
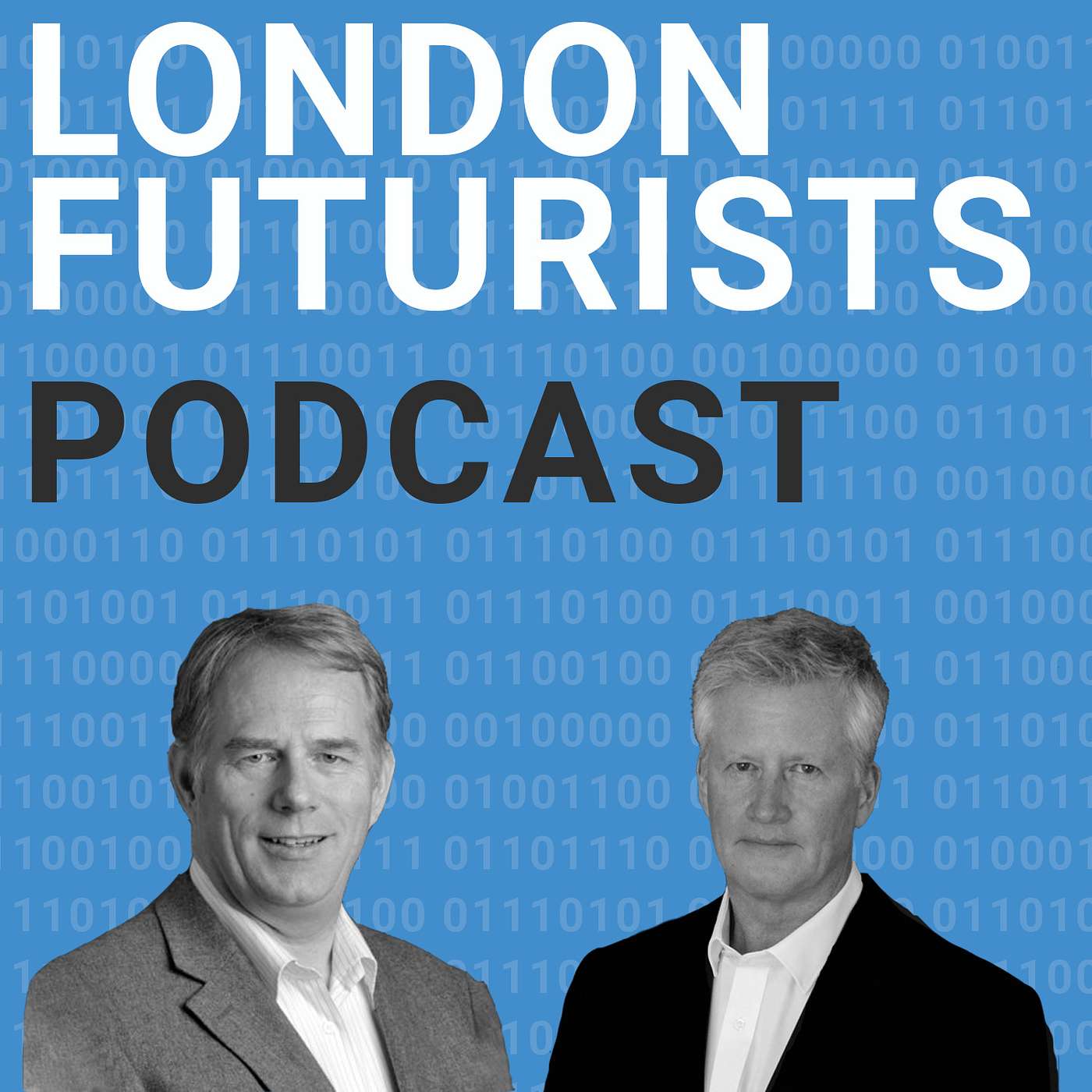
London Futurists
London Futurists
Anticipating and managing exponential impact - hosts David Wood and Calum ChaceCalum Chace is a sought-after keynote speaker and best-selling writer on artificial intelligence. He focuses on the medium- and long-term impact of AI on all of us, our societies and our economies. He advises companies and governments on AI policy.His non-fiction books on AI are Surviving AI, about superintelligence, and The Economic Singularity, about the future of jobs. Both are now in their third editions.He also wrote Pandora's Brain and Pandora’s Oracle, a pair of techno-thrillers about the first superintelligence. He is a regular contributor to magazines, newspapers, and radio.In the last decade, Calum has given over 150 talks in 20 countries on six continents. Videos of his talks, and lots of other materials are available at https://calumchace.com/.He is co-founder of a think tank focused on the future of jobs, called the Economic Singularity Foundation. The Foundation has published Stories from 2045, a collection of short stories written by its members.Before becoming a full-time writer and speaker, Calum had a 30-year career in journalism and in business, as a marketer, a strategy consultant and a CEO. He studied philosophy, politics, and economics at Oxford University, which confirmed his suspicion that science fiction is actually philosophy in fancy dress.David Wood is Chair of London Futurists, and is the author or lead editor of twelve books about the future, including The Singularity Principles, Vital Foresight, The Abolition of Aging, Smartphones and Beyond, and Sustainable Superabundance.He is also principal of the independent futurist consultancy and publisher Delta Wisdom, executive director of the Longevity Escape Velocity (LEV) Foundation, Foresight Advisor at SingularityNET, and a board director at the IEET (Institute for Ethics and Emerging Technologies). He regularly gives keynote talks around the world on how to prepare for radical disruption. See https://deltawisdom.com/.As a pioneer of the mobile computing and smartphone industry, he co-founded Symbian in 1998. By 2012, software written by his teams had been included as the operating system on 500 million smartphones.From 2010 to 2013, he was Technology Planning Lead (CTO) of Accenture Mobility, where he also co-led Accenture’s Mobility Health business initiative.Has an MA in Mathematics from Cambridge, where he also undertook doctoral research in the Philosophy of Science, and a DSc from the University of Westminster.
Episodes
Mentioned books

Dec 21, 2022 • 34min
Governing the transition to AGI, with Jerome Glenn
Our guest on this episode is someone with excellent connections to the foresight departments of governments around the world. He is Jerome Glenn, Founder and Executive Director of the Millennium Project.The Millennium Project is a global participatory think tank established in 1996, which now has over 70 nodes around the world. It has the stated purpose to "Improve humanity's prospects for building a better world". The organisation produces regular "State of the Future" reports as well as updates on what it describes as "the 15 Global Challenges". It recently released an acclaimed report on three scenarios for the future of work. One of its new projects is the main topic in this episode, namely scenarios for the global governance of the transition from Artificial Narrow Intelligence (ANI) to Artificial General Intelligence (AGI).Topics discussed in this episode include:*) Why many futurists are jealous of Alvin Toffler*) The benefits of a decentralised, incremental approach to foresight studies*) Special features of the Millennium Project compared to other think tanks*) How the Information Revolution differs from the Industrial Revolution*) What is likely to happen if there is no governance of the transition to AGI*) Comparisons with regulating the use of cars - and the use of nuclear materials*) Options for licensing, auditing, and monitoring*) How the development of a technology may be governed even if it has few visible signs*) Three options: "Hope", "Control", and "Merge" - but all face problems; in all three cases, getting the initial conditions right could make a huge difference*) Distinctions between AGI and ASI (Artificial Superintelligence), and whether an ASI could act in defiance of its initial conditions*) Controlling AGI is likely to be impossible, but controlling the companies that are creating AGI is more credible*) How actions taken by the EU might influence decisions elsewhere in the world*) Options for "aligning" AGI as opposed to "controlling" it*) Complications with the use of advanced AI by organised crime and by rogue states*) The poor level of understanding of most political advisors about AGI, and their tendency to push discussions back to the issues of ANI*) Risks of catastrophic social destabilisation if "the mother of all panics" about AGI occurs on top of existing culture wars and political tribalism*) Past examples of progress with technologies that initially seemed impossible to govern*) The importance of taking some initial steps forward, rather than being overwhelmed by the scale of the challenge.Music: Spike Protein, by Koi Discovery, available under CC0 1.0 Public Domain DeclarationSelected follow-up reading:https://en.wikipedia.org/wiki/Jerome_C._Glennhttps://www.millennium-project.org/https://www.millennium-project.org/first-steps-for-artificial-general-intelligence-governance-study-have-begun/The 2020 book "After Shock: The World's Foremost Futurists Reflect on 50 Years of Future Shock - and Look Ahead to the Next 50"Digital Disruption with Geoff Nielson Discover how technology is reshaping our lives and livelihoods.Listen on: Apple Podcasts Spotify

Dec 14, 2022 • 30min
Introducing Decision Intelligence, with Steven Coates
This episode features the CEO of Brainnwave, Steven Coates, who is a pioneer in the field of Decision Intelligence.Decision Intelligence is the use of AI to enhance the ability of companies, organisations, or individuals to make key decisions - decisions about which new business opportunities to pursue, about evidence of possible leakage or waste, about the allocation of personnel to tasks, about geographical areas to target, and so on.What these decisions have in common is that they can all be improved by the analysis of large sets of data that defy attempts to reduce them to a single dimension. In these cases, AI systems that are suited to multi-dimensional analysis can make all the difference between wise and unwise decisions.Topics discussed in this episode include:*) The ideas initially pursued at Brainnwave, and how they evolved over time*) Real-world examples of Decision Intelligence - in the mining industry, the supply of mobile power generators, and in the oil industry*) Recommendations for businesses to focus on Decision Intelligence as they adopt fuller use of AI, on account of the direct impact on business outcomes*) Factors holding up the wider adoption of AI*) Challenges when "data lakes" turn into "data swamps"*) Challenges with the limits of trust that can be placed in data*) Challenges with the lack of trust in algorithms*) Skills in explaining how algorithms are reaching their decisions*) The benefits of an agile mindset in introducing Decision Intelligence.Music: Spike Protein, by Koi Discovery, available under CC0 1.0 Public Domain DeclarationSome follow-up reading:https://brainnwave.ai/Digital Disruption with Geoff Nielson Discover how technology is reshaping our lives and livelihoods.Listen on: Apple Podcasts Spotify

Dec 7, 2022 • 32min
Developing responsible AI, with Ray Eitel-Porter
As AI automates larger portions of the activities of companies and organisations, there's a greater need to think carefully about questions of privacy, bias, transparency, and explainability. Due to scale effects, mistakes made by AI and the automated analysis of data can have wide impacts. On the other hand, evidence of effective governance of AI development can deepen trust and accelerate the adoption of significant innovations.One person who has thought a great deal about these issues is Ray Eitel-Porter, Global Lead for Responsible AI at Accenture. In this episode of the London Futurist Podcast, he explains what conclusions he has reached.Topics discussed include:*) The meaning and importance of "Responsible AI"*) Connections and contrasts with "AI ethics" and "AI safety"*) The advantages of formal AI governance processes*) Recommendations for the operation of an AI ethics board*) Anticipating the operation of the EU's AI Act*) How different intuitions of fairness can produce divergent results*) Examples where transparency has been limited*) The potential future evolution of the discipline of Responsible AI.Music: Spike Protein, by Koi Discovery, available under CC0 1.0 Public Domain DeclarationSome follow-up reading:https://www.accenture.com/gb-en/services/applied-intelligence/ai-ethics-governanceDigital Disruption with Geoff Nielson Discover how technology is reshaping our lives and livelihoods.Listen on: Apple Podcasts Spotify

Nov 30, 2022 • 31min
Anticipating Longevity Escape Velocity, with Aubrey de Grey
One area of technology that is frequently in the news these days is rejuvenation biotechnology, namely the possibility of undoing key aspects of biological aging via a suite of medical interventions. What these interventions target isn't individual diseases, such as cancer, stroke, or heart disease, but rather the common aggravating factors that lie behind the increasing prevalence of these diseases as we become older.Our guest in this episode is someone who has been at the forefront for over 20 years of a series of breakthrough initiatives in this field of rejuvenation biotechnology. He is Dr Aubrey de Grey, co-founder of the Methuselah Foundation, the SENS Research Foundation, and, most recently, the LEV Foundation - where 'LEV' stands for Longevity Escape Velocity.Topics discussed include:*) Different concepts of aging and damage repair;*) Why the outlook for damage repair is significantly more tangible today than it was ten years ago;*) The role of foundations in supporting projects which cannot receive funding from commercial ventures;*) Questions of pace of development: cautious versus bold;*) Changing timescales for the likely attainment of robust mouse rejuvenation ('RMR') and longevity escape velocity ('LEV');*) The "Less Death" initiative;*) "Anticipating anticipation" - preparing for likely sweeping changes in public attitude once understanding spreads about the forthcoming available of powerful rejuvenation treatments;*) Various advocacy initiatives that Aubrey is supporting;*) Ways in which listeners can help to accelerate the attainment of LEV.Music: Spike Protein, by Koi Discovery, available under CC0 1.0 Public Domain DeclarationSome follow-up reading:https://levf.orghttps://lessdeath.orgDigital Disruption with Geoff Nielson Discover how technology is reshaping our lives and livelihoods.Listen on: Apple Podcasts Spotify

Nov 23, 2022 • 33min
Expanding humanity's moral circle, with Jacy Reese Anthis
A Venn diagram of people interested in how AI will shape our future, and members of the effective altruism community (often abbreviated to EA), would show a lot of overlap. One of the rising stars in this overlap is our guest in this episode, the polymath Jacy Reese Anthis.Our discussion picks up themes from Jacy's 2018 book “The End of Animal Farming”, including an optimistic roadmap toward an animal-free food system, as well as factors that could alter that roadmap.We also hear about the work of an organisation co-founded by Jacy: the Sentience Institute, which researches - among other topics - the expansion of moral considerations to non-human entities. We discuss whether AIs can be sentient, how we might know if an AI is sentient, and whether the design choices made by developers of AI will influence the degree and type of sentience of AIs.The conversation concludes with some ideas about how various techniques can be used to boost personal effectiveness, and considers different ways in which people can relate to the EA community.Music: Spike Protein, by Koi Discovery, available under CC0 1.0 Public Domain DeclarationSome follow-up reading:https://www.sentienceinstitute.org/https://jacyanthis.com/Digital Disruption with Geoff Nielson Discover how technology is reshaping our lives and livelihoods.Listen on: Apple Podcasts Spotify

Nov 16, 2022 • 30min
Hacking the simulation, with Roman Yampolskiy
In the 4th century BC, the Greek philosopher Plato theorised that humans do not perceive the world as it really is. All we can see is shadows on a wall.In 2003, the Swedish philosopher Nick Bostrom published a paper which formalised an argument to prove Plato was right. The paper argued that one of the following three statements is true:1. We will go extinct fairly soon2. Advanced civilisations don’t produce simulations containing entities which think they are naturally-occurring sentient intelligences. (This could be because it is impossible.)3. We are in a simulation.The reason for this is that if it is possible, and civilisations can become advanced without exploding, then there will be vast numbers of simulations, and it is vanishingly unlikely that any randomly selected civilisation (like us) is a naturally-occurring one.Some people find this argument pretty convincing. As we will hear later, some of us have added twists to the argument. But some people go even further, and speculate about how we might bust out of the simulation.One such person is our friend and our guest in this episode, Roman Yampolskiy, Professor of Computer Science at the University of Louisville.Music: Spike Protein, by Koi Discovery, available under CC0 1.0 Public Domain DeclarationFurther reading:"How to Hack the Simulation" by Roman Yampolskiy: https://www.researchgate.net/publication/364811408_How_to_Hack_the_Simulation"The Simulation Argument" by Nick Bostrom: https://www.simulation-argument.com/Digital Disruption with Geoff Nielson Discover how technology is reshaping our lives and livelihoods.Listen on: Apple Podcasts Spotify

Nov 9, 2022 • 40min
Pioneering AI drug development, with Alex Zhavoronkov
This episode discusses progress at Insilico Medicine, the AI drug development company founded by our guest, longevity pioneer Alex Zhavoronkov.1.20 In Feb 2022, Insilico got an IPF drug into phase 1 clinical trials: a first for a wholly AI-developed drug1.50 Insilico is now well-funded; its software is widely used in the pharma industry2.30 How drug development works. First you create a hypothesis about what causes a disease4.00 Pandaomics is Insilico’s software to generate hypotheses. It combines 20+ AI models, and huge public data repositories6.00 This first phase is usually done in academia. It usually costs $ billions to develop a hypothesis. 95% of them fail6.50 The second phase is developing a molecule which might treat the disease7.15 This is the job of Insilico’s Chemistry 42 platform7.30 The classical approach is to test thousands of molecules to see if they bind to the target protein7.50 AI, by contrast, is able to "imagine" a novel molecule which might bind to it8.00 You then test 10-15 molecules which have the desired characteristics8.20 This is done with a variety of genetic algorithms, Generative Adversarial Networks (GANs), and some Transformer networks8.35 Insilico has a “zoo” of 40 validated models10.40 Given the ten-fold improvement, why hasn’t the whole drug industry adopted this process?10.50 They do all have AI groups and they are trying to change, but they are huge companies, and it takes time11.50 Is it better to invent new molecules, or re-purpose old drugs, which are already known to be safe in humans?13.00 You can’t gain IP with re-purposed drugs: either somebody else “owns” them, or they are already generic15.00 The IPF drug was identified during aging research, using aging clocks, and a deep neural net trained on longitudinal data17.10 The third phase is where Insilico’s other platform, InClinico, comes into play17.35 InClinico predicts the results of phase 2 (clinical efficacy) trials18.15 InClinico is trained on massive data sets about previous trials19.40 InClinico is actually Insilico’s oldest system. Its value has only been ascertained now that some drugs have made it all the way through the pipeline22.05 A major pharma company asked Insilico to predict the outcome of ten of its trials22.30 Nine of these ten trials were predicted correctly23.00 But the company decided that adopting this methodology would be too much of an upheaval; it was unwilling to rely on outsiders so heavily24.15 Hedge funds and banks have no such qualms24.25 Insilico is doing pilots for their investments in biotech startups26.30 Alex is from Latvia originally, studied in Canada, started his career in the US, but Insilico was established in Hong Kong. Why?27.00 Chinese CROs, Contract Research Organisations, enable you to do research without having your own wetlab 28.00 Like Apple, Insilico designs in the US and does operations in China. You can also do clinical studies there28.45 They needed their own people inside those CROs, so hadDigital Disruption with Geoff Nielson Discover how technology is reshaping our lives and livelihoods.Listen on: Apple Podcasts Spotify Inspiring Tech Leaders - The Technology PodcastInterviews with Tech Leaders and insights on the latest emerging technology trends.Listen on: Apple Podcasts Spotify

Nov 2, 2022 • 31min
The Singularity Principles
Co-hosts Calum and David dig deep into aspects of David's recent new book "The Singularity Principles". Calum (CC) says he is, in part, unconvinced. David (DW) agrees that the projects he recommends are hard, but suggests some practical ways forward.0.25 The technological singularity may be nearer than we think1.10 Confusions about the singularity1.35 “Taking back control of the singularity”2.40 The “Singularity Shadow”: over-confident predictions which repulse people3.30 The over-confidence includes predictions of timescale…4.00 … and outcomes4.45 The Singularity as the Rapture of the Nerds?5.20 The Singularity is not a religion …5.40 .. although if positive, it will confer almost godlike powers6.35 Much discussion of the Singularity is dystopian, but there could be enormous benefits, including…7.15 Digital twins for cells and whole bodies, and super longevity7.30 A new enlightenment7.50 Nuclear fusion8.10 Humanity’s superpower is intelligence8.30 Amplifying our intelligence should increase our power9.50 DW’s timeline: 50% chance of AGI by 2050, 10% by 203010.10 The timeline is contingent on human actions10.40 Even if AGI isn’t coming until 2070, we should be working on AI alignment today11.10 AI Impact’s survey of all contributors to NeurIPS11.35 Median view: 50% chance of AGI in 2059, and many were pessimistic12.15 This discussion can’t be left to AI researchers12.40 A bad beta version might be our last invention13.00 A few hundred people are now working on AI alignment, and tens of thousands on advancing AI13.35 The growth of the AI research population is still faster13.40 CC: Three routes to a positive outcome13.55 1. Luck. The world turns out to be configured in our favour14.30 2. Mathematical approaches to AI alignment succeed14.45 We either align AIs forever, or manage to control them. This is very hard14.55 3. We merge with the superintelligent machines15.40 Uploading is a huge engineering challenge15.55 Philosophical issues raised by uploading: is the self retained?16.10 DW: routes 2 and 3 are too binary. A fourth route is solving morality18.15 Individual humans will be augmented, indeed we already are18.55 But augmented humans won’t necessarily be benign19.30 DW: We have to solve beneficence20.00 CC: We can’t hope to solve our moral debates before AGI arrives20.20 In which case we are relying on route 1 – luck20.30 DW: Progress in philosophy *is* possible, and must be accelerated21.15 The Universal Declaration of Human Rights shows that generalised moral principles can be agreed22.25 CC: That sounds impossible. The UDHR is very broad and often ignored23.05 Solving morality is even harder than the MIRI project, and reinforces the idea that route 3 is our best hope23.50 It’s not unreasonable to hope that wisdom correlates with intelligence24.00 DW: We can proceed step by step, starting with progress on facial recognition, autonomous weapons, and such intermediate questions25.10 CC: We are so far from solving moral questions. Americans can’t even agree if a coup against their democracy was a bad thing25.40 DW: We have to make progress, and quickly. AI might help us.26.50 The essence of transhumanism is that we can use technology to improve ourselves27.20 CC: If you had a magic wand, your first wish should probably be to make all humans see each other as members Digital Disruption with Geoff Nielson Discover how technology is reshaping our lives and livelihoods.Listen on: Apple Podcasts Spotify

Oct 26, 2022 • 36min
Collapsing AGI timelines, with Ross Nordby
How likely is it that, by 2030, someone will build artificial general intelligence (AGI)?Ross Nordby is an AI researcher who has shortened his AGI timelines: he has changed his mind about when AGI might be expected to exist. He recently published an article on the LessWrong community discussion site, giving his argument in favour of shortening these timelines. He now identifies 2030 as the date by which it is 50% likely that AGI will exist. In this episode, we ask Ross questions about his argument, and consider some of the implications that arise.Article by Ross: https://www.lesswrong.com/posts/K4urTDkBbtNuLivJx/why-i-think-strong-general-ai-is-coming-soonEffective Altruism Long-Term Future Fund: https://funds.effectivealtruism.org/funds/far-futureMIRI (Machine Intelligence Research Institution): https://intelligence.org/00.57 Ross’ background: real-time graphics, mostly in video games02.10 Increased familiarity with AI made him reconsider his AGI timeline02.37 He submitted a grant request to the Effective Altruism Long-Term Future Fund to move into AI safety work03.50 What Ross was researching: can we make an AI intrinsically interpretable?04.25 The AGI Ross is interested in is defined by capability, regardless of consciousness or sentience04.55 An AI that is itself "goalless" might be put to uses with destructive side-effects06.10 The leading AI research groups are still DeepMind and OpenAI06.43 Other groups, like Anthropic, are more interested in alignment07.22 If you can align an AI to any goal at all, that is progress: it indicates you have some control08.00 Is this not all abstract and theoretical - a distraction from more pressing problems?08.30 There are other serious problems, like pandemics and global warming, but we have to solve them all08.45 Globally, only around 300 people are focused on AI alignment: not enough10.05 AGI might well be less than three decades away10.50 AlphaGo surprised the community, which was expecting Go to be winnable 10-15 years later11.10 Then AlphaGo was surpassed by systems like AlphaZero and MuZero, which were actually simpler, and more flexible11.20 AlphaTensor frames matrix multiplication as a game, and becomes superhuman at it11.40 In 2018, the Transformer paper was published, but no-one forecast GPT-3’s capabilities12.00 This year, Minerva (similar to GPT-3) got 50% correct on the math dataset: high school competition math problems13.16 Illustrators now feel threatened by systems like Dall-E, Stable Diffusion, etc13.30 The conclusion is that intelligence is easier to simulate than we thought13.40 But these systems also do stupid things. They are brittle18.00 But we could use transformers more intelligently19.20 They turn out to be able to write code, and to explain jokes, and do maths reasoning21:10 Google's Gopher AI22.05 Machines don’t yet have internal models of the world, which we call common sense24.00 But an early version of GPT-3 demonstrated the ability to model a human thought process alongside a machine’s27.15 Ross’ current timeline is 50% probability of AGI by 2030, and 90+% by 205027:35 Counterarguments?29.35 So what is to be done?30.55 If convinced that AGI is coming soon, most lay people would probably demand that all AI research stops immediately. Which isn’t possible31.40 Maybe publicity would be good in order to generate resources for AI alignment. AnDigital Disruption with Geoff Nielson Discover how technology is reshaping our lives and livelihoods.Listen on: Apple Podcasts Spotify

Oct 19, 2022 • 33min
The terabrain is near, with Simon Thorpe
Why do human brains consume much less power than artificial neural networks? Simon Thorpe, Research Director of CNRS, explains his view that the key to artificial general intelligence is a "terabrain" that copies from human brains the sparse-firing networks with spiking neurons.00.11 Recapping "the AI paradox"00.28 The nervousness of CTOs regarding AI00.43 Introducing Simon01.43 45 years since Oxford, working out how the brain does amazing things02.45 Brain visual perception as feed-forward vs. feedback03.40 The ideas behind the system that performed so well in the 2012 ImageNet challenge04.20 The role of prompts to alter perception05.30 Drawbacks of human perceptual expectations06.05 The video of a gorilla on the basketball court06.50 Conjuring tricks and distractions07.10 Energy consumption: human neurons vs. artificial neurons07.26 The standard model would need 500 petaflops08.40 Exaflop computing has just arrived08.50 30 MW vs. 20 W (less than a lightbulb)09.34 Companies working on low-power computing systems09.48 Power requirements for edge computing10.10 The need for 86,000 neuromorphic chips?10.25 Dense activation of neurons vs. sparse activation10.58 Real brains are event driven11.16 Real neurons send spikes not floating point numbers11.55 SpikeNET by Arnaud Delorme12.50 Why are sparse networks studied so little?14.40 A recent debate with Yann LeCun of Facebook and Bill Dally of Nvidia15.40 One spike can contain many bits of information16.24 Revisiting an experiment with eels from 1927 (Lord Edgar Adrian)17.06 Biology just needs one spike17.50 Chips moved from floating point to fixed point19.25 Other mentions of sparse systems - MoE (Mixture of Experts)19.50 Sparse systems are easier to interpret20.30 Advocacy for "grandmother cells"21.23 Chicks that imprinted on yellow boots22.35 A semantic web in the 1960s22.50 The Mozart cell23.02 An expert system implemented in a neural network with spiking neurons23.14 Power consumption reduced by a factor of one million23.40 Experimental progress23.53 Dedicated silicon: Spikenet Technology, acquired by BrainChip24.18 The Terabrain Project, using standard off-the-shelf hardware24.40 Impressive recent simulations on GPUs and on a MacBook Pro26.26 A homegrown learning rule26.44 Experiments with "frozen noise"27.28 Anticipating emulating an entire human brain on a Mac Studio M1 Ultra28.25 The likely impact of these ideas29.00 This software will be given away29.17 Anticipating "local learning" without the results being sent to Big Tech30.40 GPT-3 could run on your phone next year31.12 Our interview next year might be, not with Simon, but with his Terabrain31.22 Our phones know us better than our spouses doSimon's academic page: https://cerco.cnrs.fr/page-perso-simon-thorpe/Simon's personal blog: https://simonthorpesideas.blogspot.com/Audio engineering by Alexander Chace.Music: Spike Protein, by Koi Discovery, available under CC0 1.0 Public Domain DeclarationDigital Disruption with Geoff Nielson Discover how technology is reshaping our lives and livelihoods.Listen on: Apple Podcasts Spotify