
Data Engineering Podcast
Reconciling The Data In Your Databases With Datafold
Episode guests
Podcast summary created with Snipd AI
Quick takeaways
- Reconciliation of data across databases is crucial for ensuring consistency and reliability in data projects.
- Understanding data differences and challenges in various workloads like code changes and data migration is essential for successful data management.
- Optimizing data quality workflows through tools like Datafold can improve visibility, testing capabilities, and streamline data reconciliation processes.
- Data reconciliation across diverse database systems involves overcoming challenges like schema changes, event ordering, and data type discrepancies.
- Datafold's focus on minimizing data transfer costs and improving performance enhances data reconciliation efficiency for enterprise teams.
- Future developments in data management tools like Datafold include NoSQL support, performance enhancements, and continuous replication monitoring to meet evolving data needs.
Deep dives
Data Lakes Complexity and Starburst Analytics Platform
Data lakes are complex for data engineers striving to build high-quality data workflows. Starburst offers a SQL analytics platform for petabyte-scale data analysis at lower costs. It supports Apache iceberg, Delta Lake, and Hoodie on an open architecture for data adaptability and flexibility.
Gleb Majansky's Data Experience and Changes Over 10 Years
Gleb Majansky shares his rich experience spanning a decade in data engineering. He emphasizes how data management challenges have evolved through his work across companies like Autodesk, Lyft, and a startup. The evolution of data warehouses, scalability, and data integration has shaped the modern data platform landscape.
Data Engineering Bottlenecks and Shift to Data Engineering Focus
Gleb discusses the bottlenecks in data engineering workflows, emphasizing the significance of clean, reliable, and timely data processing. He shares his transition from practical pipeline building to data tool development and product management at Lyft. The focus on improving data workflows to address complexity and quality challenges drives his passion.
Data Reconciliation Importance and Workload Dimensions
Reconciliation is a critical aspect of data quality, emphasizing understanding data differences across databases. Gleb highlights reconciliation challenges in multiple workloads, such as code changes and data migration between database systems. The importance of comparing data accurately in different environments for consistency and reliability is crucial for successful data projects.
Challenges in Database Environment Reconciliation and Solutions
Reconciling data across database systems poses challenges like schema changes, event ordering, and handling transactions. Gleb explores the difficulties in comparing data types and ordering across diverse database engines. Solutions include hashing data for efficient comparison and resolving discrepancies to ensure data accuracy across systems.
Data Fold Overview, Goals for Tooling, and Future Plans
Data Fold optimizes data quality workflows by providing visibility and testing capabilities. Gleb outlines the distinction between the open-source Data Diff tool and the cloud product, emphasizing individual vs. enterprise usage. Future plans include NoSQL support, performance enhancements, and continuous replication monitoring to streamline data reconciliation processes.
Innovative Usage of Data Fold for Compliance and Auditing
Data Fold's application extends to compliance and auditing for financial services, ensuring data correctness and integrity during audits. Gleb highlights the value of data reconciliation for compliance and auditing purposes, demonstrating the importance of accurate data handling in high compliance environments.
Product Design Challenges and User Experience Improvements
Product design challenges revolve around building tools for data practitioners with varying needs and industries. Gleb discusses performance optimization and innovative UX features like streaming results and sampling to simplify data comparison workflows. Data Fold focuses on providing essential information and empowering users to take action for data reconciliation.
Network Cost Management and Data Transfer Optimization
Data Fold focuses on minimizing data transfer costs and optimizing performance by sending metadata and selective data chunks. Users control the amount of data transferred and can manage costs effectively. The platform prioritizes reducing network overhead while ensuring efficient data reconciliation.
Data Fold Tool Selection Criteria and Use Cases
Data Fold is suitable for teams requiring enterprise-level data reconciliation support. Gleb differentiates between the open-source Data Diff tool for individual practitioners and the cloud product for team collaboration and large-scale data comparison. The tool is ideal for facilitating data reconciliation workflows and ensuring data quality and consistency.
Future Directions in Data Reconciliation and Data Quality Tools
Data Fold's future roadmap includes NoSQL support, broadening database compatibility, and continuous performance improvements. Gleb discusses the industry trend towards AI applications and the data context necessary for accurate AI model execution. Enhancements in data tooling and AI support signify promising developments in data management practices.
Data Fold Closing Thoughts on Data Management Software Gaps
Gleb discusses the potential gaps in data management tooling relative to software engineering support, emphasizing the need for advanced tools to aid data practitioners. Content creation tools like GitHub and GitLab inspire innovative solutions for data engineers. Data Fold aims to fill these gaps by providing advanced data reconciliation capabilities and improving data management workflows.
Conclusion and Inviting to Other Podcasts
Gleb shares insights on the evolving data landscape and the critical role of data reconciliation in managing modern data challenges. Stay tuned for more episodes covering data engineering, Python, machine learning, and AI topics to gain further insights and deepen your understanding of data technologies.
Summary
A significant portion of data workflows involve storing and processing information in database engines. Validating that the information is stored and processed correctly can be complex and time-consuming, especially when the source and destination speak different dialects of SQL. In this episode Gleb Mezhanskiy, founder and CEO of Datafold, discusses the different error conditions and solutions that you need to know about to ensure the accuracy of your data.
Announcements
- Hello and welcome to the Data Engineering Podcast, the show about modern data management
- Dagster offers a new approach to building and running data platforms and data pipelines. It is an open-source, cloud-native orchestrator for the whole development lifecycle, with integrated lineage and observability, a declarative programming model, and best-in-class testability. Your team can get up and running in minutes thanks to Dagster Cloud, an enterprise-class hosted solution that offers serverless and hybrid deployments, enhanced security, and on-demand ephemeral test deployments. Go to dataengineeringpodcast.com/dagster today to get started. Your first 30 days are free!
- Data lakes are notoriously complex. For data engineers who battle to build and scale high quality data workflows on the data lake, Starburst powers petabyte-scale SQL analytics fast, at a fraction of the cost of traditional methods, so that you can meet all your data needs ranging from AI to data applications to complete analytics. Trusted by teams of all sizes, including Comcast and Doordash, Starburst is a data lake analytics platform that delivers the adaptability and flexibility a lakehouse ecosystem promises. And Starburst does all of this on an open architecture with first-class support for Apache Iceberg, Delta Lake and Hudi, so you always maintain ownership of your data. Want to see Starburst in action? Go to dataengineeringpodcast.com/starburst and get $500 in credits to try Starburst Galaxy today, the easiest and fastest way to get started using Trino.
- Join us at the top event for the global data community, Data Council Austin. From March 26-28th 2024, we'll play host to hundreds of attendees, 100 top speakers and dozens of startups that are advancing data science, engineering and AI. Data Council attendees are amazing founders, data scientists, lead engineers, CTOs, heads of data, investors and community organizers who are all working together to build the future of data and sharing their insights and learnings through deeply technical talks. As a listener to the Data Engineering Podcast you can get a special discount off regular priced and late bird tickets by using the promo code dataengpod20. Don't miss out on our only event this year! Visit dataengineeringpodcast.com/data-council and use code dataengpod20 to register today!
- Your host is Tobias Macey and today I'm welcoming back Gleb Mezhanskiy to talk about how to reconcile data in database environments
Interview
- Introduction
- How did you get involved in the area of data management?
- Can you start by outlining some of the situations where reconciling data between databases is needed?
- What are examples of the error conditions that you are likely to run into when duplicating information between database engines?
- When these errors do occur, what are some of the problems that they can cause?
- When teams are replicating data between database engines, what are some of the common patterns for managing those flows?
- How does that change between continual and one-time replication?
- What are some of the steps involved in verifying the integrity of data replication between database engines?
- If the source or destination isn't a traditional database engine (e.g. data lakehouse) how does that change the work involved in verifying the success of the replication?
- What are the challenges of validating and reconciling data?
- Sheer scale and cost of pulling data out, have to do in-place
- Performance. Pushing databases to the limit, especially hard for OLTP and legacy
- Cross-database compatibilty
- Data types
- What are the most interesting, innovative, or unexpected ways that you have seen Datafold/data-diff used in the context of cross-database validation?
- What are the most interesting, unexpected, or challenging lessons that you have learned while working on Datafold?
- When is Datafold/data-diff the wrong choice?
- What do you have planned for the future of Datafold?
Contact Info
Parting Question
- From your perspective, what is the biggest gap in the tooling or technology for data management today?
Closing Announcements
- Thank you for listening! Don't forget to check out our other shows. Podcast.__init__ covers the Python language, its community, and the innovative ways it is being used. The Machine Learning Podcast helps you go from idea to production with machine learning.
- Visit the site to subscribe to the show, sign up for the mailing list, and read the show notes.
- If you've learned something or tried out a project from the show then tell us about it! Email hosts@dataengineeringpodcast.com) with your story.
Links
- Datafold
- data-diff
- Hive
- Presto
- Spark
- SAP HANA
- Change Data Capture
- Nessie
- LakeFS
- Iceberg Tables
- SQLGlot
- Trino
- GitHub Copilot
The intro and outro music is from The Hug by The Freak Fandango Orchestra / CC BY-SA
Sponsored By:
- Starburst: 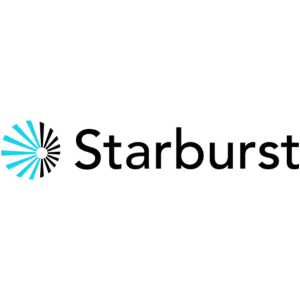 This episode is brought to you by Starburst - a data lake analytics platform for data engineers who are battling to build and scale high quality data pipelines on the data lake. Powered by Trino, Starburst runs petabyte-scale SQL analytics fast at a fraction of the cost of traditional methods, helping you meet all your data needs ranging from AI/ML workloads to data applications to complete analytics. Trusted by the teams at Comcast and Doordash, Starburst delivers the adaptability and flexibility a lakehouse ecosystem promises, while providing a single point of access for your data and all your data governance allowing you to discover, transform, govern, and secure all in one place. Starburst does all of this on an open architecture with first-class support for Apache Iceberg, Delta Lake and Hudi, so you always maintain ownership of your data. Want to see Starburst in action? Try Starburst Galaxy today, the easiest and fastest way to get started using Trino, and get $500 of credits free. [dataengineeringpodcast.com/starburst](https://www.dataengineeringpodcast.com/starburst)
- Dagster: 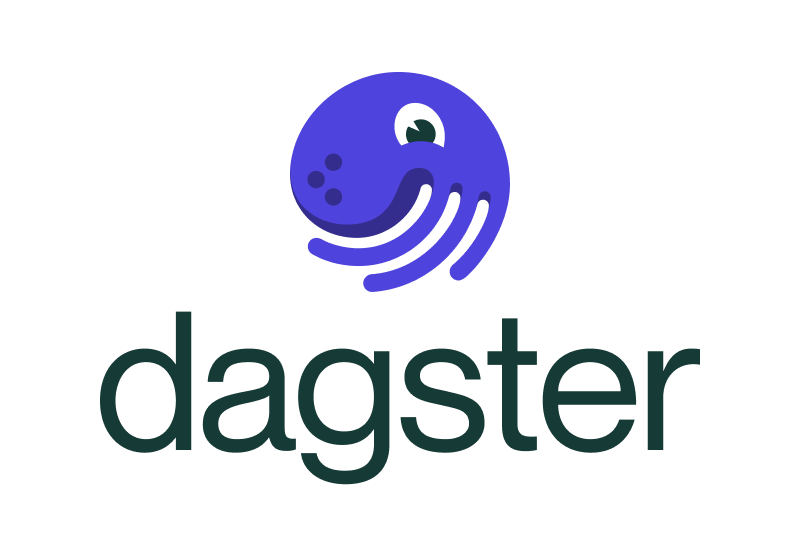 Data teams are tasked with helping organizations deliver on the premise of data, and with ML and AI maturing rapidly, expectations have never been this high. However data engineers are challenged by both technical complexity and organizational complexity, with heterogeneous technologies to adopt, multiple data disciplines converging, legacy systems to support, and costs to manage. Dagster is an open-source orchestration solution that helps data teams reign in this complexity and build data platforms that provide unparalleled observability, and testability, all while fostering collaboration across the enterprise. With enterprise-grade hosting on Dagster Cloud, you gain even more capabilities, adding cost management, security, and CI support to further boost your teams' productivity. Go to [dagster.io](https://dagster.io/lp/dagster-cloud-trial?source=data-eng-podcast) today to get your first 30 days free!
- Data Council: 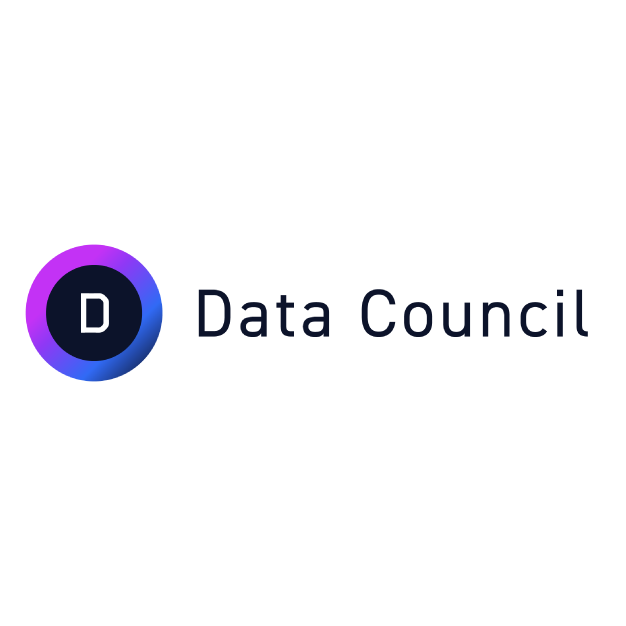 Join us at the top event for the global data community, Data Council Austin. From March 26-28th 2024, we'll play host to hundreds of attendees, 100 top speakers and dozens of startups that are advancing data science, engineering and AI. Data Council attendees are amazing founders, data scientists, lead engineers, CTOs, heads of data, investors and community organizers who are all working together to build the future of data and sharing their insights and learnings through deeply technical talks. As a listener to the Data Engineering Podcast you can get a special discount off regular priced and late bird tickets by using the promo code dataengpod20. Don't miss out on our only event this year! Visit [dataengineeringpodcast.com/data-council](https://www.dataengineeringpodcast.com/data-council) and use code **dataengpod20** to register today! Promo Code: dataengpod20