
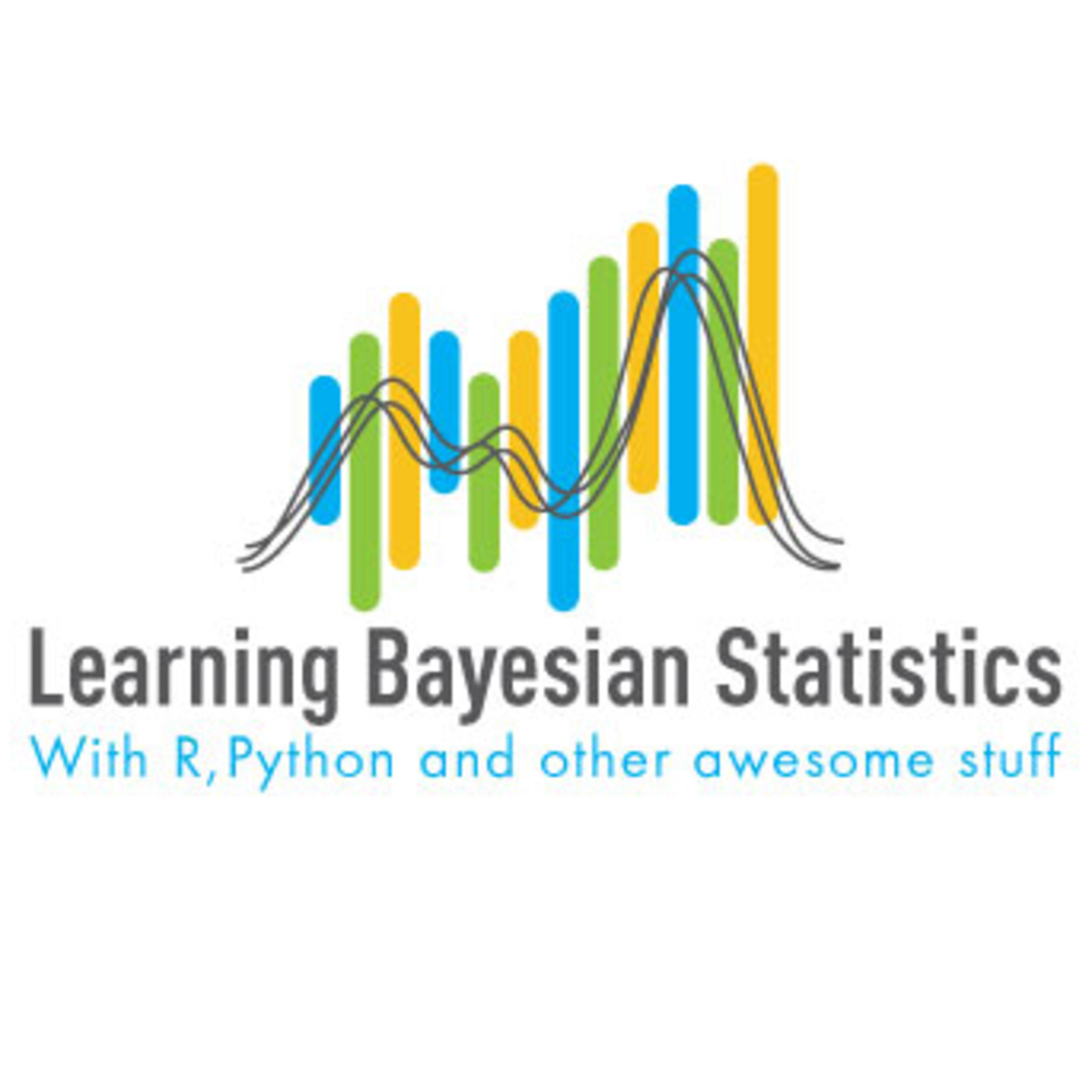
Learning Bayesian Statistics
Alexandre Andorra
Are you a researcher or data scientist / analyst / ninja? Do you want to learn Bayesian inference, stay up to date or simply want to understand what Bayesian inference is?
Then this podcast is for you! You'll hear from researchers and practitioners of all fields about how they use Bayesian statistics, and how in turn YOU can apply these methods in your modeling workflow.
When I started learning Bayesian methods, I really wished there were a podcast out there that could introduce me to the methods, the projects and the people who make all that possible.
So I created "Learning Bayesian Statistics", where you'll get to hear how Bayesian statistics are used to detect black matter in outer space, forecast elections or understand how diseases spread and can ultimately be stopped.
But this show is not only about successes -- it's also about failures, because that's how we learn best. So you'll often hear the guests talking about what *didn't* work in their projects, why, and how they overcame these challenges. Because, in the end, we're all lifelong learners!
My name is Alex Andorra by the way, and I live in Estonia. By day, I'm a data scientist and modeler at the https://www.pymc-labs.io/ (PyMC Labs) consultancy. By night, I don't (yet) fight crime, but I'm an open-source enthusiast and core contributor to the python packages https://docs.pymc.io/ (PyMC) and https://arviz-devs.github.io/arviz/ (ArviZ). I also love https://www.pollsposition.com/ (election forecasting) and, most importantly, Nutella. But I don't like talking about it – I prefer eating it.
So, whether you want to learn Bayesian statistics or hear about the latest libraries, books and applications, this podcast is for you -- just subscribe! You can also support the show and https://www.patreon.com/learnbayesstats (unlock exclusive Bayesian swag on Patreon)!
Then this podcast is for you! You'll hear from researchers and practitioners of all fields about how they use Bayesian statistics, and how in turn YOU can apply these methods in your modeling workflow.
When I started learning Bayesian methods, I really wished there were a podcast out there that could introduce me to the methods, the projects and the people who make all that possible.
So I created "Learning Bayesian Statistics", where you'll get to hear how Bayesian statistics are used to detect black matter in outer space, forecast elections or understand how diseases spread and can ultimately be stopped.
But this show is not only about successes -- it's also about failures, because that's how we learn best. So you'll often hear the guests talking about what *didn't* work in their projects, why, and how they overcame these challenges. Because, in the end, we're all lifelong learners!
My name is Alex Andorra by the way, and I live in Estonia. By day, I'm a data scientist and modeler at the https://www.pymc-labs.io/ (PyMC Labs) consultancy. By night, I don't (yet) fight crime, but I'm an open-source enthusiast and core contributor to the python packages https://docs.pymc.io/ (PyMC) and https://arviz-devs.github.io/arviz/ (ArviZ). I also love https://www.pollsposition.com/ (election forecasting) and, most importantly, Nutella. But I don't like talking about it – I prefer eating it.
So, whether you want to learn Bayesian statistics or hear about the latest libraries, books and applications, this podcast is for you -- just subscribe! You can also support the show and https://www.patreon.com/learnbayesstats (unlock exclusive Bayesian swag on Patreon)!
Episodes
Mentioned books

9 snips
Aug 23, 2023 • 1h 60min
#89 Unlocking the Science of Exercise, Nutrition & Weight Management, with Eric Trexler
Eric Trexler, a researcher at Duke University with a PhD in Human Movement Science, shares his insights on exercise, nutrition, and metabolism. He delves into metabolic adaptation and the complexities of weight management, explaining how caloric intake impacts energy expenditure. Trexler also highlights the role of Bayesian statistics in overcoming challenges in exercise science. The conversation touches on the connection between stoicism and dieting struggles, and the gap between scientific understanding and public misinformation.

4 snips
Aug 10, 2023 • 1h 12min
#88 Bridging Computation & Inference in Artificial Intelligent Systems, with Philipp Hennig
Proudly sponsored by PyMC Labs, the Bayesian Consultancy. Book a call, or get in touch!Listen on PoduramaMy Intuitive Bayes Online Courses1:1 Mentorship with meToday, we’re gonna learn about probabilistic numerics — what they are, what they are good for, and how they relate computation and inference in artificial intelligent systems.To do this, I have the honor of hosting Philipp Hennig, a distinguished expert in this field, and the Chair for the Methods of Machine Learning at the University of Tübingen, Germany. Philipp studied in Heidelberg, also in Germany, and at Imperial College, London. Philipp received his PhD from the University of Cambridge, UK, under the supervision of David MacKay, before moving to Tübingen in 2011. Since his PhD, he has been interested in the connection between computation and inference. With international colleagues, he helped establish the idea of probabilistic numerics, which describes computation as Bayesian inference. His book, Probabilistic Numerics — Computation as Machine Learning, co-authored with Mike Osborne and Hans Kersting, was published by Cambridge University Press in 2022 and is also openly available online. So get comfy to explore the principles that underpin these algorithms, how they differ from traditional numerical methods, and how to incorporate uncertainty into the decision-making process of these algorithms.Our theme music is « Good Bayesian », by Baba Brinkman (feat MC Lars and Mega Ran). Check out his awesome work at https://bababrinkman.com/ !Thank you to my Patrons for making this episode possible!Yusuke Saito, Avi Bryant, Ero Carrera, Giuliano Cruz, Tim Gasser, James Wade, Tradd Salvo, William Benton, James Ahloy, Robin Taylor, Chad Scherrer, Zwelithini Tunyiswa, Bertrand Wilden, James Thompson, Stephen Oates, Gian Luca Di Tanna, Jack Wells, Matthew Maldonado, Ian Costley, Ally Salim, Larry Gill, Ian Moran, Paul Oreto, Colin Caprani, Colin Carroll, Nathaniel Burbank, Michael Osthege, Rémi Louf, Clive Edelsten, Henri Wallen, Hugo Botha, Vinh Nguyen, Raul Maldonado, Marcin Elantkowski, Adam C. Smith, Will Kurt, Andrew Moskowitz, Hector Munoz, Marco Gorelli, Simon Kessell, Bradley Rode, Patrick Kelley, Rick Anderson, Casper de Bruin, Philippe Labonde, Michael Hankin, Cameron Smith, Tomáš Frýda, Ryan Wesslen, Andreas Netti, Riley King, Yoshiyuki Hamajima, Sven De Maeyer, Michael DeCrescenzo, Fergal M, Mason Yahr, Naoya Kanai, Steven Rowland, Aubrey Clayton, Jeannine Sue, Omri Har Shemesh, Scott Anthony Robson, Robert Yolken, Or Duek, Pavel Dusek, Paul Cox, Trey Causey, Andreas Kröpelin, Raphaël R, Nicolas Rode, Gabriel Stechschulte, Arkady, Kurt TeKolste, Gergely Juhasz, Marcus Nölke, Maggi Mackintosh, Grant Pezzolesi, Avram Aelony, Joshua Meehl, Javier Sabio, Kristian Higgins, Alex Jones, Gregorio Aguilar and Matt Rosinski.Visit https://www.patreon.com/learnbayesstats to unlock exclusive Bayesian swag ;)Links from the show:Philipp on Twitter: https://twitter.com/PhilippHennig5Philipp on Github: https://github.com/philipphennigPhilipp on LinkedIn: https://www.linkedin.com/in/philipp-hennig-635832278/An introductory course on Probabilistic Numerics, taught collaboratively by Philipp’s Group: https://youtube.com/playlist?list=PL05umP7R6ij2lwDdj7IkuHoP9vHlEcH0s An introductory tutorial on Probabilistic Numerics: https://youtu.be/0Q1ZTLHULcw Philipp’s book: https://www.probabilistic-numerics.org/textbooks/ProbNum python package: https://probnum.readthedocs.io/en/latest/Probabilistic solvers for differential equations in JAX: https://pnkraemer.github.io/probdiffeq/Probabilistic Numerical Differential Equation Solvers in Julia: https://nathanaelbosch.github.io/ProbNumDiffEq.jl/stable/#Probabilistic-Numerical-Differential-Equation-SolversPhilipp’s research: https://www.probabilistic-numerics.org/Philipp’s academic page: https://uni-tuebingen.de/en/fakultaeten/mathematisch-naturwissenschaftliche-fakultaet/fachbereiche/informatik/lehrstuehle/methods-of-machine-learning/start/ Tübingen Machine Learning on YouTube: https://www.youtube.com/c/TübingenML LBS #74 Optimizing NUTS and Developing the ZeroSumNormal Distribution, with Adrian Seyboldt: https://learnbayesstats.com/episode/74-optimizing-nuts-developing-zerosumnormal-distribution-adrian-seyboldt/LBS #12 Biostatistics and Differential Equations, with Demetri Pananos: https://learnbayesstats.com/episode/12-biostatistics-and-differential-equations-with-demetri-pananos/Abstractby Christoph BambergIn episode 88 with Philipp Henning, chair of Methods in Machine Learning at the Eberhard Karls University Tübingen, we learn about new, technical areas for the Bayesian way of thinking: Probabilistic numerics.Philipp gives us a conceptual introduction to Machine Learning as “refining a model through data” and explains what challenges Machine Learning phases due to the intractable nature of data and the used computations. The Bayesian approach, emphasising uncertainty over estimates and parameters, naturally lends itself for handling these issues. In his research group, Philipp tries to find more general implementations of classically used algorithms, while maintaining computational efficiency. They successfully achieve this goal by bringing in the Bayesian approach to inferences. Philipp explains probabilistic numerics as “redescrbiing everything a computer does as Bayesian inference” and how this approach is suitable for advancing Machine Learning.We expand on how to handle uncertainty in machine learning and Philipp details his teams approach for handling this issue.We also collect many resources for those interested in probabilistic numerics and finally talk about the future of this field.TranscriptThis is an automatic transcript and may therefore contain errors. Please get in touch if you're willing to correct them.

17 snips
Jul 30, 2023 • 1h 9min
#87 Unlocking the Power of Bayesian Causal Inference, with Ben Vincent
Proudly sponsored by PyMC Labs, the Bayesian Consultancy. Book a call, or get in touch!Listen on PoduramaMy Intuitive Bayes Online Courses1:1 Mentorship with meI’ll be honest — this episode is long overdue. Not only because Ben Vincent is a friend, fellow PyMC Labs developer, and outstanding Bayesian modeler. But because he works on so many fascinating topics — so I’m all the happier to finally have him on the show!In this episode, we’re gonna focus on causal inference, how it naturally extends Bayesian modeling, and how you can use the CausalPy open-source package to supercharge your Bayesian causal inference. We’ll also touch on marketing models and the pymc-marketing package, because, well, Ben does a lot of stuff ;)Ben got his PhD in neuroscience at Sussex University, in the UK. After a postdoc at the University of Bristol, working on robots and active vision, as well as 15 years as a lecturer at the Scottish University of Dundee, he switched to the private sector, working with us full time at PyMC Labs — and that is a treat!When he’s not working, Ben loves running 5k’s, cycling in the forest, lifting weights, and… learning about modern monetary theory.Our theme music is « Good Bayesian », by Baba Brinkman (feat MC Lars and Mega Ran). Check out his awesome work at https://bababrinkman.com/ !Thank you to my Patrons for making this episode possible!Yusuke Saito, Avi Bryant, Ero Carrera, Giuliano Cruz, Tim Gasser, James Wade, Tradd Salvo, William Benton, James Ahloy, Robin Taylor,, Chad Scherrer, Nathaniel Neitzke, Zwelithini Tunyiswa, Bertrand Wilden, James Thompson, Stephen Oates, Gian Luca Di Tanna, Jack Wells, Matthew Maldonado, Ian Costley, Ally Salim, Larry Gill, Ian Moran, Paul Oreto, Colin Caprani, Colin Carroll, Nathaniel Burbank, Michael Osthege, Rémi Louf, Clive Edelsten, Henri Wallen, Hugo Botha, Vinh Nguyen, Raul Maldonado, Marcin Elantkowski, Adam C. Smith, Will Kurt, Andrew Moskowitz, Hector Munoz, Marco Gorelli, Simon Kessell, Bradley Rode, Patrick Kelley, Rick Anderson, Casper de Bruin, Philippe Labonde, Michael Hankin, Cameron Smith, Tomáš Frýda, Ryan Wesslen, Andreas Netti, Riley King, Yoshiyuki Hamajima, Sven De Maeyer, Michael DeCrescenzo, Fergal M, Mason Yahr, Naoya Kanai, Steven Rowland, Aubrey Clayton, Jeannine Sue, Omri Har Shemesh, Scott Anthony Robson, Robert Yolken, Or Duek, Pavel Dusek, Paul Cox, Trey Causey, Andreas Kröpelin, Raphaël R, Nicolas Rode, Gabriel Stechschulte, Arkady, Kurt TeKolste, Gergely Juhasz, Marcus Nölke, Maggi Mackintosh, Grant Pezzolesi, Avram Aelony and Joshua Meehl.Visit https://www.patreon.com/learnbayesstats to unlock exclusive Bayesian swag ;)Links from the show:Ben’s website: https://drbenvincent.github.io/Ben on GitHub: https://github.com/drbenvincentBen on Twitter: https://twitter.com/inferencelabBen on LinkedIn: https://www.linkedin.com/in/dr-benjamin-vincent-503571127/CausalPy – Causal inference for quasi-experiments: https://causalpy.readthedocs.io/en/latest/PyMC Marketing – Bayesian marketing toolbox in PyMC: https://www.pymc-marketing.io/en/stable/index.htmlPyMC Labs : https://www.pymc-labs.io/products/LBS #23 – Bayesian Stats in Business and Marketing Analytics, with Elea McDonnel Feit: https://learnbayesstats.com/episode/23-bayesian-stats-in-business-and-marketing-analytics-with-elea-mcdonnel-feit/LBS #63 – Media Mix Models & Bayes for Marketing, with Luciano Paz: https://learnbayesstats.com/episode/63-media-mix-models-bayes-marketing-luciano-paz/Abstractwritten by Christoph BambergIn this podcast episode, our guest, Ben Vincent, a fellow member of PyMC Labs with a PhD in Neuroscience and extensive experience in teaching and data analysis of course, introduces us to CausalPy and PyMC Marketing.During his academic career, Ben got introduced to Bayesian statistics but, like most academics, did not come across causal inference. We discuss the importance of a systematic causal approach for important questions like health care interventions or marketing investments. Although causality is somewhat orthogonal to the choice of statistical approach, Bayesian statistics is a good basis for causal analyses, for example in the for of Directed Acyclical Graphs. To make causal inference more accessible, Ben developed a Python package called CausalPy, which allows you perform common causal inferences, e.g. working with natural experiments.Ben was also involved in the development of PyMC Marketing, a package that conveniently bundles important analysis capacities for Marketing. The package focuses on Media Mix Modelling and customer lifetime analysis. We also talked about his extensive experience teaching statistics at university and current teaching of Bayesian methods in industry. His advice to students is to really engage with your learning material, coding through examples, making the learning more pleasurable and practical. TranscriptPlease note that this is an automated transcript that may contain errors. Feel free to reach out if you're willing to correct them.

Jul 14, 2023 • 59min
#86 Exploring Research Synchronous Languages & Hybrid Systems, with Guillaume Baudart
Proudly sponsored by PyMC Labs, the Bayesian Consultancy. Book a call, or get in touch!Listen on PoduramaMy Intuitive Bayes Online Courses1:1 Mentorship with meThis episode is unlike anything I’ve covered so far on the show. Let me ask you: Do you know what a research synchronous language is? What about hybrid systems? Last try: have you heard of Zelus, or ProbZelus?If you answered “no” to one of the above, then you’re just like me! And that’s why I invited Guillaume Baudart for this episode — to teach us about all these fascinating topics!A researcher in the PARKAS team of Inria, Guillaume's research focuses on probabilistic and reactive programming languages. In particular, he works on ProbZelus, a probabilistic extension to Zelus, itself a research synchronous language to implement hybrid systems.To simplify, Zelus is a modeling framework to simulate the dynamics of systems both smooth and subject to discrete dynamics — if you’ve ever worked with ODEs, you may be familiar with these terms.If you’re not — great, Guillaume will explain everything in the episode! And I know it might sound niche, but this kind of approach actually has very important applications — such as proving that there are no bugs in a program.Guillaume did his PhD at École Normale Supérieure, in Paris, working on reactive programming languages and quasi-periodic systems. He then worked in the AI programming team of IBM Research, before coming back to the École Normale Supérieure, working mostly on reactive and probabilistic programming.In his free time, Guillaume loves spending time with his family, playing the violin with friends, and… cooking!Our theme music is « Good Bayesian », by Baba Brinkman (feat MC Lars and Mega Ran). Check out his awesome work at https://bababrinkman.com/ !Thank you to my Patrons for making this episode possible!Yusuke Saito, Avi Bryant, Ero Carrera, Giuliano Cruz, Tim Gasser, James Wade, Tradd Salvo, William Benton, James Ahloy, Robin Taylor,, Chad Scherrer, Nathaniel Neitzke, Zwelithini Tunyiswa, Bertrand Wilden, James Thompson, Stephen Oates, Gian Luca Di Tanna, Jack Wells, Matthew Maldonado, Ian Costley, Ally Salim, Larry Gill, Ian Moran, Paul Oreto, Colin Caprani, Colin Carroll, Nathaniel Burbank, Michael Osthege, Rémi Louf, Clive Edelsten, Henri Wallen, Hugo Botha, Vinh Nguyen, Raul Maldonado, Marcin Elantkowski, Adam C. Smith, Will Kurt, Andrew Moskowitz, Hector Munoz, Marco Gorelli, Simon Kessell, Bradley Rode, Patrick Kelley, Rick Anderson, Casper de Bruin, Philippe Labonde, Michael Hankin, Cameron Smith, Tomáš Frýda, Ryan Wesslen, Andreas Netti, Riley King, Yoshiyuki Hamajima, Sven De Maeyer, Michael DeCrescenzo, Fergal M, Mason Yahr, Naoya Kanai, Steven Rowland, Aubrey Clayton, Jeannine Sue, Omri Har Shemesh, Scott Anthony Robson, Robert Yolken, Or Duek, Pavel Dusek, Paul Cox, Trey Causey, Andreas Kröpelin, Raphaël R, Nicolas Rode, Gabriel Stechschulte, Arkady, Kurt TeKolste, Gergely Juhasz, Marcus Nölke, Maggi Mackintosh, Grant Pezzolesi, Avram Aelony and Joshua Meehl.Visit https://www.patreon.com/learnbayesstats to unlock exclusive Bayesian swag ;)Links from the show:Guillaume’s website: https://guillaume.baudart.eu/ProbZelus on GitHub: https://github.com/IBM/probzelusZelus docs: https://zelus.di.ens.fr/Short Zelus introduction: https://www.di.ens.fr/~pouzet/bib/hscc13.pdf Guillaume’s course : https://wikimpri.dptinfo.ens-cachan.fr/doku.php?id=cours:c-2-40LBS #74 – Optimizing NUTS and Developing the ZeroSumNormal Distribution, with Adrian Seyboldt: https://learnbayesstats.com/episode/74-optimizing-nuts-developing-zerosumnormal-distribution-adrian-seyboldt/ProbZelus (design, semantics, delayed-sampling): https://dl.acm.org/doi/abs/10.1145/3385412.3386009Semi-symbolic inference: https://dl.acm.org/doi/abs/10.1145/3563347Static analysis for bounded memory inference: https://dl.acm.org/doi/abs/10.1145/3485492Abstractby Christoph BambergGuillaume Baudart is researcher at Inria in the PARKAS team at the Département d'Informatique (DI) of the École normale supérieure. He joins us for episode 86 to tell us about ProbZelus, a synchronous probabilistic programming language, that he develops.We have not covered synchronous languages yet, so, Guillaume gives us some context on this kind of programming approach and how ProbZelus adds probabilistic notions to it.He explains the advantages of the probabilistic aspects of ProbZelus and what practitioners may profit from it. For example, synchronous languages are used to program and test autopilots of planes and ensure that they do not have any bugs. ProbZelus may be useful here as Guillaume argues.Finally, we also touch upon his teaching work and what difficulties he encounters in teaching probabilistic programming. TranscriptPlease note that this is an automated transcript that may contain errors. Feel free to reach out if you're willing to correct them.

Jun 27, 2023 • 1h 6min
#85 A Brief History of Sports Analytics, with Jim Albert
Proudly sponsored by PyMC Labs, the Bayesian Consultancy. Book a call, or get in touch!My Intuitive Bayes Online Courses1:1 Mentorship with meIn this episode, I am honored to talk with a legend of sports analytics in general, and baseball analytics in particular. I am of course talking about Jim Albert.Jim grew up in the Philadelphia area and studied statistics at Purdue University. He then spent his entire 41-year academic career at Bowling Green State University, which gave him a wide diversity of classes to teach – from intro statistics through doctoral level.As you’ll hear, he’s always had a passion for Bayesian education, Bayesian modeling and learning about statistics through sports. I find that passion fascinating about Jim, and I suspect that’s one of the main reasons for his prolific career — really, the list of his writings and teachings is impressive; just go take a look at the show notes.Now an Emeritus Professor of Bowling Green, Jim is retired, but still an active tennis player and writer on sports analytics — his blog, “Exploring Baseball with R”, is nearing 400 posts!Our theme music is « Good Bayesian », by Baba Brinkman (feat MC Lars and Mega Ran). Check out his awesome work at https://bababrinkman.com/ !Thank you to my Patrons for making this episode possible!Yusuke Saito, Avi Bryant, Ero Carrera, Giuliano Cruz, Tim Gasser, James Wade, Tradd Salvo, William Benton, James Ahloy, Robin Taylor,, Chad Scherrer, Nathaniel Neitzke, Zwelithini Tunyiswa, Bertrand Wilden, James Thompson, Stephen Oates, Gian Luca Di Tanna, Jack Wells, Matthew Maldonado, Ian Costley, Ally Salim, Larry Gill, Ian Moran, Paul Oreto, Colin Caprani, Colin Carroll, Nathaniel Burbank, Michael Osthege, Rémi Louf, Clive Edelsten, Henri Wallen, Hugo Botha, Vinh Nguyen, Raul Maldonado, Marcin Elantkowski, Adam C. Smith, Will Kurt, Andrew Moskowitz, Hector Munoz, Marco Gorelli, Simon Kessell, Bradley Rode, Patrick Kelley, Rick Anderson, Casper de Bruin, Philippe Labonde, Michael Hankin, Cameron Smith, Tomáš Frýda, Ryan Wesslen, Andreas Netti, Riley King, Yoshiyuki Hamajima, Sven De Maeyer, Michael DeCrescenzo, Fergal M, Mason Yahr, Naoya Kanai, Steven Rowland, Aubrey Clayton, Jeannine Sue, Omri Har Shemesh, Scott Anthony Robson, Robert Yolken, Or Duek, Pavel Dusek, Paul Cox, Trey Causey, Andreas Kröpelin, Raphaël R, Nicolas Rode, Gabriel Stechschulte, Arkady, Kurt TeKolste, Gergely Juhasz, Marcus Nölke, Maggi Mackintosh, Grant Pezzolesi, Avram Aelony and Joshua Meehl.Visit https://www.patreon.com/learnbayesstats to unlock exclusive Bayesian swag ;)Links from the show:Jim’s website: https://bayesball.github.io/Jim’s baseball blog: https://baseballwithr.wordpress.com/Jim on GitHub: https://github.com/bayesballJim on Twitter: https://twitter.com/albertbayesJim on Linkedin: https://www.linkedin.com/in/jim-albert-22846b41/Jim’s baseball research: https://bayesball.github.io/BLOG/Probability and Bayesian Modeling book: https://monika76five.github.io/ProbBayes/Curve Ball -- Baseball, Statistics, and the Role of Chance in the Game: https://bayesball.github.io/curveball/curveball.htmVisualizing Baseball: https://bayesball.github.io/VB/Analyzing Baseball Data with R: https://www.amazon.com/gp/product/0815353510?pf_rd_p=c2945051-950f-485c-b4df-15aac5223b10&pf_rd_r=SFAV7QEGY9A2EDADZTJ5Teaching Statistics Using Baseball: https://bayesball.github.io/TSUB2/Ordinal Data Modeling: https://link.springer.com/book/10.1007/b98832?changeHeaderWorkshop Statistics (an intro stats course taught from a Bayesian point of view): https://bayesball.github.io/nsf_web/main.htmLBS #76, The Past, Present & Future of Stan, with Bob Carpenter: https://learnbayesstats.com/episode/76-past-present-future-of-stan-bob-carpenter/MCMC Interactive Gallery: https://chi-feng.github.io/mcmc-demo/app.html?algorithm=HamiltonianMC&target=bananaAbstractwritten by Christoph BambergIn this episode, Jim Albert, a legend of sports analytics, Emeritus Professor at Bowling Green university, is our guest.We talk about a range of topics, including his early interest in math and sports, challenges in analysing sports data and his experience teaching statistics.We trace back the history of baseball sport analytics to the 1960s and discuss how new, advanced ways to collect data change the possibilities of what can be modelled.There are also statistical approaches to American football, soccer and basketball games. Jim explains why these team sports are more difficult to model than baseball. The conversation then turns to Jim’s substantial experience teaching statistics ad the challenges he sees in that. Jim worked on several books on sports analytics and has many blog posts on this topic.We also touch upon the challenges of prior elicitation, a topic that has come up frequently in recent podcasts, how different stakeholders such as coaches and managers think differently about the sport and how to extract priors from their information.For more tune in to episode 85 with Jim Albert.Chapters[00:00:00] Episode Begins[00:04:04] How did you get into the world of statistics?[00:11:17] Baseball is more advanced on the analytics path compared to other sports[00:17:02] How is the data collected?[00:24:43] Why is sports analytics important and is it turning humans into robots?[00:32:51] Loss in translation problem between modellers and domain experts...?[00:41:43] Active learning and learning through workshops[00:51:08] Principles before methods[00:52:30] Your favorite sports analytics model[01:02:07] If you had unlimited time and resources which problem would you try to solve?TranscriptPlease note that this transcript is generated automatically and may contain errors. Feel free to reach out if you are willing to correct them.

Jun 13, 2023 • 1h 6min
#84 Causality in Neuroscience & Psychology, with Konrad Kording
Proudly sponsored by PyMC Labs, the Bayesian Consultancy. Book a call, or get in touch!My Intuitive Bayes Online Courses1:1 Mentorship with meThis is another installment in our neuroscience modeling series! This time, I talked with Konrad Kording, about the role of Bayesian stats in neuroscience and psychology, electrophysiological data to study what neurons do, and how this helps explain human behavior.Konrad studied at ETH Zurich, then went to UC London and MIT for his postdocs. After a decade at Northwestern University, he is now Penn Integrated Knowledge Professor at the University of Pennsylvania.As you’ll hear, Konrad is particularly interested in the question of how the brain solves the credit assignment problem and similarly how we should assign credit in the real world (through causality). Building on this, he is also interested in applications of causality in biomedical research.And… he’s also a big hiker, skier and salsa dancer!Our theme music is « Good Bayesian », by Baba Brinkman (feat MC Lars and Mega Ran). Check out his awesome work at https://bababrinkman.com/ !Thank you to my Patrons for making this episode possible!Yusuke Saito, Avi Bryant, Ero Carrera, Giuliano Cruz, Tim Gasser, James Wade, Tradd Salvo, William Benton, James Ahloy, Robin Taylor,, Chad Scherrer, Nathaniel Neitzke, Zwelithini Tunyiswa, Bertrand Wilden, James Thompson, Stephen Oates, Gian Luca Di Tanna, Jack Wells, Matthew Maldonado, Ian Costley, Ally Salim, Larry Gill, Ian Moran, Paul Oreto, Colin Caprani, Colin Carroll, Nathaniel Burbank, Michael Osthege, Rémi Louf, Clive Edelsten, Henri Wallen, Hugo Botha, Vinh Nguyen, Raul Maldonado, Marcin Elantkowski, Adam C. Smith, Will Kurt, Andrew Moskowitz, Hector Munoz, Marco Gorelli, Simon Kessell, Bradley Rode, Patrick Kelley, Rick Anderson, Casper de Bruin, Philippe Labonde, Michael Hankin, Cameron Smith, Tomáš Frýda, Ryan Wesslen, Andreas Netti, Riley King, Yoshiyuki Hamajima, Sven De Maeyer, Michael DeCrescenzo, Fergal M, Mason Yahr, Naoya Kanai, Steven Rowland, Aubrey Clayton, Jeannine Sue, Omri Har Shemesh, Scott Anthony Robson, Robert Yolken, Or Duek, Pavel Dusek, Paul Cox, Trey Causey, Andreas Kröpelin, Raphaël R, Nicolas Rode, Gabriel Stechschulte, Arkady, Kurt TeKolste, Gergely Juhasz, Marcus Nölke, Maggi Mackintosh, Grant Pezzolesi, Avram Aelony and Joshua Meehl.Visit https://www.patreon.com/learnbayesstats to unlock exclusive Bayesian swag ;)Links from the show:Konrad’s lab: https://kordinglab.com/Konrad’s lab on GitHub: https://github.com/KordingLabKonrad’s lab on Twitter: https://twitter.com/KordingLabLBS #81, Neuroscience of Perception: Exploring the Brain, with Alan Stocker: https://learnbayesstats.com/episode/81-neuroscience-of-perception-exploring-the-brain-alan-stocker/LBS #77, How a Simple Dress Helped Uncover Hidden Prejudices, with Pascal Wallisch: https://learnbayesstats.com/episode/77-how-a-simple-dress-helped-uncover-hidden-prejudices-pascal-wallisch/The Sports Gene, Inside the Science of Extraordinary Athletic Performance: https://davidepstein.com/david-epstein-the-sports-gene/Decoding with good ML: https://github.com/KordingLab/Neural_Decoding and https://www.eneuro.org/content/7/4/ENEURO.0506-19.2020Bayesian decoding: https://www.ncbi.nlm.nih.gov/pmc/articles/PMC5578432/Textbook on Bayesian modeling of behavior: bayesianmodeling.comBayesian philosophy: https://www.ncbi.nlm.nih.gov/pmc/articles/PMC3981868/Konrad talking about Neuromatch Bayes day: https://www.youtube.com/watch?v=neDaPap_5TgThe Neuromatch Bayes tutorials: compneuro.neuromatch.ioTranscriptPlease note that this is an automatic transcript and may contain errors. Feel free to reach out if you would like to correct them.

May 25, 2023 • 1h 17min
#83 Multilevel Regression, Post-Stratification & Electoral Dynamics, with Tarmo Jüristo
Proudly sponsored by PyMC Labs, the Bayesian Consultancy. Book a call, or get in touch!My Intuitive Bayes Online Courses1:1 Mentorship with meOne of the greatest features of this podcast, and my work in general, is that I keep getting surprised. Along the way, I keep learning, and I meet fascinating people, like Tarmo Jüristo.Tarmo is hard to describe. These days, he’s heading an NGO called Salk, in the Baltic state called Estonia. Among other things, they are studying and forecasting elections, which is how we met and ended up collaborating with PyMC Labs, our Bayesian consultancy.But Tarmo is much more than that. Born in 1971 in what was still the Soviet Union, he graduated in finance from Tartu University. He worked in finance and investment banking until the 2009 crisis, when he quit and started a doctorate in… cultural studies. He then went on to write for theater and TV, teaching literature, anthropology and philosophy. An avid world traveler, he also teaches kendo and Brazilian jiu-jitsu.As you’ll hear in the episode, after lots of adventures, he established Salk, and they just used a Bayesian hierarchical model with post-stratification to forecast the results of the 2023 Estonian parliamentary elections and target the campaign efforts to specific demographics.Oh, and let thing: Tarmo is a fan of the show — I told you he was a great guy ;)Our theme music is « Good Bayesian », by Baba Brinkman (feat MC Lars and Mega Ran). Check out his awesome work at https://bababrinkman.com/ !Thank you to my Patrons for making this episode possible!Yusuke Saito, Avi Bryant, Ero Carrera, Giuliano Cruz, Tim Gasser, James Wade, Tradd Salvo, William Benton, James Ahloy, Robin Taylor,, Chad Scherrer, Nathaniel Neitzke, Zwelithini Tunyiswa, Bertrand Wilden, James Thompson, Stephen Oates, Gian Luca Di Tanna, Jack Wells, Matthew Maldonado, Ian Costley, Ally Salim, Larry Gill, Ian Moran, Paul Oreto, Colin Caprani, Colin Carroll, Nathaniel Burbank, Michael Osthege, Rémi Louf, Clive Edelsten, Henri Wallen, Hugo Botha, Vinh Nguyen, Raul Maldonado, Marcin Elantkowski, Adam C. Smith, Will Kurt, Andrew Moskowitz, Hector Munoz, Marco Gorelli, Simon Kessell, Bradley Rode, Patrick Kelley, Rick Anderson, Casper de Bruin, Philippe Labonde, Michael Hankin, Cameron Smith, Tomáš Frýda, Ryan Wesslen, Andreas Netti, Riley King, Yoshiyuki Hamajima, Sven De Maeyer, Michael DeCrescenzo, Fergal M, Mason Yahr, Naoya Kanai, Steven Rowland, Aubrey Clayton, Jeannine Sue, Omri Har Shemesh, Scott Anthony Robson, Robert Yolken, Or Duek, Pavel Dusek, Paul Cox, Trey Causey, Andreas Kröpelin, Raphaël R, Nicolas Rode, Gabriel Stechschulte, Arkady, Kurt TeKolste, Gergely Juhasz, Marcus Nölke, Maggi Mackintosh and Grant Pezzolesi.Visit https://www.patreon.com/learnbayesstats to unlock exclusive Bayesian swag ;)Links from the show:Tarmo on GitHub: https://github.com/tarmojuristoTarmo on Linkedin: https://www.linkedin.com/in/tarmo-j%C3%BCristo-7018bb7/Tarmo on Twitter: https://twitter.com/tarmojuristoSalk website: https://salk.ee/Hierarchical Bayesian Modeling of Survey Data with Post-stratification: https://www.youtube.com/watch?v=efID35XUQ3IAbstractby Christoph BambergIn episode 83 of the podcast Tarmo Jüristo is our guest. He recently received media attention for his electoral forecasting in the Estonian election and potential positive role in aiding liberal parties gain more votes than expected. Tarmo explains to us how he used Bayesian models with his NGO SALK to forecast the election and how he leveraged these models to unify the different liberal parties that participated in the election. So, we get a firsthand view of how to use Bayesian modelling smartly.Furthermore, we talk about when to use Bayesian models, difficulties in modelling survey data and how post-stratification can help.He also explains how he, with the help of PyMC Labs, added Gaussian Processes to his models to better model the time-series structure of their survey data. We close this episode by discussing the responsibility that comes with modelling data in politics. Transcriptplease note that the transcript was generated automatically and may therefore contain errors. Feel free to reach out if you're willing to correct them.

May 5, 2023 • 1h 7min
#82 Sequential Monte Carlo & Bayesian Computation Algorithms, with Nicolas Chopin
Proudly sponsored by PyMC Labs, the Bayesian Consultancy. Book a call, or get in touch!My Intuitive Bayes Online Courses1:1 Mentorship with me------------------------------------------------------------------------------Max Kochurov’s State of Bayes Lecture Series: https://www.youtube.com/playlist?list=PL1iMFW7frOOsh5KOcfvKWM12bjh8zs9BQSign up here for upcoming lessons: https://www.meetup.com/pymc-labs-online-meetup/events/293101751/------------------------------------------------------------------------------We talk a lot about different MCMC methods on this podcast, because they are the workhorses of the Bayesian models. But other methods exist to infer the posterior distributions of your models — like Sequential Monte Carlo (SMC) for instance. You’ve never heard of SMC? Well perfect, because Nicolas Chopin is gonna tell you all about it in this episode!A lecturer at the French university of ENSAE since 2006, Nicolas is one of the world experts on SMC. Before that, he graduated from Ecole Polytechnique and… ENSAE, where he did his PhD from 1999 to 2003.Outside of work, Nicolas enjoys spending time with his family, practicing aikido, and reading a lot of books.Our theme music is « Good Bayesian », by Baba Brinkman (feat MC Lars and Mega Ran). Check out his awesome work at https://bababrinkman.com/ !Thank you to my Patrons for making this episode possible!Yusuke Saito, Avi Bryant, Ero Carrera, Giuliano Cruz, Tim Gasser, James Wade, Tradd Salvo, William Benton, James Ahloy, Robin Taylor,, Chad Scherrer, Nathaniel Neitzke, Zwelithini Tunyiswa, Bertrand Wilden, James Thompson, Stephen Oates, Gian Luca Di Tanna, Jack Wells, Matthew Maldonado, Ian Costley, Ally Salim, Larry Gill, Ian Moran, Paul Oreto, Colin Caprani, Colin Carroll, Nathaniel Burbank, Michael Osthege, Rémi Louf, Clive Edelsten, Henri Wallen, Hugo Botha, Vinh Nguyen, Raul Maldonado, Marcin Elantkowski, Adam C. Smith, Will Kurt, Andrew Moskowitz, Hector Munoz, Marco Gorelli, Simon Kessell, Bradley Rode, Patrick Kelley, Rick Anderson, Casper de Bruin, Philippe Labonde, Michael Hankin, Cameron Smith, Tomáš Frýda, Ryan Wesslen, Andreas Netti, Riley King, Yoshiyuki Hamajima, Sven De Maeyer, Michael DeCrescenzo, Fergal M, Mason Yahr, Naoya Kanai, Steven Rowland, Aubrey Clayton, Jeannine Sue, Omri Har Shemesh, Scott Anthony Robson, Robert Yolken, Or Duek, Pavel Dusek, Paul Cox, Trey Causey, Andreas Kröpelin, Raphaël R, Nicolas Rode, Gabriel Stechschulte, Arkady and Kurt TeKolste.Visit https://www.patreon.com/learnbayesstats to unlock exclusive Bayesian swag ;)Links from the show:Old episodes relevant to these topics:LBS #14, Hidden Markov Models & Statistical Ecology, with Vianey Leos-Barajas: https://learnbayesstats.com/episode/14-hidden-markov-models-statistical-ecology-with-vianey-leos-barajas/LBS #41, Thinking Bayes, with Allen Downey: https://learnbayesstats.com/episode/41-think-bayes-allen-downey/Nicolas’ show notes:Nicolas on Mastodon: nchopin@mathstodon.xyz2-hour introduction to particle filters: https://www.youtube.com/watch?v=mE_PJ9ASc8YNicolas’ website: https://nchopin.github.io/Nicolas on GitHub: https://github.com/nchopinNicolas on Linkedin: https://www.linkedin.com/in/nicolas-chopin-442a78102/Nicolas’ blog (shared with others): https://statisfaction.wordpress.com/INLA original paper: https://people.bath.ac.uk/man54/SAMBa/ITTs/ITT2/EDF/INLARueetal2009.pdfNicolas’ book, An introduction to Sequential Monte Carlo: https://nchopin.github.io/books.htmlLaplace’s Demon, A Seminar Series about Bayesian Machine Learning at Scale: https://ailab.criteo.com/laplaces-demon-bayesian-machine-learning-at-scale/Paper about Expectation Propagation, Leave Pima Indians Alone – Binary Regression as a Benchmark for Bayesian Computation: https://projecteuclid.org/journals/statistical-science/volume-32/issue-1/Leave-Pima-Indians-Alone--Binary-Regression-as-a-Benchmark/10.1214/16-STS581.fullBlackjax website: https://blackjax-devs.github.io/blackjax/Abstractby Christoph BambergIn episode 82 Nicolas Chopin is our guest. He is a graduate from the Ecole Polytechnique and currently lectures at the French university of ENSAE. He is a specialist for Sequential Monte Carlo (SMC) samplers and explains in detail what they are, clearing up some confusion about what SMC stands for and when to use them. We discuss the advantages of SMC over other types of commonly used samplers for bayesian models such as MCMC or Gibbs samplers. Besides a detailed look at SMC we also cover INLA. INLA stands for Integrated Nested LaPlace Approximation. INLA can be a fast, approximate sampler for specific kinds of models. It works well for geographic data and relationships, such as for example relationships between regions in a country.We discuss the difficulties with and future of SMC and INLA and probabilistic sampling in general.Transcriptplease note that the transcript was generated automatically and may therefore contain errors. Feel free to reach out if you're willing to correct them.

4 snips
Apr 24, 2023 • 1h 15min
#81 Neuroscience of Perception: Exploring the Brain, with Alan Stocker
Proudly sponsored by PyMC Labs, the Bayesian Consultancy. Book a call, or get in touch!My Intuitive Bayes Online Courses1:1 Mentorship with meDid you know that the way your brain perceives speed depends on your priors? And it’s not the same at night? And it’s not the same for everybody?This is another of these episodes I love where we dive into neuroscience, how the brain works, and how it relates to Bayesian stats. It’s actually a follow-up to episode 77, where Pascal Wallisch told us how the famous black and blue dress tells a lot about our priors about how we perceive the world. So I strongly recommend listening to episode 77 first, and then come back here, to have your mind blown away again, this time by Alan Stocker.Alan was born and raised in Switzerland. After a PhD in physics at ETH Zurich, he somehow found himself doing neuroscience, during a postdoc at NYU. And then he never stopped — still leading the Computational Perception and Cognition Laboratory of the University of Pennsylvania.But Alan is also a man of music (playing the piano when he can), a man of coffee (he’ll never refuse an olympia cremina or a kafatek) and a man of the outdoors (he loves trashing through deep powder with his snowboard).Our theme music is « Good Bayesian », by Baba Brinkman (feat MC Lars and Mega Ran). Check out his awesome work at https://bababrinkman.com/ !Thank you to my Patrons for making this episode possible!Yusuke Saito, Avi Bryant, Ero Carrera, Giuliano Cruz, Tim Gasser, James Wade, Tradd Salvo, William Benton, James Ahloy, Robin Taylor, Thomas Wiecki, Chad Scherrer, Nathaniel Neitzke, Zwelithini Tunyiswa, Bertrand Wilden, James Thompson, Stephen Oates, Gian Luca Di Tanna, Jack Wells, Matthew Maldonado, Ian Costley, Ally Salim, Larry Gill, Joshua Duncan, Ian Moran, Paul Oreto, Colin Caprani, Colin Carroll, Nathaniel Burbank, Michael Osthege, Rémi Louf, Clive Edelsten, Henri Wallen, Hugo Botha, Vinh Nguyen, Raul Maldonado, Marcin Elantkowski, Adam C. Smith, Will Kurt, Andrew Moskowitz, Hector Munoz, Marco Gorelli, Simon Kessell, Bradley Rode, Patrick Kelley, Rick Anderson, Casper de Bruin, Philippe Labonde, Michael Hankin, Cameron Smith, Tomáš Frýda, Ryan Wesslen, Andreas Netti, Riley King, Yoshiyuki Hamajima, Sven De Maeyer, Michael DeCrescenzo, Fergal M, Mason Yahr, Naoya Kanai, Steven Rowland, Aubrey Clayton, Jeannine Sue, Omri Har Shemesh, Scott Anthony Robson, David Haas, Robert Yolken, Or Duek, Pavel Dusek, Paul Cox, Trey Causey, Andreas Kröpelin, Raphaël R, Nicolas Rode, Gabriel Stechschulte, Arkady and Kurt TeKolste.Visit https://www.patreon.com/learnbayesstats to unlock exclusive Bayesian swag ;)Links from the show:Alan’s website: https://www.sas.upenn.edu/~astocker/lab/members-files/alan.phpNoise characteristics and prior expectations in human visual speed perception: https://www.nature.com/articles/nn1669Combining efficient coding with Bayesian inference as a model of human perception:Video: https://vimeo.com/138238753Paper: https://www.nature.com/articles/nn.4105LBS #77 How a Simple Dress Helped Uncover Hidden Prejudices, with Pascal Wallisch: https://learnbayesstats.com/episode/77-how-a-simple-dress-helped-uncover-hidden-prejudices-pascal-wallisch/LBS #72 Why the Universe is so Deliciously Crazy, with Daniel Whiteson: https://learnbayesstats.com/episode/72-why-the-universe-is-so-deliciously-crazy-daniel-whiteson/Abstractby Christoph BambergIn episode 81 of the podcast, Alan Stocker helps us update our priors of how the brain works. Alan, born in Switzerland, studied mechanical engineering and earned his PhD in physics before being introduced to the field of neuroscience through an internship. He is now Associate Professor at the University of Pennsylvania. Our conversation covers various topics related to the human brain and whether it what it does can be characterised as a Bayesian inferences. Low-level visual processing, such as identifying the orientation of moving grids, can be explained with reference to Bayesian priors and updating under uncertainty. We go through several examples of this such as driving a car in foggy conditions. More abstract cognitive processes, such as reasoning about politics, may be more difficult to explain in Bayesian terms.We also touch upon the question to what degree priors may be innate and how to educate people to change their priors.In the end, Alan gives two recommendations for improving your Bayesian inferences in a political context: 1) Go out and get your own feedback and 2) try to give and receive true feedback. Listen to the episode for details.Transcriptplease note that the transcript was generated automatically and may therefore contain errors. Feel free to reach out if you're willing to correct them.

Apr 11, 2023 • 1h 9min
#80 Bayesian Additive Regression Trees (BARTs), with Sameer Deshpande
Proudly sponsored by PyMC Labs, the Bayesian Consultancy. Book a call, or get in touch!I’m sure you know at least one Bart. Maybe you’ve even used one — but you’re not proud of it, because you didn’t know what you were doing. Thankfully, in this episode, we’ll go to the roots of regression trees — oh yeah, that’s what BART stands for. What were you thinking about?Our tree expert will be no one else than Sameer Deshpande. Sameer is an assistant professor of Statistics at the University of Wisconsin-Madison. Prior to that, he completed a postdoc at MIT and earned his Ph.D. in Statistics from UPenn.On the methodological front, he is interested in Bayesian hierarchical modeling, regression trees, model selection, and causal inference. Much of his applied work is motivated by an interest in understanding the long-term health consequences of playing American-style tackle football. He also enjoys modeling sports data and was a finalist in the 2019 NFL Big Data Bowl.Outside of Statistics, he enjoys cooking, making cocktails, and photography — sometimes doing all of those at the same time…Our theme music is « Good Bayesian », by Baba Brinkman (feat MC Lars and Mega Ran). Check out his awesome work at https://bababrinkman.com/ !Thank you to my Patrons for making this episode possible!Yusuke Saito, Avi Bryant, Ero Carrera, Giuliano Cruz, Tim Gasser, James Wade, Tradd Salvo, William Benton, James Ahloy, Robin Taylor, Thomas Wiecki, Chad Scherrer, Nathaniel Neitzke, Zwelithini Tunyiswa, Bertrand Wilden, James Thompson, Stephen Oates, Gian Luca Di Tanna, Jack Wells, Matthew Maldonado, Ian Costley, Ally Salim, Larry Gill, Joshua Duncan, Ian Moran, Paul Oreto, Colin Caprani, Colin Carroll, Nathaniel Burbank, Michael Osthege, Rémi Louf, Clive Edelsten, Henri Wallen, Hugo Botha, Vinh Nguyen, Raul Maldonado, Marcin Elantkowski, Adam C. Smith, Will Kurt, Andrew Moskowitz, Hector Munoz, Marco Gorelli, Simon Kessell, Bradley Rode, Patrick Kelley, Rick Anderson, Casper de Bruin, Philippe Labonde, Michael Hankin, Cameron Smith, Tomáš Frýda, Ryan Wesslen, Andreas Netti, Riley King, Yoshiyuki Hamajima, Sven De Maeyer, Michael DeCrescenzo, Fergal M, Mason Yahr, Naoya Kanai, Steven Rowland, Aubrey Clayton, Jeannine Sue, Omri Har Shemesh, Scott Anthony Robson, David Haas, Robert Yolken, Or Duek, Pavel Dusek, Paul Cox, Trey Causey, Andreas Kröpelin, Raphaël R, Nicolas Rode, Gabriel Stechschulte, and Arkady.Visit https://www.patreon.com/learnbayesstats to unlock exclusive Bayesian swag ;)Links from the show:Sameer’s website: https://skdeshpande91.github.io/Sameer on GitHub: https://github.com/skdeshpande91Sameer on Twitter: https://twitter.com/skdeshpande91 Sameer on Google Scholar: https://scholar.google.com/citations?user=coVrnWIAAAAJ&hl=enLBS #50 Ta(l)king Risks & Embracing Uncertainty, with David Spiegelhalter: https://learnbayesstats.com/episode/50-talking-risks-embracing-uncertainty-david-spiegelhalter/LBS #51 Bernoulli’s Fallacy & the Crisis of Modern Science, with Aubrey Clayton: https://learnbayesstats.com/episode/51-bernoullis-fallacy-crisis-modern-science-aubrey-clayton/LBS #58 Bayesian Modeling and Computation, with Osvaldo Martin, Ravin Kumar and Junpeng Lao: https://learnbayesstats.com/episode/58-bayesian-modeling-computation-osvaldo-martin-ravin-kumar-junpeng-lao/Book Bayesian Modeling and Computation in Python: https://bayesiancomputationbook.com/welcome.htmlLBS #39 Survival Models & Biostatistics for Cancer Research, with Jacki Buros: https://learnbayesstats.com/episode/39-survival-models-biostatistics-cancer-research-jacki-buros/Original BART paper (Chipman, George, and McCulloch 2010): https://doi.org/10.1214/09-AOAS285Hill (2011) on BART in causal inference: https://doi.org/10.1198/jcgs.2010.08162Hahn, Murray, and Carvalho on Bayesian causal forests: https://doi.org/10.1214/19-BA1195Main BART package in R: https://cran.r-project.org/web/packages/BART/index.htmldbart R package: https://cran.r-project.org/web/packages/dbarts/index.htmlSameer’s own re-implementation of BART: https://github.com/skdeshpande91/flexBARTAbstractby Christoph BambergIn episode 80, Sameer Deshpande, assistant professor of Statistics at the University of Wisconsin-Madison is our guest. He had a passion for math from a young age. And got into Bayesian statistics at university, teaching statistics now himself. We talk about the intricacies of teaching bayesian statistics, such as helping students accept that there are no objective answers. Sameer’s current work focuses on Bayesian Additive Regression Trees (BARTs). He also works on prior specification, and numerous cool applied projects, for example on the effects of playing American football as an adolescent and its effects for later healthWe primarily talk about BARTs as a way of approximating complex functions by using a collection of step functions. They work off the shelf pretty well and can be applied to various models such as survival models, linear models, and smooth models. BARTs are somewhat analogous to splines and can capture trajectories well over time. However, they are also a bit like a black box making them hard to interpret.We further touch upon some of his work on practical problems, such as how cognitive processes change over time or models of baseball empires’ decision making.TranscriptPlease note that the following transcript was generated automatically and may therefore contain errors. Feel free to reach out if you're willing to correct them.