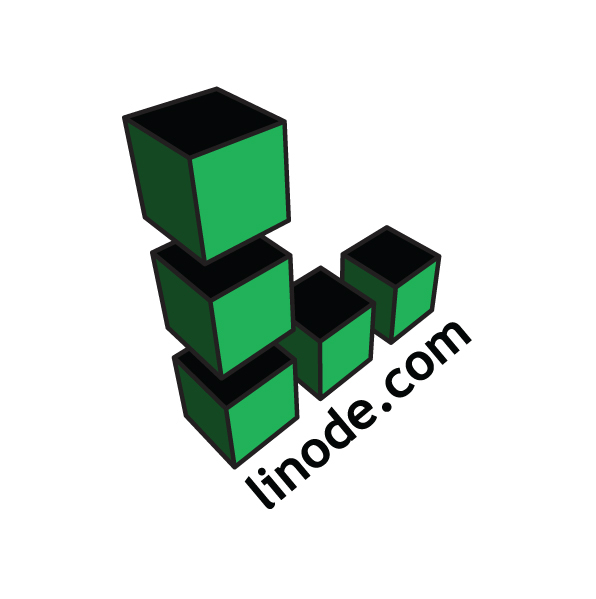
Your data platform needs to be scalable, fault tolerant, and performant, which means that you need the same from your cloud provider. Linode has been powering production systems for over 17 years, and now they’ve launched a fully managed Kubernetes platform. With the combined power of the Kubernetes engine for flexible and scalable deployments, and features like dedicated CPU instances, GPU instances, and object storage you’ve got everything you need to build a bulletproof data pipeline. If you go to: [dataengineeringpodcast.com/linode](https://www.dataengineeringpodcast.com/linode) today you’ll even get a…

Dec 12, 2022 • 54min
Convert Your Unstructured Data To Embedding Vectors For More Efficient Machine Learning With Towhee
Preamble
This is a cross-over episode from our new show The Machine Learning Podcast, the show about going from idea to production with machine learning.
Summary
Data is one of the core ingredients for machine learning, but the format in which it is understandable to humans is not a useful representation for models. Embedding vectors are a way to structure data in a way that is native to how models interpret and manipulate information. In this episode Frank Liu shares how the Towhee library simplifies the work of translating your unstructured data assets (e.g. images, audio, video, etc.) into embeddings that you can use efficiently for machine learning, and how it fits into your workflow for model development.
Announcements
Hello and welcome to the Machine Learning Podcast, the podcast about machine learning and how to bring it from idea to delivery.
Building good ML models is hard, but testing them properly is even harder. At Deepchecks, they built an open-source testing framework that follows best practices, ensuring that your models behave as expected. Get started quickly using their built-in library of checks for testing and validating your model’s behavior and performance, and extend it to meet your specific needs as your model evolves. Accelerate your machine learning projects by building trust in your models and automating the testing that you used to do manually. Go to themachinelearningpodcast.com/deepchecks today to get started!
Your host is Tobias Macey and today I’m interviewing Frank Liu about how to use vector embeddings in your ML projects and how Towhee can reduce the effort involved
Interview
Introduction
How did you get involved in machine learning?
Can you describe what Towhee is and the story behind it?
What is the problem that Towhee is aimed at solving?
What are the elements of generating vector embeddings that pose the greatest challenge or require the most effort?
Once you have an embedding, what are some of the ways that it might be used in a machine learning project?
Are there any design considerations that need to be addressed in the form that an embedding takes and how it impacts the resultant model that relies on it? (whether for training or inference)
Can you describe how the Towhee framework is implemented?
What are some of the interesting engineering challenges that needed to be addressed?
How have the design/goals/scope of the project shifted since it began?
What is the workflow for someone using Towhee in the context of an ML project?
What are some of the types optimizations that you have incorporated into Towhee?
What are some of the scaling considerations that users need to be aware of as they increase the volume or complexity of data that they are processing?
What are some of the ways that using Towhee impacts the way a data scientist or ML engineer approach the design development of their model code?
What are the interfaces available for integrating with and extending Towhee?
What are the most interesting, innovative, or unexpected ways that you have seen Towhee used?
What are the most interesting, unexpected, or challenging lessons that you have learned while working on Towhee?
When is Towhee the wrong choice?
What do you have planned for the future of Towhee?
Contact Info
LinkedIn
fzliu on GitHub
Website
@frankzliu on Twitter
Parting Question
From your perspective, what is the biggest barrier to adoption of machine learning today?
Closing Announcements
Thank you for listening! Don’t forget to check out our other shows. The Data Engineering Podcast covers the latest on modern data management. Podcast.__init__ covers the Python language, its community, and the innovative ways it is being used.
Visit the site to subscribe to the show, sign up for the mailing list, and read the show notes.
If you’ve learned something or tried out a project from the show then tell us about it! Email hosts@themachinelearningpodcast.com) with your story.
To help other people find the show please leave a review on iTunes and tell your friends and co-workers
Links
Towhee
Zilliz
Milvus
Data Engineering Podcast Episode
Computer Vision
Tensor
Autoencoder
Latent Space
Diffusion Model
HSL == Hue, Saturation, Lightness
Weights and Biases
The intro and outro music is from Hitman’s Lovesong feat. Paola Graziano by The Freak Fandango Orchestra/CC BY-SA 3.0
Support Data Engineering Podcast

Dec 12, 2022 • 50min
Run Your Applications Worldwide Without Worrying About The Database With Planetscale
Summary
One of the most critical aspects of software projects is managing its data. Managing the operational concerns for your database can be complex and expensive, especially if you need to scale to large volumes of data, high traffic, or geographically distributed usage. Planetscale is a serverless option for your MySQL workloads that lets you focus on your applications without having to worry about managing the database or fight with differences between development and production. In this episode Nick van Wiggeren explains how the Planetscale platform is implemented, their strategies for balancing maintenance and improvements of the underlying Vitess project with their business goals, and how you can start using it today to free up the time you spend on database administration.
Announcements
Hello and welcome to the Data Engineering Podcast, the show about modern data management
When you’re ready to build your next pipeline, or want to test out the projects you hear about on the show, you’ll need somewhere to deploy it, so check out our friends at Linode. With their new managed database service you can launch a production ready MySQL, Postgres, or MongoDB cluster in minutes, with automated backups, 40 Gbps connections from your application hosts, and high throughput SSDs. Go to dataengineeringpodcast.com/linode today and get a $100 credit to launch a database, create a Kubernetes cluster, or take advantage of all of their other services. And don’t forget to thank them for their continued support of this show!
Modern data teams are dealing with a lot of complexity in their data pipelines and analytical code. Monitoring data quality, tracing incidents, and testing changes can be daunting and often takes hours to days or even weeks. By the time errors have made their way into production, it’s often too late and damage is done. Datafold built automated regression testing to help data and analytics engineers deal with data quality in their pull requests. Datafold shows how a change in SQL code affects your data, both on a statistical level and down to individual rows and values before it gets merged to production. No more shipping and praying, you can now know exactly what will change in your database! Datafold integrates with all major data warehouses as well as frameworks such as Airflow & dbt and seamlessly plugs into CI workflows. Visit dataengineeringpodcast.com/datafold today to book a demo with Datafold.
RudderStack helps you build a customer data platform on your warehouse or data lake. Instead of trapping data in a black box, they enable you to easily collect customer data from the entire stack and build an identity graph on your warehouse, giving you full visibility and control. Their SDKs make event streaming from any app or website easy, and their extensive library of integrations enable you to automatically send data to hundreds of downstream tools. Sign up free at dataengineeringpodcast.com/rudder
Build Data Pipelines. Not DAGs. That’s the spirit behind Upsolver SQLake, a new self-service data pipeline platform that lets you build batch and streaming pipelines without falling into the black hole of DAG-based orchestration. All you do is write a query in SQL to declare your transformation, and SQLake will turn it into a continuous pipeline that scales to petabytes and delivers up to the minute fresh data. SQLake supports a broad set of transformations, including high-cardinality joins, aggregations, upserts and window operations. Output data can be streamed into a data lake for query engines like Presto, Trino or Spark SQL, a data warehouse like Snowflake or Redshift., or any other destination you choose. Pricing for SQLake is simple. You pay $99 per terabyte ingested into your data lake using SQLake, and run unlimited transformation pipelines for free. That way data engineers and data users can process to their heart’s content without worrying about their cloud bill. For data engineering podcast listeners, we’re offering a 30 day trial with unlimited data, so go to dataengineeringpodcast.com/upsolver today and see for yourself how to avoid DAG hell.
Your host is Tobias Macey and today I’m interviewing Nick van Wiggeren about Planetscale, a serverless and globally distributed MySQL database as a service
Interview
Introduction
How did you get involved in the area of data management?
Can you describe what Planetscale is and the story behind it?
What are the core problems that you are solving with the Planetscale platform?
How might an engineering team address those challenges in the absence of Planetscale/Vitess?
Can you describe how Planetscale is implemented?
What are some of the addons that you have had to build on top of Vitess to make Planetscale
What are the impacts that a serverless database has on the way teams approach their application/platform design and development?
metrics exposed to help users optimize their usage
What is your policy/philosophy for determining what capabilities to include in Vitess and what belongs in the Planetscale platform?
What are the most interesting, innovative, or unexpected ways that you have seen Planetscale/Vitess used?
What are the most interesting, unexpected, or challenging lessons that you have learned while working on Planetscale?
When is Planetscale the wrong choice?
What do you have planned for the future of Planetscale?
Contact Info
@nickvanwig on Twitter
LinkedIn
nickvanw on GitHub
Parting Question
From your perspective, what is the biggest gap in the tooling or technology for data management today?
Closing Announcements
Thank you for listening! Don’t forget to check out our other shows. Podcast.__init__ covers the Python language, its community, and the innovative ways it is being used. The Machine Learning Podcast helps you go from idea to production with machine learning.
Visit the site to subscribe to the show, sign up for the mailing list, and read the show notes.
If you’ve learned something or tried out a project from the show then tell us about it! Email hosts@dataengineeringpodcast.com) with your story.
To help other people find the show please leave a review on Apple Podcasts and tell your friends and co-workers
Links
Planetscale
Vitess
CNCF == Cloud Native Computing Foundation
Hadoop
OLTP == Online Transactional Processing
Galera
Yugabyte DB
Podcast Episode
CitusDB
MariaDB SkySQL
Podcast Episode
CockroachDB
Podcast Episode
NewSQL
AWS PrivateLink
Planetscale Connect
Segment
Podcast Episode
BigQuery
The intro and outro music is from The Hug by The Freak Fandango Orchestra / CC BY-SA
Support Data Engineering Podcast

Dec 5, 2022 • 47min
Business Intelligence In The Palm Of Your Hand With Zing Data
Summary
Business intelligence is the foremost application of data in organizations of all sizes. The typical conception of how it is accessed is through a web or desktop application running on a powerful laptop. Zing Data is building a mobile native platform for business intelligence. This opens the door for busy employees to access and analyze their company information away from their desk, but it has the more powerful effect of bringing first-class support to companies operating in mobile-first economies. In this episode Sabin Thomas shares his experiences building the platform and the interesting ways that it is being used.
Announcements
Hello and welcome to the Data Engineering Podcast, the show about modern data management
When you’re ready to build your next pipeline, or want to test out the projects you hear about on the show, you’ll need somewhere to deploy it, so check out our friends at Linode. With their new managed database service you can launch a production ready MySQL, Postgres, or MongoDB cluster in minutes, with automated backups, 40 Gbps connections from your application hosts, and high throughput SSDs. Go to dataengineeringpodcast.com/linode today and get a $100 credit to launch a database, create a Kubernetes cluster, or take advantage of all of their other services. And don’t forget to thank them for their continued support of this show!
Atlan is the metadata hub for your data ecosystem. Instead of locking your metadata into a new silo, unleash its transformative potential with Atlan’s active metadata capabilities. Push information about data freshness and quality to your business intelligence, automatically scale up and down your warehouse based on usage patterns, and let the bots answer those questions in Slack so that the humans can focus on delivering real value. Go to dataengineeringpodcast.com/atlan today to learn more about how Atlan’s active metadata platform is helping pioneering data teams like Postman, Plaid, WeWork & Unilever achieve extraordinary things with metadata and escape the chaos.
Data engineers don’t enjoy writing, maintaining, and modifying ETL pipelines all day, every day. Especially once they realize 90% of all major data sources like Google Analytics, Salesforce, Adwords, Facebook, Spreadsheets, etc., are already available as plug-and-play connectors with reliable, intuitive SaaS solutions. Hevo Data is a highly reliable and intuitive data pipeline platform used by data engineers from 40+ countries to set up and run low-latency ELT pipelines with zero maintenance. Boasting more than 150 out-of-the-box connectors that can be set up in minutes, Hevo also allows you to monitor and control your pipelines. You get: real-time data flow visibility, fail-safe mechanisms, and alerts if anything breaks; preload transformations and auto-schema mapping precisely control how data lands in your destination; models and workflows to transform data for analytics; and reverse-ETL capability to move the transformed data back to your business software to inspire timely action. All of this, plus its transparent pricing and 24*7 live support, makes it consistently voted by users as the Leader in the Data Pipeline category on review platforms like G2. Go to dataengineeringpodcast.com/hevodata and sign up for a free 14-day trial that also comes with 24×7 support.
Struggling with broken pipelines? Stale dashboards? Missing data? If this resonates with you, you’re not alone. Data engineers struggling with unreliable data need look no further than Monte Carlo, the leading end-to-end Data Observability Platform! Trusted by the data teams at Fox, JetBlue, and PagerDuty, Monte Carlo solves the costly problem of broken data pipelines. Monte Carlo monitors and alerts for data issues across your data warehouses, data lakes, dbt models, Airflow jobs, and business intelligence tools, reducing time to detection and resolution from weeks to just minutes. Monte Carlo also gives you a holistic picture of data health with automatic, end-to-end lineage from ingestion to the BI layer directly out of the box. Start trusting your data with Monte Carlo today! Visit dataengineeringpodcast.com/montecarlo to learn more.
Your host is Tobias Macey and today I’m interviewing Sabin Thomas about Zing Data, a mobile-friendly business intelligence platform
Interview
Introduction
How did you get involved in the area of data management?
Can you describe what Zing Data is and the story behind it?
Why is mobile access to a business intelligence system important?
What does it mean for a business intelligence system to be mobile friendly? (e.g. just looking at charts vs. creating reports, etc.)
What are the interaction patterns that don’t translate well to mobile from web or desktop BI systems?
What are the new interaction patterns that are enabled by the mobile experience?
What are the capabilities that a native app can provide which would be clunky or impossible as a web app on a mobile device?
Who are the personas that benefit from a product like Zing Data?
Can you describe how the platform (backend and app) are implemented?
How have the design and goals of the system changed/evolved since you started working on it?
Can you describe a typical workflow for a team that uses Zing?
Is it typically the sole/primary BI system, or is it more of an augmentation?
What are the most interesting, innovative, or unexpected ways that you have seen Zing used?
What are the most interesting, unexpected, or challenging lessons that you have learned while working on Zing?
When is Zing the wrong choice?
What do you have planned for the future of Zing Data?
Contact Info
LinkedIn
Parting Question
From your perspective, what is the biggest gap in the tooling or technology for data management today?
Closing Announcements
Thank you for listening! Don’t forget to check out our other shows. Podcast.__init__ covers the Python language, its community, and the innovative ways it is being used. The Machine Learning Podcast helps you go from idea to production with machine learning.
Visit the site to subscribe to the show, sign up for the mailing list, and read the show notes.
If you’ve learned something or tried out a project from the show then tell us about it! Email hosts@dataengineeringpodcast.com) with your story.
To help other people find the show please leave a review on Apple Podcasts and tell your friends and co-workers
Links
Zing Data
Rakuten
Flutter
Cordova
React Native
T-SQL
ANSI SQL
The intro and outro music is from The Hug by The Freak Fandango Orchestra / CC BY-SA
Support Data Engineering Podcast

11 snips
Dec 5, 2022 • 50min
Adopting Real-Time Data At Organizations Of Every Size
Summary
The term "real-time data" brings with it a combination of excitement, uncertainty, and skepticism. The promise of insights that are always accurate and up to date is appealing to organizations, but the technical realities to make it possible have been complex and expensive. In this episode Arjun Narayan explains how the technical barriers to adopting real-time data in your analytics and applications have become surmountable by organizations of all sizes.
Announcements
Hello and welcome to the Data Engineering Podcast, the show about modern data management
When you’re ready to build your next pipeline, or want to test out the projects you hear about on the show, you’ll need somewhere to deploy it, so check out our friends at Linode. With their new managed database service you can launch a production ready MySQL, Postgres, or MongoDB cluster in minutes, with automated backups, 40 Gbps connections from your application hosts, and high throughput SSDs. Go to dataengineeringpodcast.com/linode today and get a $100 credit to launch a database, create a Kubernetes cluster, or take advantage of all of their other services. And don’t forget to thank them for their continued support of this show!
Modern data teams are dealing with a lot of complexity in their data pipelines and analytical code. Monitoring data quality, tracing incidents, and testing changes can be daunting and often takes hours to days or even weeks. By the time errors have made their way into production, it’s often too late and damage is done. Datafold built automated regression testing to help data and analytics engineers deal with data quality in their pull requests. Datafold shows how a change in SQL code affects your data, both on a statistical level and down to individual rows and values before it gets merged to production. No more shipping and praying, you can now know exactly what will change in your database! Datafold integrates with all major data warehouses as well as frameworks such as Airflow & dbt and seamlessly plugs into CI workflows. Visit dataengineeringpodcast.com/datafold today to book a demo with Datafold.
RudderStack helps you build a customer data platform on your warehouse or data lake. Instead of trapping data in a black box, they enable you to easily collect customer data from the entire stack and build an identity graph on your warehouse, giving you full visibility and control. Their SDKs make event streaming from any app or website easy, and their extensive library of integrations enable you to automatically send data to hundreds of downstream tools. Sign up free at dataengineeringpodcast.com/rudder
Build Data Pipelines. Not DAGs. That’s the spirit behind Upsolver SQLake, a new self-service data pipeline platform that lets you build batch and streaming pipelines without falling into the black hole of DAG-based orchestration. All you do is write a query in SQL to declare your transformation, and SQLake will turn it into a continuous pipeline that scales to petabytes and delivers up to the minute fresh data. SQLake supports a broad set of transformations, including high-cardinality joins, aggregations, upserts and window operations. Output data can be streamed into a data lake for query engines like Presto, Trino or Spark SQL, a data warehouse like Snowflake or Redshift., or any other destination you choose. Pricing for SQLake is simple. You pay $99 per terabyte ingested into your data lake using SQLake, and run unlimited transformation pipelines for free. That way data engineers and data users can process to their heart’s content without worrying about their cloud bill. For data engineering podcast listeners, we’re offering a 30 day trial with unlimited data, so go to dataengineeringpodcast.com/upsolver today and see for yourself how to avoid DAG hell.
Your host is Tobias Macey and today I’m interviewing Arjun Narayan about the benefits of real-time data for teams of all sizes
Interview
Introduction
How did you get involved in the area of data management?
Can you describe what your conception of real-time data is and the benefits that it can provide?
types of organizations/teams who are adopting real-time
consumers of real-time data
locations in data/application stacks where real-time needs to be integrated
challenges (technical/infrastructure/talent) involved in adopting/supporting streaming/real-time
lessons learned working with early customers that influenced design/implementation of Materialize to simplify adoption of real-time
types of queries that are run on materialize vs. warehouse
how real-time changes the way stakeholders think about the data
sourcing real-time data
What are the most interesting, innovative, or unexpected ways that you have seen real-time data used?
What are the most interesting, unexpected, or challenging lessons that you have learned while working on Materialize to support real-time data applications?
When is real-time the wrong choice?
What do you have planned for the future of Materialize and real-time data?
Contact Info
@narayanarjun on Twitter
Email
LinkedIn
Parting Question
From your perspective, what is the biggest gap in the tooling or technology for data management today?
Closing Announcements
Thank you for listening! Don’t forget to check out our other shows. Podcast.__init__ covers the Python language, its community, and the innovative ways it is being used. The Machine Learning Podcast helps you go from idea to production with machine learning.
Visit the site to subscribe to the show, sign up for the mailing list, and read the show notes.
If you’ve learned something or tried out a project from the show then tell us about it! Email hosts@dataengineeringpodcast.com) with your story.
To help other people find the show please leave a review on Apple Podcasts and tell your friends and co-workers
Links
Materialize
Podcast Episode
Cockroach Labs
Podcast Episode
SQL
Kafka
Debezium
Podcast Episode
Change Data Capture
Reverse ETL
Pulsar
Podcast Episode
The intro and outro music is from The Hug by The Freak Fandango Orchestra / CC BY-SA
Support Data Engineering Podcast

Nov 28, 2022 • 50min
Supporting And Expanding The Arrow Ecosystem For Fast And Efficient Data Processing At Voltron Data
Summary
The data ecosystem has been growing rapidly, with new communities joining and bringing their preferred programming languages to the mix. This has led to inefficiencies in how data is stored, accessed, and shared across process and system boundaries. The Arrow project is designed to eliminate wasted effort in translating between languages, and Voltron Data was created to help grow and support its technology and community. In this episode Wes McKinney shares the ways that Arrow and its related projects are improving the efficiency of data systems and driving their next stage of evolution.
Announcements
Hello and welcome to the Data Engineering Podcast, the show about modern data management
When you’re ready to build your next pipeline, or want to test out the projects you hear about on the show, you’ll need somewhere to deploy it, so check out our friends at Linode. With their new managed database service you can launch a production ready MySQL, Postgres, or MongoDB cluster in minutes, with automated backups, 40 Gbps connections from your application hosts, and high throughput SSDs. Go to dataengineeringpodcast.com/linode today and get a $100 credit to launch a database, create a Kubernetes cluster, or take advantage of all of their other services. And don’t forget to thank them for their continued support of this show!
Atlan is the metadata hub for your data ecosystem. Instead of locking your metadata into a new silo, unleash its transformative potential with Atlan’s active metadata capabilities. Push information about data freshness and quality to your business intelligence, automatically scale up and down your warehouse based on usage patterns, and let the bots answer those questions in Slack so that the humans can focus on delivering real value. Go to dataengineeringpodcast.com/atlan today to learn more about how Atlan’s active metadata platform is helping pioneering data teams like Postman, Plaid, WeWork & Unilever achieve extraordinary things with metadata and escape the chaos.
Struggling with broken pipelines? Stale dashboards? Missing data? If this resonates with you, you’re not alone. Data engineers struggling with unreliable data need look no further than Monte Carlo, the leading end-to-end Data Observability Platform! Trusted by the data teams at Fox, JetBlue, and PagerDuty, Monte Carlo solves the costly problem of broken data pipelines. Monte Carlo monitors and alerts for data issues across your data warehouses, data lakes, dbt models, Airflow jobs, and business intelligence tools, reducing time to detection and resolution from weeks to just minutes. Monte Carlo also gives you a holistic picture of data health with automatic, end-to-end lineage from ingestion to the BI layer directly out of the box. Start trusting your data with Monte Carlo today! Visit dataengineeringpodcast.com/montecarlo to learn more.
Data engineers don’t enjoy writing, maintaining, and modifying ETL pipelines all day, every day. Especially once they realize 90% of all major data sources like Google Analytics, Salesforce, Adwords, Facebook, Spreadsheets, etc., are already available as plug-and-play connectors with reliable, intuitive SaaS solutions. Hevo Data is a highly reliable and intuitive data pipeline platform used by data engineers from 40+ countries to set up and run low-latency ELT pipelines with zero maintenance. Boasting more than 150 out-of-the-box connectors that can be set up in minutes, Hevo also allows you to monitor and control your pipelines. You get: real-time data flow visibility, fail-safe mechanisms, and alerts if anything breaks; preload transformations and auto-schema mapping precisely control how data lands in your destination; models and workflows to transform data for analytics; and reverse-ETL capability to move the transformed data back to your business software to inspire timely action. All of this, plus its transparent pricing and 24*7 live support, makes it consistently voted by users as the Leader in the Data Pipeline category on review platforms like G2. Go to dataengineeringpodcast.com/hevodata and sign up for a free 14-day trial that also comes with 24×7 support.
Your host is Tobias Macey and today I’m interviewing Wes McKinney about his work at Voltron Data and on the Arrow ecosystem
Interview
Introduction
How did you get involved in the area of data management?
Can you describe what you are building at Voltron Data and the story behind it?
What is the vision for the broader data ecosystem that you are trying to realize through your investment in Arrow and related projects?
How does your work at Voltron Data contribute to the realization of that vision?
What is the impact on engineer productivity and compute efficiency that gets introduced by the impedance mismatches between language and framework representations of data?
The scope and capabilities of the Arrow project have grown substantially since it was first introduced. Can you give an overview of the current features and extensions to the project?
What are some of the ways that ArrowVe and its related projects can be integrated with or replace the different elements of a data platform?
Can you describe how Arrow is implemented?
What are the most complex/challenging aspects of the engineering needed to support interoperable data interchange between language runtimes?
How are you balancing the desire to move quickly and improve the Arrow protocol and implementations, with the need to wait for other players in the ecosystem (e.g. database engines, compute frameworks, etc.) to add support?
With the growing application of data formats such as graphs and vectors, what do you see as the role of Arrow and its ideas in those use cases?
For workflows that rely on integrating structured and unstructured data, what are the options for interaction with non-tabular data? (e.g. images, documents, etc.)
With your support-focused business model, how are you approaching marketing and customer education to make it viable and scalable?
What are the most interesting, innovative, or unexpected ways that you have seen Arrow used?
What are the most interesting, unexpected, or challenging lessons that you have learned while working on Arrow and its ecosystem?
When is Arrow the wrong choice?
What do you have planned for the future of Arrow?
Contact Info
Website
wesm on GitHub
@wesmckinn on Twitter
Parting Question
From your perspective, what is the biggest gap in the tooling or technology for data management today?
Closing Announcements
Thank you for listening! Don’t forget to check out our other shows. Podcast.__init__ covers the Python language, its community, and the innovative ways it is being used. The Machine Learning Podcast helps you go from idea to production with machine learning.
Visit the site to subscribe to the show, sign up for the mailing list, and read the show notes.
If you’ve learned something or tried out a project from the show then tell us about it! Email hosts@dataengineeringpodcast.com) with your story.
To help other people find the show please leave a review on Apple Podcasts and tell your friends and co-workers
Links
Voltron Data
Pandas
Podcast Episode
Apache Arrow
Partial Differential Equation
FPGA == Field-Programmable Gate Array
GPU == Graphics Processing Unit
Ursa Labs
Voltron (cartoon)
Feature Engineering
PySpark
Substrait
Arrow Flight
Acero
Arrow Datafusion
Velox
Ibis
SIMD == Single Instruction, Multiple Data
Lance
DuckDB
Podcast Episode
Data Threads Conference
Nano-Arrow
Arrow ADBC Protocol
Apache Iceberg
Podcast Episode
The intro and outro music is from The Hug by The Freak Fandango Orchestra / CC BY-SA
Sponsored By:Atlan: 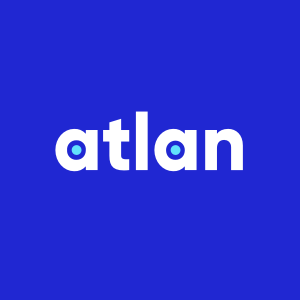
Have you ever woken up to a crisis because a number on a dashboard is broken and no one knows why? Or sent out frustrating slack messages trying to find the right data set? Or tried to understand what a column name means?
Our friends at Atlan started out as a data team themselves and faced all this collaboration chaos themselves, and started building Atlan as an internal tool for themselves. Atlan is a collaborative workspace for data-driven teams, like Github for engineering or Figma for design teams. By acting as a virtual hub for data assets ranging from tables and dashboards to SQL snippets & code, Atlan enables teams to create a single source of truth for all their data assets, and collaborate across the modern data stack through deep integrations with tools like Snowflake, Slack, Looker and more.
Go to [dataengineeringpodcast.com/atlan](https://www.dataengineeringpodcast.com/atlan) and sign up for a free trial. If you’re a data engineering podcast listener, you get credits worth $3000 on an annual subscription.

Nov 28, 2022 • 59min
Analyze Massive Data At Interactive Speeds With The Power Of Bitmaps Using FeatureBase
Summary
The most expensive part of working with massive data sets is the work of retrieving and processing the files that contain the raw information. FeatureBase (formerly Pilosa) avoids that overhead by converting the data into bitmaps. In this episode Matt Jaffee explains how to model your data as bitmaps and the benefits that this representation provides for fast aggregate computation. He also discusses the improvements that have been incorporated into FeatureBase to simplify integration with the rest of your data stack, and the SQL interface that was added to make working with the product easier.
Announcements
Hello and welcome to the Data Engineering Podcast, the show about modern data management
When you’re ready to build your next pipeline, or want to test out the projects you hear about on the show, you’ll need somewhere to deploy it, so check out our friends at Linode. With their new managed database service you can launch a production ready MySQL, Postgres, or MongoDB cluster in minutes, with automated backups, 40 Gbps connections from your application hosts, and high throughput SSDs. Go to dataengineeringpodcast.com/linode today and get a $100 credit to launch a database, create a Kubernetes cluster, or take advantage of all of their other services. And don’t forget to thank them for their continued support of this show!
Modern data teams are dealing with a lot of complexity in their data pipelines and analytical code. Monitoring data quality, tracing incidents, and testing changes can be daunting and often takes hours to days or even weeks. By the time errors have made their way into production, it’s often too late and damage is done. Datafold built automated regression testing to help data and analytics engineers deal with data quality in their pull requests. Datafold shows how a change in SQL code affects your data, both on a statistical level and down to individual rows and values before it gets merged to production. No more shipping and praying, you can now know exactly what will change in your database! Datafold integrates with all major data warehouses as well as frameworks such as Airflow & dbt and seamlessly plugs into CI workflows. Visit dataengineeringpodcast.com/datafold today to book a demo with Datafold.
RudderStack helps you build a customer data platform on your warehouse or data lake. Instead of trapping data in a black box, they enable you to easily collect customer data from the entire stack and build an identity graph on your warehouse, giving you full visibility and control. Their SDKs make event streaming from any app or website easy, and their extensive library of integrations enable you to automatically send data to hundreds of downstream tools. Sign up free at dataengineeringpodcast.com/rudder
Build Data Pipelines. Not DAGs. That’s the spirit behind Upsolver SQLake, a new self-service data pipeline platform that lets you build batch and streaming pipelines without falling into the black hole of DAG-based orchestration. All you do is write a query in SQL to declare your transformation, and SQLake will turn it into a continuous pipeline that scales to petabytes and delivers up to the minute fresh data. SQLake supports a broad set of transformations, including high-cardinality joins, aggregations, upserts and window operations. Output data can be streamed into a data lake for query engines like Presto, Trino or Spark SQL, a data warehouse like Snowflake or Redshift., or any other destination you choose. Pricing for SQLake is simple. You pay $99 per terabyte ingested into your data lake using SQLake, and run unlimited transformation pipelines for free. That way data engineers and data users can process to their heart’s content without worrying about their cloud bill. For data engineering podcast listeners, we’re offering a 30 day trial with unlimited data, so go to dataengineeringpodcast.com/upsolver today and see for yourself how to avoid DAG hell.
Your host is Tobias Macey and today I’m interviewing Matt Jaffee about FeatureBase (formerly known as Pilosa and Molecula), a real-time analytical database engine built on bitmaps
Interview
Introduction
How did you get involved in the area of data management?
Can you describe what FeatureBase is?
What are the use cases that it is designed and optimized for?
What are some applications or analyses that are uniquely suited to FeatureBase’s capabilities?
What are the notable changes/evolutions that it has gone through in recent years?
What are the forces in the broader data ecosystem that have had the greatest impact on your project/product focus?
What are the data modeling concepts that platform and data engineers need to consider when working with FeatureBase?
With bitmaps as the core data structure, what is involved in translating existing data into bitmaps?
How does schema evolution translate to the data representation used in FeatureBase?
How does the data model influence considerations around security policies and governance?
What are the most interesting, innovative, or unexpected ways that you have seen FeatureBase used?
What are the most interesting, unexpected, or challenging lessons that you have learned while working on FeatureBase?
When is FeatureBase the wrong choice?
What do you have planned for the future of FeatureBase?
Contact Info
LinkedIn
jaffee on GitHub
@mattjaffee on Twitter
Parting Question
From your perspective, what is the biggest gap in the tooling or technology for data management today?
Closing Announcements
Thank you for listening! Don’t forget to check out our other shows. Podcast.__init__ covers the Python language, its community, and the innovative ways it is being used. The Machine Learning Podcast helps you go from idea to production with machine learning.
Visit the site to subscribe to the show, sign up for the mailing list, and read the show notes.
If you’ve learned something or tried out a project from the show then tell us about it! Email hosts@dataengineeringpodcast.com) with your story.
To help other people find the show please leave a review on Apple Podcasts and tell your friends and co-workers
Links
FeatureBase
Pilosa Episode
Molecula Episode
Bitmap
Roaring Bitmaps
Pinecone
Podcast Episode
Milvus
Podcast Episode
The intro and outro music is from The Hug by The Freak Fandango Orchestra / CC BY-SA
Sponsored By:Rudderstack: 
RudderStack provides all your customer data pipelines in one platform. You can collect, transform, and route data across your entire stack with its event streaming, ETL, and reverse ETL pipelines.
RudderStack’s warehouse-first approach means it does not store sensitive information, and it allows you to leverage your existing data warehouse/data lake infrastructure to build a single source of truth for every team.
RudderStack also supports real-time use cases. You can Implement RudderStack SDKs once, then automatically send events to your warehouse and 150+ business tools, and you’ll never have to worry about API changes again.
Visit [dataengineeringpodcast.com/rudderstack](https://www.dataengineeringpodcast.com/rudderstack) to sign up for free today, and snag a free T-Shirt just for being a Data Engineering Podcast listener.Support Data Engineering Podcast

Nov 21, 2022 • 47min
Tame The Entropy In Your Data Stack And Prevent Failures With Sifflet
Summary
The problems that are easiest to fix are the ones that you prevent from happening in the first place. Sifflet is a platform that brings your entire data stack into focus to improve the reliability of your data assets and empower collaboration across your teams. In this episode CEO and founder Salma Bakouk shares her views on the causes and impacts of "data entropy" and how you can tame it before it leads to failures.
Announcements
Hello and welcome to the Data Engineering Podcast, the show about modern data management
When you’re ready to build your next pipeline, or want to test out the projects you hear about on the show, you’ll need somewhere to deploy it, so check out our friends at Linode. With their new managed database service you can launch a production ready MySQL, Postgres, or MongoDB cluster in minutes, with automated backups, 40 Gbps connections from your application hosts, and high throughput SSDs. Go to dataengineeringpodcast.com/linode today and get a $100 credit to launch a database, create a Kubernetes cluster, or take advantage of all of their other services. And don’t forget to thank them for their continued support of this show!
Modern data teams are dealing with a lot of complexity in their data pipelines and analytical code. Monitoring data quality, tracing incidents, and testing changes can be daunting and often takes hours to days or even weeks. By the time errors have made their way into production, it’s often too late and damage is done. Datafold built automated regression testing to help data and analytics engineers deal with data quality in their pull requests. Datafold shows how a change in SQL code affects your data, both on a statistical level and down to individual rows and values before it gets merged to production. No more shipping and praying, you can now know exactly what will change in your database! Datafold integrates with all major data warehouses as well as frameworks such as Airflow & dbt and seamlessly plugs into CI workflows. Visit dataengineeringpodcast.com/datafold today to book a demo with Datafold.
RudderStack helps you build a customer data platform on your warehouse or data lake. Instead of trapping data in a black box, they enable you to easily collect customer data from the entire stack and build an identity graph on your warehouse, giving you full visibility and control. Their SDKs make event streaming from any app or website easy, and their extensive library of integrations enable you to automatically send data to hundreds of downstream tools. Sign up free at dataengineeringpodcast.com/rudder
Data teams are increasingly under pressure to deliver. According to a recent survey by Ascend.io, 95% in fact reported being at or over capacity. With 72% of data experts reporting demands on their team going up faster than they can hire, it’s no surprise they are increasingly turning to automation. In fact, while only 3.5% report having current investments in automation, 85% of data teams plan on investing in automation in the next 12 months. 85%!!! That’s where our friends at Ascend.io come in. The Ascend Data Automation Cloud provides a unified platform for data ingestion, transformation, orchestration, and observability. Ascend users love its declarative pipelines, powerful SDK, elegant UI, and extensible plug-in architecture, as well as its support for Python, SQL, Scala, and Java. Ascend automates workloads on Snowflake, Databricks, BigQuery, and open source Spark, and can be deployed in AWS, Azure, or GCP. Go to dataengineeringpodcast.com/ascend and sign up for a free trial. If you’re a data engineering podcast listener, you get credits worth $5,000 when you become a customer.
Your host is Tobias Macey and today I’m interviewing Salma Bakouk about achieving data reliability and reducing entropy within your data stack with sifflet
Interview
Introduction
How did you get involved in the area of data management?
Can you describe what Sifflet is and the story behind it?
What is the motivating goal for the company and product?
What are the categories of errors that you consider to be preventable?
How does the visibility provided by Sifflet contribute to those prevention efforts?
What are the UI/UX patterns that you rely on to allow for meaningful exploration and analysis of dependency chains/impact assessments in the lineage graph?
Can you describe how you’ve implemented Sifflet?
How have the scope and focus of the product evolved from when you first launched?
What is the workflow for someone getting Sifflet integrated into their data stack?
What are some of the data modeling considerations that need to be considered when pushing metadata to Sifflet?
What are the most interesting, innovative, or unexpected ways that you have seen Sifflet used?
What are the most interesting, unexpected, or challenging lessons that you have learned while working on Sifflet?
When is Sifflet the wrong choice?
What do you have planned for the future of Sifflet?
Contact Info
LinkedIn
@SalmaBakouk on Twitter
Parting Question
From your perspective, what is the biggest gap in the tooling or technology for data management today?
Closing Announcements
Thank you for listening! Don’t forget to check out our other shows. Podcast.__init__ covers the Python language, its community, and the innovative ways it is being used. The Machine Learning Podcast helps you go from idea to production with machine learning.
Visit the site to subscribe to the show, sign up for the mailing list, and read the show notes.
If you’ve learned something or tried out a project from the show then tell us about it! Email hosts@dataengineeringpodcast.com) with your story.
To help other people find the show please leave a review on Apple Podcasts and tell your friends and co-workers
Links
Sifflet
Data Observability
DataDog
NewRelic
Splunk
Modern Data Stack
GoCardless
Airbyte
Fivetran
ORM == Object Relational Mapping
The intro and outro music is from The Hug by The Freak Fandango Orchestra / CC BY-SA
Sponsored By:Ascend: 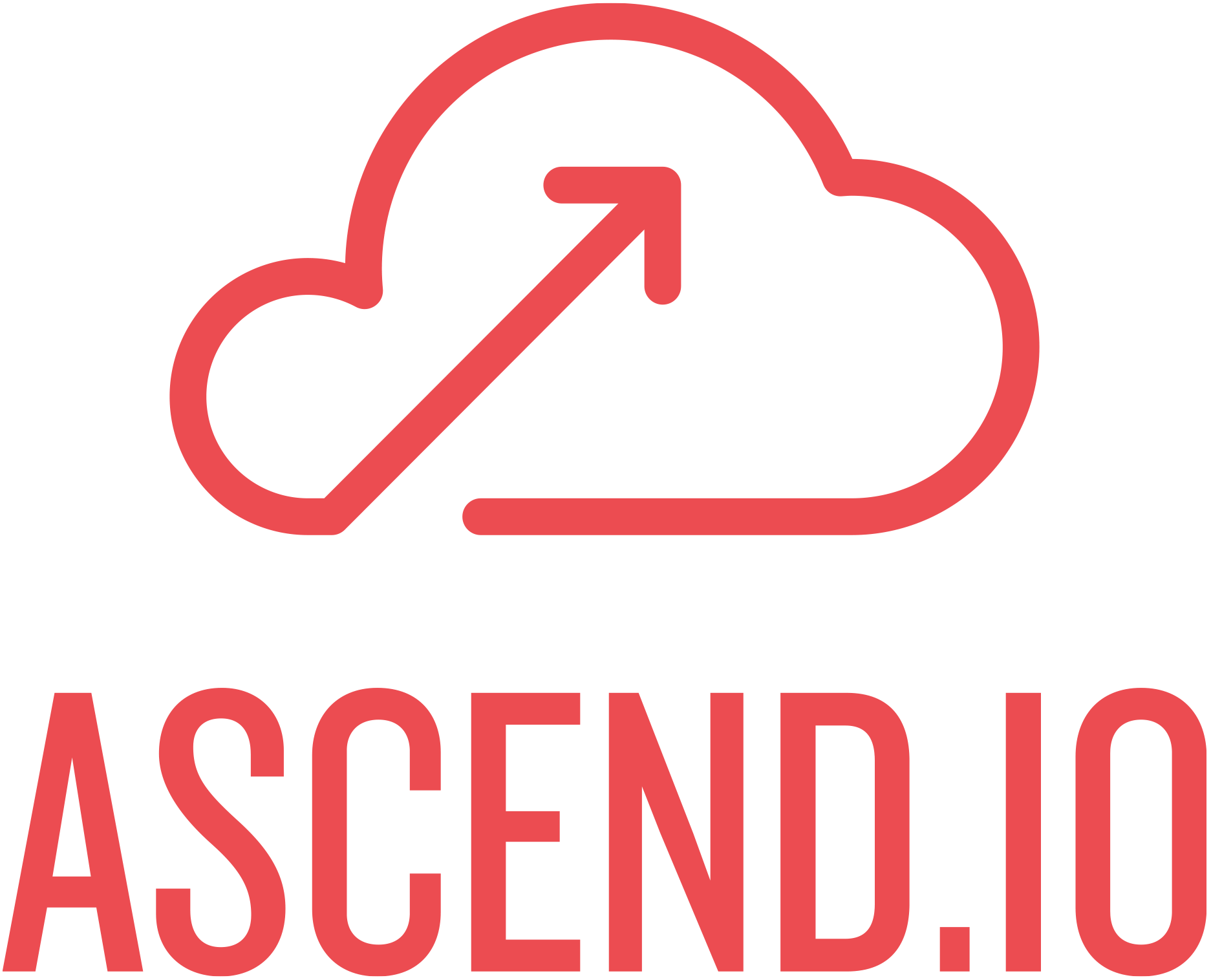
Ascend.io, the Data Automation Cloud, provides the most advanced automation for data and analytics engineering workloads. Ascend.io unifies the core capabilities of data engineering—data ingestion, transformation, delivery, orchestration, and observability—into a single platform so that data teams deliver 10x faster. With 95% of data teams already at or over capacity, engineering productivity is a top priority for enterprises. Ascend’s Flex-code user interface empowers any member of the data team—from data engineers to data scientists to data analysts—to quickly and easily build and deliver on the data and analytics workloads they need. And with Ascend’s DataAware™ intelligence, data teams no longer spend hours carefully orchestrating brittle data workloads and instead rely on advanced automation to optimize the entire data lifecycle. Ascend.io runs natively on data lakes and warehouses and in AWS, Google Cloud and Microsoft Azure.
Go to [dataengineeringpodcast.com/ascend](https://www.dataengineeringpodcast.com/ascend)
to find out more.Support Data Engineering Podcast

Nov 21, 2022 • 1h 1min
A Look At The Data Systems Behind The Gameplay For League Of Legends
In this podcast, Ian Schweer shares his experiences at Riot Games in supporting player-focused features in League of Legends. He discusses the challenges of building useful data products on top of a legacy platform and the constraints his team faces. The podcast also explores data management and collaboration in game development, as well as the data systems architecture for League of Legends gameplay. Additionally, the speakers discuss reducing onboarding overhead and challenges in data platforms and systems, lessons learned, and the need for improved tools in data management.
Remember Everything You Learn from Podcasts
Save insights instantly, chat with episodes, and build lasting knowledge - all powered by AI.