
Data Engineering Podcast
Powering Vector Search With Real Time And Incremental Vector Indexes
Podcast summary created with Snipd AI
Quick takeaways
- Vector search is used for recommendation systems, semantic matching, and chatbots in AI applications.
- Incremental indexing and metadata filtering are major challenges in vector search.
- Adding vector indexes to existing databases is often easier than building a new database for vector search.
- OLAP databases with vector indexes are a promising approach for incorporating vector search capabilities.
- Understanding the meaning of dimensions within vectors and standardizing tools for querying vector-based results are ongoing challenges.
- Data engineering and data info teams typically manage vector capabilities as part of the core data stack.
Deep dives
Vector Search and its Applications
Vector search is a two-stage process that involves turning an unstructured search problem into a structured search by projecting the input into a vector space. This structured search involves finding the nearest neighbors to the input vector in the vector space. Vector search has been traditionally used for recommendation systems and semantic search, where it finds similar items or documents based on their embeddings. With the rise of large language models, vector search is also being used in chatbots and AI applications for semantic matching of user queries to relevant documents. However, vector search is not suitable for cases where the problem cannot be modeled in a vector space or when the cost of using vectors outweighs the benefits.
Challenges in Vector Search
Two major challenges in vector search are incremental indexing and metadata filtering. Incremental indexing, especially in real-time or continuous streaming scenarios, is difficult because continuously adding new vectors to the index can degrade its performance. Similarly, metadata filtering, which involves combining vector search with other filtering criteria like location or timestamp, presents challenges in maintaining the fast lookup capabilities of the index. These challenges require thoughtful consideration and innovation to ensure efficient and effective vector search.
Vector Search Infrastructure and Decision Making
When considering vector search capabilities, it is important to evaluate whether a dedicated vector database is necessary or if vector indexes can be added to existing database technologies. It is often easier to add vector indexes to an existing database as opposed to building a new database specifically for vector search. However, the decision should be made based on the specific requirements of the use case and the necessary trade-offs in terms of consistency, durability, latency, and access control. Organizations should also be cautious about unintentionally building their own databases instead of focusing on vector search capabilities alone. Proper understanding of the difference between vector search and a vector database is crucial for making informed decisions in utilizing this technology.
OLAP databases with vector indexes as a way forward
OLAP databases with vector indexes are seen as a promising approach for incorporating vector search capabilities. OLAP databases prioritize analytics and separating them from transactional databases to avoid performance issues. The idea is that vector databases will resemble OLAP databases, and OLAP databases will incorporate vector search. The preferred data stack is to have an OLTP database paired with an OLAP database with vector search capabilities.
Treating vectors as a new column and challenges of data modeling
Vectors are treated as a new column in the database, similar to any other column with an index. One column can represent a vector, and different models may generate multiple vector columns for different semantic relevance definitions. The vector search can be conducted using SQL-like queries that combine metadata filters and vector searches. However, understanding the meaning of dimensions within vectors is still an unsolved problem. The ecosystem for querying and interpreting vector-based results is still evolving and lacks standardized tools and libraries.
Data ownership and system architecture for vector capabilities
Teams that own and manage vector capabilities within their data stack typically include data engineering and data info teams. These teams handle the implementation and maintenance of vector search, while other teams, such as data scientists and ML engineers, utilize the capabilities for their specific needs. Vector search is considered to be part of the core data stack, integrated with other components like transactional databases. However, incorporating vector search capabilities may vary depending on the specific use case and organization.
Challenges and potential applications of vector indexing
The future potential applications of vector indexing depend on the quality and value of the upstream embeddings used. As embedding technologies improve, new valuable use cases for vector search emerge. Currently, potential applications include chatbots, natural language prompt search queries, and multi-modal analysis. However, the process of transforming data into meaningful vectors and managing vector quality and anomalies is still a challenge that requires further research and development.
Need for unified systems and real-time analytics
Unified systems that seamlessly integrate vector search and metadata filtering are seen as the ideal path forward. The distinction between these components should be synthetic, enabling users to write queries that involve both vector filters and metadata filters. Currently, real-time analytics using vector search is an area that lags behind. Building real-time systems for vector search is critical to prevent abuse of other systems like Elasticsearch. Furthermore, the availability of first-class tools and primitives for ML and AI applications within data management platforms is a major gap that needs to be addressed.
Data quality challenges and anomaly detection in vector spaces
Ensuring data quality in vector spaces presents unique challenges. In vector spaces, all values are valid by definition, making it difficult to identify nonsensical data. However, the concept of anomaly detection is well-suited for vector spaces, where anomalies are identified as vectors that are far from other vectors in the space. An interesting feature is the ability to flag anomalous vectors at the time of ingestion, providing valuable insights for applications like fraud detection. However, research on anomaly detection and overall data quality management in vector spaces is still limited.
Closing the gap in real-time analytics and ML primitives
The current gap in real-time analytics needs to be addressed to provide efficient and usable systems. In addition, data management platforms lack first-class primitives for ML and AI applications. These platforms must offer features for versioning, tracking, AP testing, and time-traveling ML features. The lifecycle management of vectors, as discussed, is just one example of the broader need for advanced primitives for ML and AI in data management platforms.
Summary
The adoption of vector search and indexing is increasing, driven by the application of large language models. OLAP databases with vector indexes are seen as a viable approach to incorporating vector search capabilities. Vector search is treated as a new column in the database, allowing for queries combining metadata filters and vector searches. However, challenges persist in understanding the meaning of vector dimensions and developing standardized tools for working with vectors. Data engineering and data info teams typically own and manage vector capabilities, which should be integrated into the core data stack. The future potential of vector indexing depends on the quality of the embeddings used. Unified systems that integrate vector search and metadata filtering are crucial. Real-time analytics and ML primitives in data management platforms require significant improvements. Data quality management in vector spaces and anomaly detection are ongoing challenges.
Summary
The rapid growth of machine learning, especially large language models, have led to a commensurate growth in the need to store and compare vectors. In this episode Louis Brandy discusses the applications for vector search capabilities both in and outside of AI, as well as the challenges of maintaining real-time indexes of vector data.
Announcements
- Hello and welcome to the Data Engineering Podcast, the show about modern data management
- Introducing RudderStack Profiles. RudderStack Profiles takes the SaaS guesswork and SQL grunt work out of building complete customer profiles so you can quickly ship actionable, enriched data to every downstream team. You specify the customer traits, then Profiles runs the joins and computations for you to create complete customer profiles. Get all of the details and try the new product today at dataengineeringpodcast.com/rudderstack
- This episode is brought to you by Datafold – a testing automation platform for data engineers that finds data quality issues before the code and data are deployed to production. Datafold leverages data-diffing to compare production and development environments and column-level lineage to show you the exact impact of every code change on data, metrics, and BI tools, keeping your team productive and stakeholders happy. Datafold integrates with dbt, the modern data stack, and seamlessly plugs in your data CI for team-wide and automated testing. If you are migrating to a modern data stack, Datafold can also help you automate data and code validation to speed up the migration. Learn more about Datafold by visiting dataengineeringpodcast.com/datafold
- You shouldn't have to throw away the database to build with fast-changing data. You should be able to keep the familiarity of SQL and the proven architecture of cloud warehouses, but swap the decades-old batch computation model for an efficient incremental engine to get complex queries that are always up-to-date. With Materialize, you can! It’s the only true SQL streaming database built from the ground up to meet the needs of modern data products. Whether it’s real-time dashboarding and analytics, personalization and segmentation or automation and alerting, Materialize gives you the ability to work with fresh, correct, and scalable results — all in a familiar SQL interface. Go to dataengineeringpodcast.com/materialize today to get 2 weeks free!
- If you’re a data person, you probably have to jump between different tools to run queries, build visualizations, write Python, and send around a lot of spreadsheets and CSV files. Hex brings everything together. Its powerful notebook UI lets you analyze data in SQL, Python, or no-code, in any combination, and work together with live multiplayer and version control. And now, Hex’s magical AI tools can generate queries and code, create visualizations, and even kickstart a whole analysis for you – all from natural language prompts. It’s like having an analytics co-pilot built right into where you’re already doing your work. Then, when you’re ready to share, you can use Hex’s drag-and-drop app builder to configure beautiful reports or dashboards that anyone can use. Join the hundreds of data teams like Notion, AllTrails, Loom, Mixpanel and Algolia using Hex every day to make their work more impactful. Sign up today at dataengineeringpodcast.com/hex to get a 30-day free trial of the Hex Team plan!
- Your host is Tobias Macey and today I'm interviewing Louis Brandy about building vector indexes in real-time for analytics and AI applications
Interview
- Introduction
- How did you get involved in the area of data management?
- Can you describe what vector search is and how it differs from other search technologies?
- What are the technical challenges related to providing vector search?
- What are the applications for vector search that merit the added complexity?
- Vector databases have been gaining a lot of attention recently with the proliferation of LLM applications. Is a dedicated database technology required to support vector indexes/vector search queries?
- What are the use cases for native vector data types that are separate from AI?
- With the increasing usage of vectors for data and AI/ML applications, who do you typically see as the owner of that problem space? (e.g. data engineers, ML engineers, data scientists, etc.)
- For teams who are investing in vector search, what are the architectural considerations that they need to be aware of?
- How does it impact the data pipeline strategies/topologies used?
- What are the complexities that need to be addressed when updating vector data in a real-time/streaming fashion?
- How does that influence the client strategies that are querying that data?
- What are the most interesting, innovative, or unexpected ways that you have seen vector search used?
- What are the most interesting, unexpected, or challenging lessons that you have learned while working on vector search applications?
- When is vector search the wrong choice?
- What do you see as future potential applications for vector indexes/vector search?
Contact Info
Parting Question
- From your perspective, what is the biggest gap in the tooling or technology for data management today?
Closing Announcements
- Thank you for listening! Don't forget to check out our other shows. The Machine Learning Podcast helps you go from idea to production with machine learning. Podcast.__init__ covers the Python language, its community, and the innovative ways it is being used.
- Visit the site to subscribe to the show, sign up for the mailing list, and read the show notes.
- If you've learned something or tried out a project from the show then tell us about it! Email hosts@dataengineeringpodcast.com) with your story.
- To help other people find the show please leave a review on Apple Podcasts and tell your friends and co-workers
Links
- Rockset
- Vector Index
- Vector Search
- Vector Space
- Euclidean Distance
- OLAP == Online Analytical Processing
- OLTP == Online Transaction Processing
The intro and outro music is from The Hug by The Freak Fandango Orchestra / CC BY-SA
Sponsored By:
- Rudderstack:  Introducing RudderStack Profiles. RudderStack Profiles takes the SaaS guesswork and SQL grunt work out of building complete customer profiles so you can quickly ship actionable, enriched data to every downstream team. You specify the customer traits, then Profiles runs the joins and computations for you to create complete customer profiles. Get all of the details and try the new product today at [dataengineeringpodcast.com/rudderstack](https://www.dataengineeringpodcast.com/rudderstack)
- Materialize: 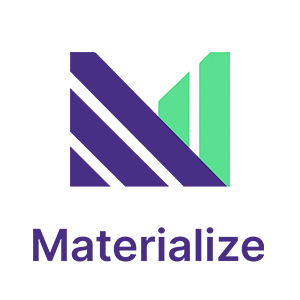 You shouldn't have to throw away the database to build with fast-changing data. Keep the familiar SQL, keep the proven architecture of cloud warehouses, but swap the decades-old batch computation model for an efficient incremental engine to get complex queries that are always up-to-date. That is Materialize, the only true SQL streaming database built from the ground up to meet the needs of modern data products: Fresh, Correct, Scalable — all in a familiar SQL UI. Built on Timely Dataflow and Differential Dataflow, open source frameworks created by cofounder Frank McSherry at Microsoft Research, Materialize is trusted by data and engineering teams at Ramp, Pluralsight, Onward and more to build real-time data products without the cost, complexity, and development time of stream processing. Go to [materialize.com](https://materialize.com/register/?utm_source=depodcast&utm_medium=paid&utm_campaign=early-access) today and get 2 weeks free!
- Hex: 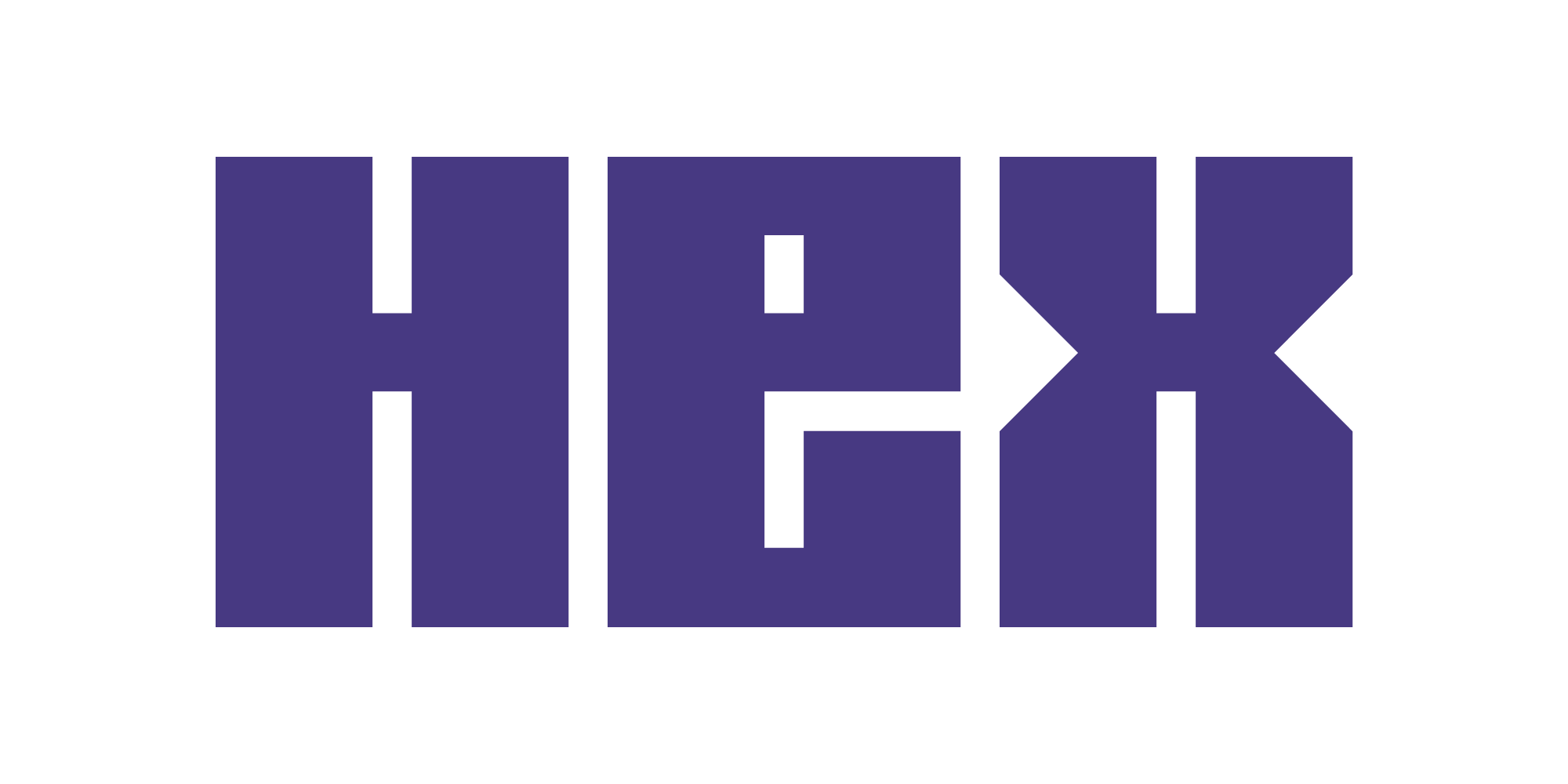 Hex is a collaborative workspace for data science and analytics. A single place for teams to explore, transform, and visualize data into beautiful interactive reports. Use SQL, Python, R, no-code and AI to find and share insights across your organization. Empower everyone in an organization to make an impact with data. Sign up today at dataengineeringpodcast.com/hex to get a 30-day free trial of the Hex Team plan!
- Datafold: 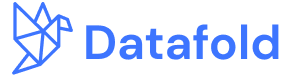 This episode is brought to you by Datafold – a testing automation platform for data engineers that finds data quality issues before the code and data are deployed to production. Datafold leverages data-diffing to compare production and development environments and column-level lineage to show you the exact impact of every code change on data, metrics, and BI tools, keeping your team productive and stakeholders happy. Datafold integrates with dbt, the modern data stack, and seamlessly plugs in your data CI for team-wide and automated testing. If you are migrating to a modern data stack, Datafold can also help you automate data and code validation to speed up the migration. Learn more about Datafold by visiting [dataengineeringpodcast.com/datafold](https://www.dataengineeringpodcast.com/datafold) today!