
Data Engineering Podcast
Building Linked Data Products With JSON-LD
Podcast summary created with Snipd AI
Quick takeaways
- Linked data products enable high-integrity, trusted data management, facilitating seamless integration and automatic inference.
- JSON-LD simplifies the representation of RDF triples as JSON objects, enabling data interoperability across domains.
- Graph-native databases for knowledge graphs simplify data engineering efforts, providing advanced graph-based analytics and accelerating data cataloging processes.
Deep dives
The Advantages of Using Linked Data Products
Linked data products allow for a shift in focus from apps to data, enabling high-integrity, trusted data management. It facilitates seamless integration of data across domains and enables automatic inference and merging of data. Machine learning and AI can leverage linked data for better understanding and analysis. The adoption of linked data products, especially graph-based solutions, supports interoperability, reusability, and scalability of data.
The Role of JSON LD in Linked Data
JSON LD provides a user-friendly and familiar way of working with linked data by using RDF under the hood. It simplifies the representation of RDF triples as JSON objects, making it easy for developers to work with JSON while still embracing semantic web concepts. The adoption of JSON LD has seen significant usage in embedding linked data in web pages, regulation compliance, and AI applications. It enables data interoperability across domains, both internally within organizations and externally across boundaries.
Benefits of Native Graph Data Storage in Knowledge Graphs
Using graph-native databases for knowledge graphs simplifies data engineering efforts by eliminating the need for complex data transformations and enables scalable querying across multiple datasets. Graph data provides a superset of capabilities compared to traditional relational databases, combining the simplicity of document databases with advanced graph-based analytics. Graph data can be both flattened to relational structures for traditional analytics and expanded to leverage the full power of graph querying. The adoption of graph as a system of record accelerates data cataloging, data discovery, and schema enforcement processes.
Embedding Contextual Awareness into Data Modeling
One of the main ideas discussed in this podcast episode is the importance of embedding contextual awareness into data modeling exercises. The speaker emphasizes the need to make contextual awareness native to the data itself, rather than treating it as an afterthought or something imposed upon the data. In the context of JSON LD, the speaker mentions the use of special keywords like ID and app type to assign global identifiers and class hierarchies to the data. These features allow for mapping different data items together and adding powerful capabilities to the data. The mapping is done through the use of special keys, specifically the 'at context' field, which allows for mapping key names to global identifiers, providing a consistent and standardized vocabulary for the data.
Architectural Approaches for Performance and Data Integration
The second main idea discussed in this podcast episode is the architectural approaches for performance and data integration in the world of linked data. The speaker highlights the importance of addressing the lookup cost and performance challenges associated with handling the attributes and properties of linked data. They mention the use of ELT approaches and data transformation techniques to ensure consistency and coherence in data integration. The idea of breaking up the database into two pieces - a server for updates and a server for queries - is mentioned as an architectural approach to achieve horizontal scalability and to push compute layers to the edge for better performance. By leveraging distributed computing and pushing computationally intense algorithms to the edge, organizations can optimize the performance of their linked data systems. The speaker also mentions the importance of gradually adopting linked data concepts and transitioning from rectangular data models to graph-based representations, such as JSON LD, to enable greater interoperability and take advantage of the emergent properties of linked data.
Summary
A significant amount of time in data engineering is dedicated to building connections and semantic meaning around pieces of information. Linked data technologies provide a means of tightly coupling metadata with raw information. In this episode Brian Platz explains how JSON-LD can be used as a shared representation of linked data for building semantic data products.
Announcements
- Hello and welcome to the Data Engineering Podcast, the show about modern data management
- This episode is brought to you by Datafold – a testing automation platform for data engineers that finds data quality issues before the code and data are deployed to production. Datafold leverages data-diffing to compare production and development environments and column-level lineage to show you the exact impact of every code change on data, metrics, and BI tools, keeping your team productive and stakeholders happy. Datafold integrates with dbt, the modern data stack, and seamlessly plugs in your data CI for team-wide and automated testing. If you are migrating to a modern data stack, Datafold can also help you automate data and code validation to speed up the migration. Learn more about Datafold by visiting dataengineeringpodcast.com/datafold
- Introducing RudderStack Profiles. RudderStack Profiles takes the SaaS guesswork and SQL grunt work out of building complete customer profiles so you can quickly ship actionable, enriched data to every downstream team. You specify the customer traits, then Profiles runs the joins and computations for you to create complete customer profiles. Get all of the details and try the new product today at dataengineeringpodcast.com/rudderstack
- You shouldn't have to throw away the database to build with fast-changing data. You should be able to keep the familiarity of SQL and the proven architecture of cloud warehouses, but swap the decades-old batch computation model for an efficient incremental engine to get complex queries that are always up-to-date. With Materialize, you can! It’s the only true SQL streaming database built from the ground up to meet the needs of modern data products. Whether it’s real-time dashboarding and analytics, personalization and segmentation or automation and alerting, Materialize gives you the ability to work with fresh, correct, and scalable results — all in a familiar SQL interface. Go to dataengineeringpodcast.com/materialize today to get 2 weeks free!
- If you’re a data person, you probably have to jump between different tools to run queries, build visualizations, write Python, and send around a lot of spreadsheets and CSV files. Hex brings everything together. Its powerful notebook UI lets you analyze data in SQL, Python, or no-code, in any combination, and work together with live multiplayer and version control. And now, Hex’s magical AI tools can generate queries and code, create visualizations, and even kickstart a whole analysis for you – all from natural language prompts. It’s like having an analytics co-pilot built right into where you’re already doing your work. Then, when you’re ready to share, you can use Hex’s drag-and-drop app builder to configure beautiful reports or dashboards that anyone can use. Join the hundreds of data teams like Notion, AllTrails, Loom, Mixpanel and Algolia using Hex every day to make their work more impactful. Sign up today at dataengineeringpodcast.com/hex to get a 30-day free trial of the Hex Team plan!
- Your host is Tobias Macey and today I'm interviewing Brian Platz about using JSON-LD for building linked-data products
Interview
- Introduction
- How did you get involved in the area of data management?
- Can you describe what the term "linked data product" means and some examples of when you might build one?
- What is the overlap between knowledge graphs and "linked data products"?
- What is JSON-LD?
- What are the domains in which it is typically used?
- How does it assist in developing linked data products?
- what are the characteristics that distinguish a knowledge graph from
- What are the layers/stages of applications and data that can/should incorporate JSON-LD as the representation for records and events?
- What is the level of native support/compatibiliity that you see for JSON-LD in data systems?
- What are the modeling exercises that are necessary to ensure useful and appropriate linkages of different records within and between products and organizations?
- Can you describe the workflow for building autonomous linkages across data assets that are modelled as JSON-LD?
- What are the most interesting, innovative, or unexpected ways that you have seen JSON-LD used for data workflows?
- What are the most interesting, unexpected, or challenging lessons that you have learned while working on linked data products?
- When is JSON-LD the wrong choice?
- What are the future directions that you would like to see for JSON-LD and linked data in the data ecosystem?
Contact Info
Parting Question
- From your perspective, what is the biggest gap in the tooling or technology for data management today?
Closing Announcements
- Thank you for listening! Don't forget to check out our other shows. Podcast.__init__ covers the Python language, its community, and the innovative ways it is being used. The Machine Learning Podcast helps you go from idea to production with machine learning.
- Visit the site to subscribe to the show, sign up for the mailing list, and read the show notes.
- If you've learned something or tried out a project from the show then tell us about it! Email hosts@dataengineeringpodcast.com) with your story.
- To help other people find the show please leave a review on Apple Podcasts and tell your friends and co-workers
Links
- Fluree
- JSON-LD
- Knowledge Graph
- Adjacency List
- RDF == Resource Description Framework
- Semantic Web
- Open Graph
- Schema.org
- RDF Triple
- IDMP == Identification of Medicinal Products
- FIBO == Financial Industry Business Ontology
- OWL Standard
- NP-Hard
- Forward-Chaining Rules
- SHACL == Shapes Constraint Language)
- Zero Knowledge Cryptography
- Turtle Serialization
The intro and outro music is from The Hug by The Freak Fandango Orchestra / CC BY-SA
Sponsored By:
- Materialize: 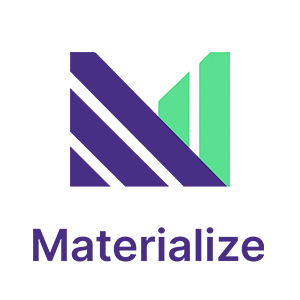 You shouldn't have to throw away the database to build with fast-changing data. Keep the familiar SQL, keep the proven architecture of cloud warehouses, but swap the decades-old batch computation model for an efficient incremental engine to get complex queries that are always up-to-date. That is Materialize, the only true SQL streaming database built from the ground up to meet the needs of modern data products: Fresh, Correct, Scalable — all in a familiar SQL UI. Built on Timely Dataflow and Differential Dataflow, open source frameworks created by cofounder Frank McSherry at Microsoft Research, Materialize is trusted by data and engineering teams at Ramp, Pluralsight, Onward and more to build real-time data products without the cost, complexity, and development time of stream processing. Go to [materialize.com](https://materialize.com/register/?utm_source=depodcast&utm_medium=paid&utm_campaign=early-access) today and get 2 weeks free!
- Hex: 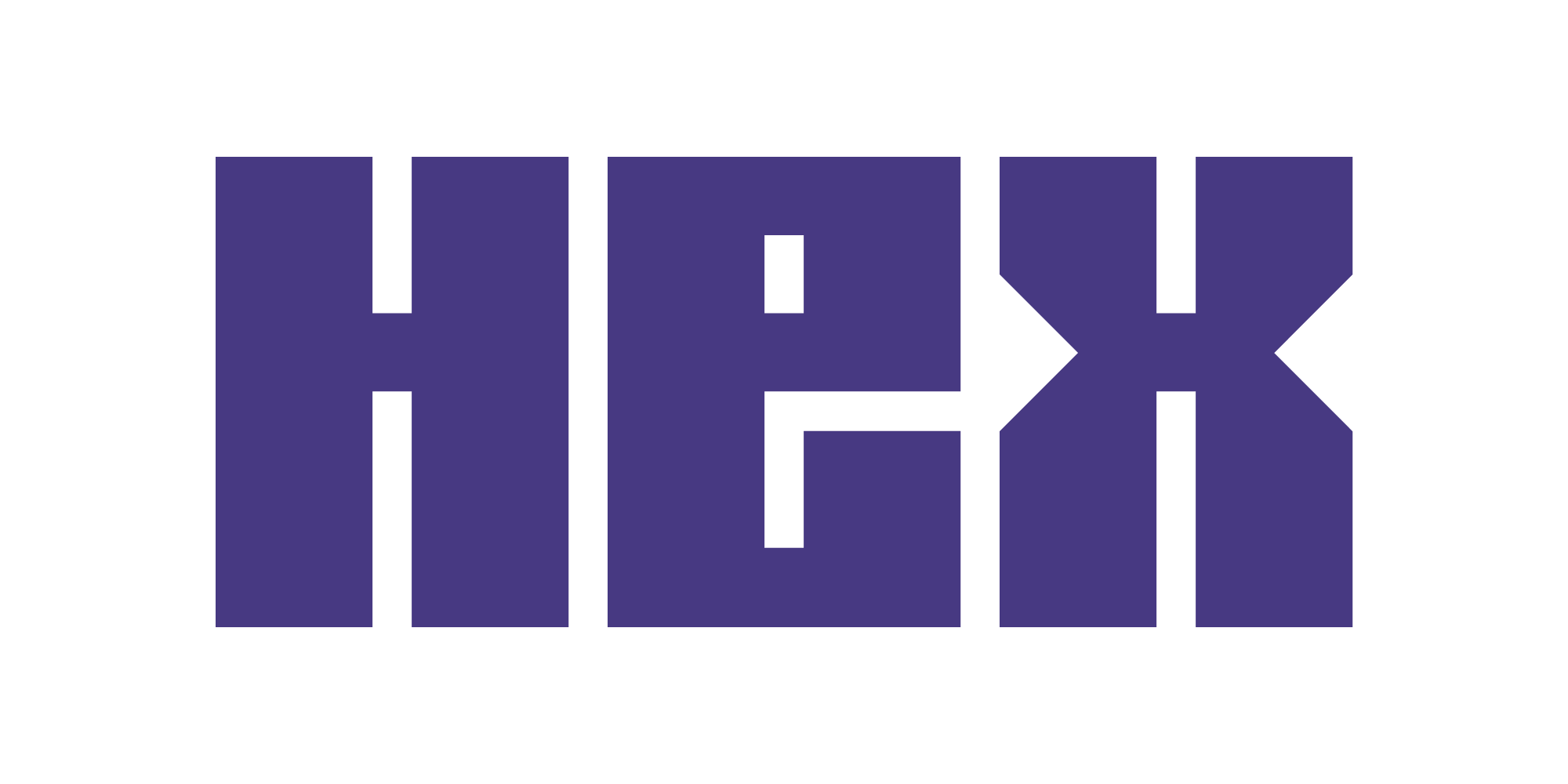 Hex is a collaborative workspace for data science and analytics. A single place for teams to explore, transform, and visualize data into beautiful interactive reports. Use SQL, Python, R, no-code and AI to find and share insights across your organization. Empower everyone in an organization to make an impact with data. Sign up today at dataengineeringpodcast.com/hex to get a 30-day free trial of the Hex Team plan!
- Rudderstack:  Introducing RudderStack Profiles. RudderStack Profiles takes the SaaS guesswork and SQL grunt work out of building complete customer profiles so you can quickly ship actionable, enriched data to every downstream team. You specify the customer traits, then Profiles runs the joins and computations for you to create complete customer profiles. Get all of the details and try the new product today at [dataengineeringpodcast.com/rudderstack](https://www.dataengineeringpodcast.com/rudderstack)
- Datafold: 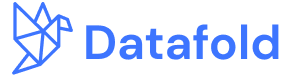 This episode is brought to you by Datafold – a testing automation platform for data engineers that finds data quality issues before the code and data are deployed to production. Datafold leverages data-diffing to compare production and development environments and column-level lineage to show you the exact impact of every code change on data, metrics, and BI tools, keeping your team productive and stakeholders happy. Datafold integrates with dbt, the modern data stack, and seamlessly plugs in your data CI for team-wide and automated testing. If you are migrating to a modern data stack, Datafold can also help you automate data and code validation to speed up the migration. Learn more about Datafold by visiting [dataengineeringpodcast.com/datafold](https://www.dataengineeringpodcast.com/datafold) today!