
Data Engineering Podcast
Unpacking The Seven Principles Of Modern Data Pipelines
Episode guests
Podcast summary created with Snipd AI
Quick takeaways
- Balancing simplicity and complexity is key in implementing modern data pipelines for efficient data management.
- Using cloud technologies and transition to cloud environments are essential for effective implementation of modern data pipeline principles.
- Managed services with usage-based pricing models offer cost efficiency and scalability for optimizing data operations.
- Finding the right balance between simplicity and capability is crucial for user-friendly yet powerful data management tools.
- Generative AI technologies play a significant role in advancing data operations, streamlining processes, and enhancing user experiences in data management systems.
- Supporting diverse storage layers and enabling self-service tools for various user roles accelerates data utilization and promotes collaborative decision-making in organizations.
Deep dives
Challenges in Legacy Tools
The biggest challenge in implementing the seven principles of modern data pipelines is finding a balance between simplicity and complexity. Legacy tools often require extensive learning curves due to the accumulation of features over time to solve specific complex scenarios. Conversely, oversimplified tools may lack the capability to address unique and intricate use cases, leading organizations to develop additional solutions. Striking the right balance between offering an intuitive user experience for simple tasks while enabling solutions for complex scenarios remains a significant challenge across many data management tools.
Cloud-based Infrastructure
The application of cloud technologies is foundational to implementing the seven principles of modern data pipelines. Utilizing zero infrastructure management and an ELT approach versus ETL models are intrinsic to cloud environments. However, if organizations are restricted to on-premises deployments due to regulations or internal decisions, the full benefits of these principles may not be achievable. Transitioning to the cloud opens up opportunities to leverage modern data pipeline principles effectively.
Usage-based Pricing Models
The shift towards usage-based pricing models offered by managed services presents a significant opportunity to fine-tune costs and optimize data operations. This pricing approach enables organizations to match expenses with value metrics, ensuring cost efficiency and scalability. While navigating the complexities of pricing metrics such as data volumes and operations can be challenging, leveraging usage-based models facilitates aligning costs with the outcomes achieved, promoting rapid experimentation and value delivery.
Balancing Simplicity and Complexity
A critical lesson learned in applying the seven principles of modern data pipelines is the challenge of balancing simplicity without sacrificing capabilities to handle complex use cases. Tools often either oversimplify or overcomplicate data management processes, creating barriers for users. The quest for the right balance between enabling an easy user experience for routine tasks and offering sophisticated functionalities to address intricate scenarios remains a focal point for tool development.
Future Advancements with Generative AI
The integration of generative AI technologies holds promise for advancing data operations and insights. Tools designed to expedite SQL writing, process optimization, and streamline data pipeline health monitoring are emerging. Leveraging generative AI to enhance user experiences, reduce development times, and boost data value delivery represents a critical area for further innovation in the data management landscape.
Hybrid Data Architectures
An intriguing evolution observed in modern data pipelines involves supporting diverse storage layers and locations within organizations. As businesses adopt hybrid data architectures, combining cloud solutions like Snowflake with proprietary systems, accommodating various data storage needs becomes essential. The ability to seamlessly navigate between different data storage environments while ensuring data integrity and efficiency opens up new possibilities for data-driven decision-making.
Self-Service Data Pipelines
Enabling various user roles, such as data analysts and BI developers, to build data pipelines through self-service tools transforms data operations. Empowering users to create pipelines without extensive ETL expertise accelerates time-to-insight and data utilization. A shift towards self-serve data pipeline experiences simplifies access for a wider range of users, reducing bottlenecks and promoting collaborative data-driven decision-making.
Advanced Use Cases in Financial Analysis
Innovative applications of modern data pipelines are evident in scenarios such as real-time financial analysis simulations. For example, enabling business users to trigger data pipelines from within their core applications, processing data and presenting financial insights seamlessly. Such advanced use cases demonstrate the potential of bringing data closer to the end users, empowering informed decision-making and driving operational efficiencies.
Practicality for On-Premises Deployments
While cloud-based infrastructure aligns well with modern data pipeline principles, on-premises deployments may raise challenges. Implementing these principles in non-cloud environments, due to regulatory or strategic reasons, can limit the full realization of benefits. Transitioning to the cloud environment is recommended to leverage the capabilities and efficiencies offered by the principles of modern data pipelines.
Prospects for Data-driven Decision-making
The future advancement possibilities revolve around facilitating generative AI technologies to enhance data operations. Streamlining processes such as SQL writing and data health monitoring, along with boosting user experience and accelerating data delivery, presents a realm for growth and innovation in data management systems.
Simplification and Complexity in Tool Development
A critical aspect of modern data pipeline implementation is the need to balance simplicity with complexity in tool development. Many tools either oversimplify data management processes or introduce unnecessary complexities, hindering user adoption. Striving to deliver an intuitive user experience for routine tasks while accommodating advanced functionalities for complex scenarios remains a key challenge for tool developers.
Summary
Data pipelines are the core of every data product, ML model, and business intelligence dashboard. If you're not careful you will end up spending all of your time on maintenance and fire-fighting. The folks at Rivery distilled the seven principles of modern data pipelines that will help you stay out of trouble and be productive with your data. In this episode Ariel Pohoryles explains what they are and how they work together to increase your chances of success.
Announcements
- Hello and welcome to the Data Engineering Podcast, the show about modern data management
- Introducing RudderStack Profiles. RudderStack Profiles takes the SaaS guesswork and SQL grunt work out of building complete customer profiles so you can quickly ship actionable, enriched data to every downstream team. You specify the customer traits, then Profiles runs the joins and computations for you to create complete customer profiles. Get all of the details and try the new product today at dataengineeringpodcast.com/rudderstack
- This episode is brought to you by Datafold – a testing automation platform for data engineers that finds data quality issues before the code and data are deployed to production. Datafold leverages data-diffing to compare production and development environments and column-level lineage to show you the exact impact of every code change on data, metrics, and BI tools, keeping your team productive and stakeholders happy. Datafold integrates with dbt, the modern data stack, and seamlessly plugs in your data CI for team-wide and automated testing. If you are migrating to a modern data stack, Datafold can also help you automate data and code validation to speed up the migration. Learn more about Datafold by visiting dataengineeringpodcast.com/datafold
- Your host is Tobias Macey and today I'm interviewing Ariel Pohoryles about the seven principles of modern data pipelines
Interview
- Introduction
- How did you get involved in the area of data management?
- Can you start by defining what you mean by a "modern" data pipeline?
- At Rivery you published a white paper identifying seven principles of modern data pipelines:
- Zero infrastructure management
- ELT-first mindset
- Speaks SQL and Python
- Dynamic multi-storage layers
- Reverse ETL & operational analytics
- Full transparency
- Faster time to value
- What are the applications of data that you focused on while identifying these principles?
- How do the application of these principles influence the ability of organizations and their data teams to encourage and keep pace with the use of data in the business?
- What are the technical components of a pipeline infrastructure that are necessary to support a "modern" workflow?
- How do the technologies involved impact the organizational involvement with how data is applied throughout the business?
- When using managed services, what are the ways that the pricing model acts to encourage/discourage experimentation/exploration with data?
- What are the most interesting, innovative, or unexpected ways that you have seen these seven principles implemented/applied?
- What are the most interesting, unexpected, or challenging lessons that you have learned while working with customers to adapt to these principles?
- What are the cases where some/all of these principles are undesirable/impractical to implement?
- What are the opportunities for further advancement/sophistication in the ways that teams work with and gain value from data?
Contact Info
Parting Question
- From your perspective, what is the biggest gap in the tooling or technology for data management today?
Closing Announcements
- Thank you for listening! Don't forget to check out our other shows. Podcast.__init__ covers the Python language, its community, and the innovative ways it is being used. The Machine Learning Podcast helps you go from idea to production with machine learning.
- Visit the site to subscribe to the show, sign up for the mailing list, and read the show notes.
- If you've learned something or tried out a project from the show then tell us about it! Email hosts@dataengineeringpodcast.com) with your story.
- To help other people find the show please leave a review on Apple Podcasts and tell your friends and co-workers
Links
- Rivery
- 7 Principles Of The Modern Data Pipeline
- ELT
- Reverse ETL
- Martech Landscape
- Data Lakehouse
- Databricks
- Snowflake
The intro and outro music is from The Hug by The Freak Fandango Orchestra / CC BY-SA
Sponsored By:
- Datafold: 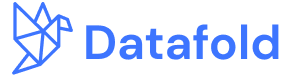 This episode is brought to you by Datafold – a testing automation platform for data engineers that finds data quality issues before the code and data are deployed to production. Datafold leverages data-diffing to compare production and development environments and column-level lineage to show you the exact impact of every code change on data, metrics, and BI tools, keeping your team productive and stakeholders happy. Datafold integrates with dbt, the modern data stack, and seamlessly plugs in your data CI for team-wide and automated testing. If you are migrating to a modern data stack, Datafold can also help you automate data and code validation to speed up the migration. Learn more about Datafold by visiting [dataengineeringpodcast.com/datafold](https://www.dataengineeringpodcast.com/datafold) today!
- Rudderstack:  Introducing RudderStack Profiles. RudderStack Profiles takes the SaaS guesswork and SQL grunt work out of building complete customer profiles so you can quickly ship actionable, enriched data to every downstream team. You specify the customer traits, then Profiles runs the joins and computations for you to create complete customer profiles. Get all of the details and try the new product today at [dataengineeringpodcast.com/rudderstack](https://www.dataengineeringpodcast.com/rudderstack)